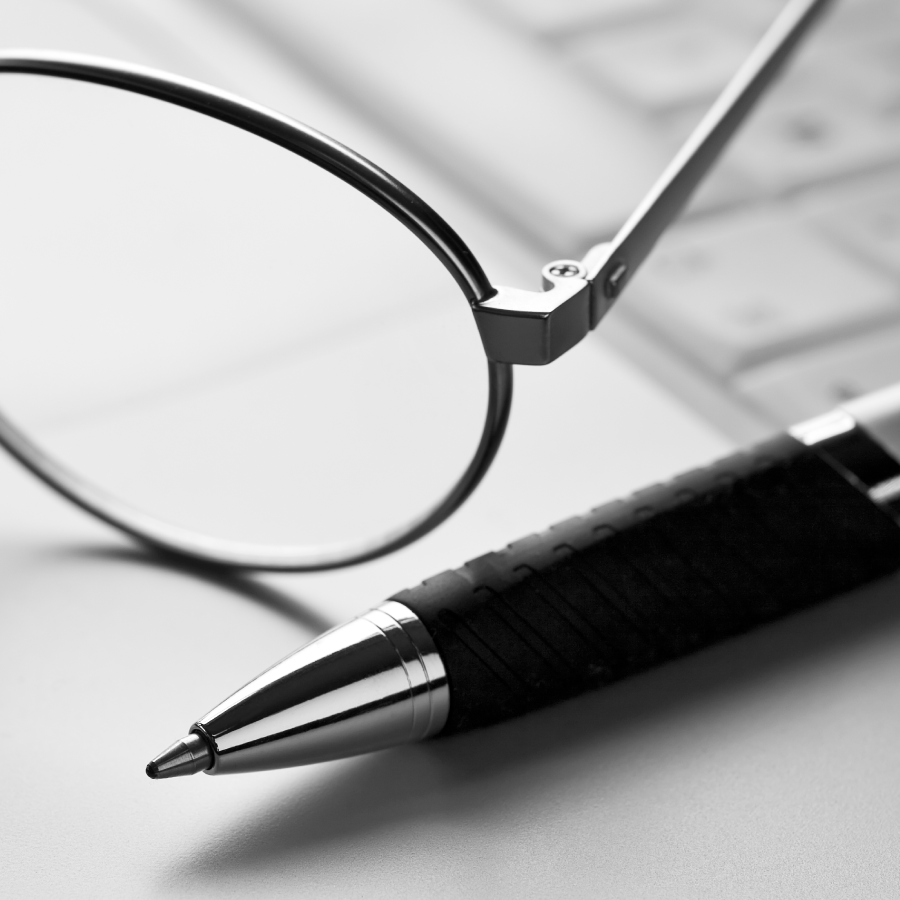
Over data en analyse
In de afgelopen decennia heb ik allerlei artikelen over data, analyse en Customer Relationship Management (CRM) geschreven. Op deze pagina zijn veel van mijn wetenschappelijke publicaties, artikelen in de media en interviews terug te lezen. Mijn blogs zijn te lezen op de site van de Marketing Coöperatie.
Next best offer
Drie artikelen van begin deze eeuw over de waarde van analyse en voorspelmodellen in het benutten van inkomende klantcontacten. In ‘Online Scoring’ wordt uitgelegd wat de mogelijkheden van voorspelmodellen zijn en hoe ze het beste kunnen worden ingepast in de toen gangbare outbound CRM benadering. ‘RealTime Interaction Management’ beschrijft mijn toenmalige visie op wat voorspellingsmodellen zouden kunnen betekenen in het benutten van klantinteracties en voeren van klantdialogen, over alle kanalen en media heen. En Gartner’s Spaarbeleg case is de vroege erkenning (2000) van onze unieke oplossing en van wat later een interessant verdienmodel bleek te zijn.
De door ons ontwikkelde oplossing is later bekend geworden als ‘RealTime Recommendations’ en ‘Next-Best-Offer’. Het werd het fundament onder de latere ‘RealTime Marketing’, ‘1-op-1 Marketing’, ‘Inbound Marketing’ en ‘Personalisatie’. Tegenwoordig heeft elke website en mobiele app wel een (zelf)lerend algoritme om individuele bezoekers te kunnen benaderen met de best passende propositie en het meest kansrijke aanbod. Specifiek de maatwerk software die we in het Spaarbeleg project gezamenlijk hebben ontwikkeld, is na de implementatie door Data Distilleries teruggekocht, en doorverkocht aan SPSS (Clementine) en later IBM (Modeler).
Onderstaande artikelen weerspiegelden mijn geloof in een toekomst met analytics en modellen/ algoritmen. De Spaarbeleg ervaring en deze artikelen waren voor mij het startpunt van een continue zoektocht naar het creëren van (klant)waarde met marketing analytics en voorspelmodellen.
Gartner's verhaal over de Spaarbeleg case
Spaarbeleg’s Contact Center Scores in Real Time (Gareth Herschel, maart 2000)
Printversie: Gartner Spaarbeleg
By providing real-time customer information to contact center agents, a Dutch bank is able to generate strong leads and add value to customer interactions.
Many marketing organizations are familiar with the application of customer scoring to the improvement of outbound telephone sales and direct-marketing campaigns. The use of this technique to achieve returns on inbound calls is gaining attention. One company applying customer scoring to good effect is Spaarbeleg, a Dutch financial services enterprise.
Problem: Spaarbeleg was faced with the challenge of increasing sales to its customer base. Aware of the danger of oversaturating its customers with unsolicited messages, and with most inbound callers using automated channels, Spaarbeleg was looking for a way to improve the conversion rate of inbound service calls to generate new business.
Objective: To improve the conversion rate of inbound calls, Spaarbeleg decided that its customer service representatives (CSRs) needed a clearer indication of which customers were good prospects for cross-selling. The return on investment (ROI) would come from two primary areas:
• Increased sales volumes generated from more-effective targeting of customers for cross-selling during the interaction; Spaarbeleg estimates that a 0.1 percent increase in successfully cross-sold inbound calls will result in a $4.4 million (NLG10 million) increase in sales
• More-profitable sales, as a result of identifying the optimal product for cross-selling and eliminating the cost of outbound communications or resellers.
Approach: Since the project’s goal was to provide CSRs with better customer information, Spaarbeleg needed to perfect the technical aspects of making customer insight available to CSRs, as well as address the issues related to handling the CSRs’ know-how. Early in the project, the company decided to use realtime systems to generate customer scores. This decision was made because the alternative would be to batch score the entire database, which would not be a scalable option as the number of scoring models grew. With this decision made, the enterprise began to evaluate data-mining vendors, with a focus on finding one that had a vision that matched Spaarbeleg’s own. The eventual choice was Data Distilleries (see Note 1). Ensuring that a technical capability is present does not guarantee that it will be used effectively. Thus, ensuring that a system works well in the contact center environment is crucial to such a project’s success. The skill set of the Spaarbeleg contact center determined that the project could be run with the active participation of the CSRs. Three organizational decisions were made: 1) Use of the system by CSRs is voluntary, but an incentive plan provides benefits for those successfully using it. 2) The contact center manager can define how strong a prospect must be before the CSR is prompted to cross-sell. During busy call periods, only the best prospects are displayed, which limits the impact cross-selling has on satisfying routine customer inquiries. 3) The final element of the organizational effectiveness of the project was the creation of an intuitive interface for the CSR. In this case, a simple bar chart represents the customer’s propensity score, and the data-mining models used can be easily explained to the CSR.
Results: The project is in a pilot phase (see Note 2), with only one product being scored; however, early results are promising. The system is performing well, with a three-second response time from the entry of the client’s account number to the display (or not) of a cross-selling prompt. The flexible adoption of the system into the operational life of the contact center means that fewer than 20 percent of inbound calls generate a cross-sell suggestion, and less than one-third of the suggestions are acted upon by agents (who exercise their own judgment about the prospects for cross-selling during that call). As a result of this two-stage filtering system, more than 50 percent of prompts generate a qualified lead to the sales force, and its conversion rate is also around 50 percent. Overall, the project is currently returning approximately 10 percent of the business case with only one product (of the anticipated 15 to 20) being modeled and offered to clients.
Bottom Line: Effective cross-selling requires the organization to be able to generate and deliver accurate customer information to the point of contact. The integration of customer analysis systems with channels such as the contact center enables significantly more-effective cross-selling when the customer chooses to contact the bank. Spaarbeleg has demonstrated both the feasibility of such a strategy and the significant business benefits that can be gained.
RealTime Interaction Management
Gert Haanstra en Pieter W. Boelens, Defying the Limits, A Thought Leadership Project from Montgomery Research, Montgomery Research Inc., San Francisco, Volume 2, 2001, blz 145 t/m 148
Printversie: RealTime Interaction Management
Analytical Customer Relationship Management (aCRM) has become a vital link in creating, developing and maintaining long life customer relationships. Recent developments reveal that the potential of aCRM goes beyond its original analysis function. Looking for unexpected patterns in the database, explaining behaviour and producing predictive models has made way for direct and interactive use of the predictive customer information obtained. The new generation of aCRM is focused on making the dynamic interaction process with the individual customer predictable, with opportunities and threats being flagged up in real time and exploited immediately. The real-time exploitation of opportunities is achieved by generating the best propositions automatically, and distributing them via central control of the distribution channels. The development reveals that aCRM is even more the engine initiating interactions with customers, providing and promoting a personal response, everywhere and at all times.
A second interesting development is the change that marketing itself is experiencing. The marketing function is slowly changing from an “art” with a large measure of “gut feel”, into a controllable, manageable process which needs proven measurable results. This trend started with customer lifetime value marketing (CLTV) where companies opt for a clearly different approach to customers. The (expected) profitability of the customer and the (expected) lifespan of the customer relationship take pride of place with customer lifetime value marketing. CLTV marketing is designed to maximise customer relationships. It calculates the net cash value of the expected profit during the life of the customer or customer group. The company then tailors its investment per individual customer accordingly. In the process, the company seeks to achieve maximum profit at minimum investment.
Targeted approach
Companies are seeking to use analytical CRM as a means of making (outbound) marketing campaigns more effective. The first step is often to test out what is possible with the analytical CRM. What could analytical CRM mean for the company? Does it create money? If so, how much? What investment is entailed? Companies are accustomed by virtue of their profession to store large amounts of customer details. They understand that the data that they have stored on the various databases yield money. But the data only achieves real value when it is given meaning. ACRM is required for this “translation exercise” to enhance the effectiveness of marketing efforts, but also to discover new sales opportunities in the customer base. The database is examined for commercially interesting and surprising (customer) segments. The point is to achieve interesting commercial success in order to induce the company to further investigate the potential for analytical CRM.
The effectiveness of marketing-initiated (outbound) activities is enhanced by looking to see which customer best fits with a particular produce or service. Or to put it another way, finding the right customer and the right offering. The benefit of such score models is that the company can approach its customers in a much more targeted manner. In this way, costs are reduced and at the same time a larger volume of contracts is signed. Score models are drawn up for this purpose to predict who in all likelihood will purchase the specific product. The models are produced by comparing customers who give a smart response with those who do not respond. This enables customer characteristics to be identified that have the greatest predictive value for evoking a response. The same commercial result, or even a better result can be achieved using a score model, with significantly lower runs and/or contact frequency. Higher sales are therefore achieved, at lower cost. It also offers the major benefit that the customer is subjected less frequently to campaigns, which she wasn’t sitting waiting for.
Closed-loop Multi-Channel Marketing
The success of aCRM is enhanced if it becomes a structural part of the marketing and sales process. With the rise of new distribution channels such as the Internet and mobile phones, companies are expanding their distribution channels and integrating aCRM with the existing marketing and sales process. On the one hand to gain maximum benefit from the revenues by consistently using aCRM in the multi-channel marketing process. On the other, to be continuously evaluating and further refining forecasting models that have been developed. Integration ultimately leads to major improvements in the marketing and sales results.
“Closed loop marketing” is the aim. This entails ongoing improvements to the quality of the models, to the creation of more models for new target groups and for other products and earning higher sales volumes. Or, to put it another way, continuity in optimising the commercial process.
The right mix of customers, products and channels is sought with a view to coming into contact with the customer through a wide range of channels for integrating aCRM into the multi-channel concepts. Marketers will then no longer make open-ended use of score models for approaching client groups. Instead, for each campaign there will seek out the highest-scoring target group in the database. Their aim is to optimise campaigns as far as possible by using score models.
By making customer contacts structurally more effective, aCRM clearly adds greater value compared to operational applications (often already in place) such as campaign management, sales force automation, call centre and web applications). As the customers with the highest likelihood of conversion or response are identified, more and profitable products can be sold to the most profitable and loyal customers. The maximum result is obtained where there is seamless technical integration between the aCRM application and the existing infrastructure.
The predictive quality of existing models is enhanced by using them and testing them against old models. Ultimately, these cycles lead to a set of good-quality prediction models for most “standard” computerised campaigns. The ultimate result is a smooth-running campaign management process, ongoing monitoring of the quality of selections and effective distribution of customers among marketers’ campaigns. In short, an instrument for processing existing customers in an effective and efficient manner.
Example Closed-Loop Marketing
An example of a successful application of aCRM concerns a large financial institution which uses direct marketing as its main means of serving its eight million-plus customers.
Objective: to optimise the direct mail processes by means of an integrated aCRM solution.
Application: Data Distilleries software is used in combination with campaign management software. As a result of the integrated aCRM solution, database marketers are actually in charge of defining customer selections for direct mail campaigns. Data Distilleries software rapidly analyses the whole customer database and presents the results in the form of clear customer profiles. The solution offers database marketers the possibility of analysing customer data and incorporating the results of these analyses more rapidly in new campaigns.
How is aCRM used?
The bank uses DD/ Marketer, a special module for marketers, in order to analyse the whole customer database more quickly and to export the results with the associated scoring probabilities quickly and easily to the campaign database. The integration has made the building of mail models a simple process and one which can be carried out independently by the database marketers. In order to select the most promising customers from the database, the database marketers apply various types of analysis. For example, they can first carry out a trial mailing, and analyse the response to this mailing. The Data Distilleries software then generates a model which specifies which customers will show most interest in the bank’s financial products. Alternatively they might use the so-called propensity analysis. Here the software analyses product purchases by current customers, in order to produce a model for target group selection.
In the example of this Dutch bank:
Direct mail process before aCRM was used:
– the building of models took over 4 weeks,
– this was very labour-intensive and required effort on the part of several departments,
– only 30% of the mailings sent out were based on models,
– no customer knowledge was built up by the database marketing department.
Direct mail process after implementation of aCRM:
– the building of models takes only a few days,
– the customer data is always ready,
– the data can be used immediately in the campaign management software, in this case, Vantage from PrimeResponse,
– 70% of the mailings sent out are based on customer models, enabling more targeted mailing,
– the database marketing department learns more about its customers with each direct mail action.
The bank uses Data Distilleries software to distil usable customer knowledge from the enormous volume of available customer data. This results in a shortening of the database marketing process so more campaigns can be implemented on the basis of models by the same number of people. The benefits of this operation are obvious: a higher response, less irritation among customers and a reduction in waste. The large numbers of mailings which the bank sends out in the form of leaflets with statements, further reinforce this effect. If the waste can be reduced by just a few per cent, this will quickly lead to annual savings of many millions.
Real-Time Recommendations
The customer is increasingly taking the helm, specifically in the interactive distribution channels such as call centres, voice response units and the Internet. The customer or prospect is himself taking the initiative to contact the company. That is the ultimate moment for marketing. Real-Time Recommendations is a suitable approach to adopt here.
The concept remains the same whether one is dealing with a call centre or the Internet. At the time when the customer seeks contact with the company, existing knowledge of that customer must immediately lead to a personal, commercial proposition tailored by the company. In itself, not a problem. Selecting the best approach on the basis of a battery of scores would appear to be the solution. But what if the customer comes to make a complaint or order a product, making the proposed approach pointless? The customer is taking the initiative to make contact and will therefore first be asking something of the company. This information is essential to determining any commercial approach towards the customer. Pre-calculated scores on the basis of score models are insufficient. The real-time calculation of score models, with existing customer information along with information that has just been obtained (in real-time) is therefore a must; hence the term Real-Time Recommendations.
Real-Time Recommendations are highly lucrative. (See example) It is a simple matter to flag the best cross-selling and up-selling opportunities for inbound customer contacts and to use them directly, driven by underlying score models. Sales are directly and visibly increased, because the customer is given a product offering that best suits him, and he receives the product offering at the time when he is actually receptive to it. In addition, using Real-Time Recommendations focuses inbound marketing activities on the most profitable and most loyal customers in the database. Marketers can opt to sell the products with the highest margin. They are given the opportunity to give a higher priority to recommendations which will increase the profitability and loyalty of these most valuable customers.
Example: Real-Time Recommendations
A good example of a successful ROI is the case of a financial organisation which does not operate through intermediaries – a direct writer.
Objective: to exploit a percentage of the incoming customer contacts for cross-selling to promote the most profitable product.
Application: Data Distilleries software in the call centre. As soon as an attractive opportunity arises, the software gives a suggestion for a product to be offered by means of real-time recommendations . In the corner of the screen a separate small screen appears. This states the name of the product and the probability of success. With a double-click the employee gains an insight into the structure of the underlying model and a number of product-specific sales arguments, and he sees a possible opening sentence.
What was measured?
Prior to the actual measurement, the ‘autonomous’ response was measured over a certain period. This data made it possible to determine the extra returns of real-time recommendations . After all, the real return is equivalent to the real-time recommendations results minus the autonomous response. In addition, two groups of call centre agents were defined: one group used real-time recommendations , and the other engaged in cross-selling without the software. This structure made it possible to take account of the difference in the selling quality of the agents. Three sets of measurements were then taken:
1) The number of times an opportunity arose; how often the suggestion was presented; how many telephone calls were received for the agents connected to the system and how often the suggestion actually appeared above the threshold value.
2) The number of times the call centre agent took up the suggestion; how often he was presented with a suggestion and did nothing with it, and how often the agent tried to do so, but without success.
3) How often success was actually achieved. This figure was broken down into commercial successes (leads created, brochures sent out, information provided, a product sold other than the proposed product) and genuine success (actually selling the proposed product).
In our example of the direct writer:
– a suggestion was presented in 15% of the telephone calls,
– 30% of these were taken up by the agent in order to make an offer to the customer,
– 50% of these were commercially successful,
– 50% of these were successful sales of the proposed product.
The precise figures were:
– 50,000 telephone calls per month (via the connected agents),
– 7,500 telephone calls were potentially attractive for sales,
– agents made offers to customers on 2,250 occasions,
– 1,125 successes were achieved, and
– 563 contracts were concluded.
With a profit margin per contract of 500 Euro (spread over 20 years), this generated an additional profit contribution of more than 3.3 million Euros per year. And that was just for one product and a small group of approximately six call centre agents.
The software has a very favourable ROI which is relatively easy to achieve. Good results can be achieved in a very short period even with a limited number of products. In order to gain an even better picture of the ROI, the measurement can be regularly repeated for different products and across several channels.
Real-Time Analytical Interaction Management
The logical step after implementing Real-Time Recommendations is to use aCRM for real-time control of all marketing activities. Companies wish to get a better grip on the dynamic process of interaction with the individual customer (including the interactions initiated by the marketer himself). They want to be able to control and adjust the process rapidly and be better able to predict the results of the interactions so as to bring the objectives at customer level in line with corporate objectives. The aim is to actually maximise total yield and profit over the long term for each individual customer (Customer Life Time Value).
Real-Time Analytical Interaction Management allows a marketer to pursue a wide range of objectives, and define them in various personal, real-time interaction strategies with the customer. Targeted propositions aimed at the individual customer are developed for this purpose. These propositions cover a specific action in which a product or service is offered, expressed in a particular message. Various propositions are related to different corporate objectives (such as cross-selling, up-selling, cost reduction, retention, penetration and profitability). The interaction strategies are complementary.
Interaction strategies are defined, or may be defined covering a wide range of distribution channels (= multi-channel), contact moments in time (= multi-step), for various customer segments, in a combination of inbound and outbound contacts. Predictive models are used to do so. The models are easy to use and provide a stable prediction of customer behaviour, evolving over time, among the various distribution channels and in a rapidly changing environment.
Interaction strategies are implemented automatically. The situation is constantly being analysed to see whether there are opportunities and/or threats. On the basis of the complete (including the most recent) information, changes in the customer situation are flagged that provide good grounds for communicating a specific proposition. This information may emanate from the customer himself (if for example he calls, visits the website or responds to an offer). It is information stored in the databases that generate interesting events (such as a birthday, wedding day, Valentine’s day, etc.). It may also be information which is initiated by the company (defined interactions for direct mail, or a phone call to the customer).
In addition, companies are prepared to exploit opportunities/threats that may arise at any time and for various propositions and company objectives. This requires pro-active preparation for interaction with the customer. The best proposition for this specific customer is generated at the time of interaction. This means a dynamic weighing-up between various customer objectives such as cross-selling, up-selling, cost savings, loyalty and customer value. This is based on a consistent customer picture which changes (in real-time) to the new situations. Only then is it possible to exploit opportunities in time.
Furthermore, the propositions are constantly being disseminated by central control of the various distribution channels by automatically providing the best propositions, at the right time. Customer knowledge is collected and disseminated in turn among the various distribution channels from this central position. This calls for customer knowledge to be integrated from/in this central position. A clear understanding of all activities targeted at the customer enables every customer to be approached to best effect and consistently via each distribution channel required and at each desired moment. Only then is it possible to flag opportunities and threats in a timely manner from analysis and to make pro-active preparations at every interaction with the customer.
If supplier wishes to make the central position of CRM a reality, he must be able to collaborate. Apart from the functionality listed above, the application will have to integrate with any application, database or infrastructure. The source of the supplier determines the technical possibilities that can be achieved in this central position. The fact is that the technology could soon become outdated because the wrong architecture was adopted in the past. Technical developments are proceeding so quickly that incorrect choices at the heart of things are virtually beyond rectification. The technology for the CRM engine must in any event be easy to expand across all distribution channels, and easy to integrate with the existing infrastructure in line with generally accepted standards.
To achieve the objectives set, the results of the strategies are evaluated continuously in real-time, and the marketer is given the opportunity to adapt the strategies “on-the-fly”. How does one work towards achieving customer objectives?
Real-Time Monitoring and Real-Time Alerts are deployed to remain abreast of the result. Performance being achieved with the strategies is monitored by a number of pre-defined key variables. These mainly focus on the results during the period in which the strategy is active. The information is presented to the marketer in real-time, i.e. in (milli-)seconds by means of a monitor/dashboard. Various types of monitors may also be developed (for example for marketing and sales directors, marketing analysts, call centre managers, etc.) to monitor their specific area. To be able to flag (significant) deviations, the results are compared directly with the forecasts, expectations and/or objectives previously made.
Concrete modifications to ensure that the objectives are attained are proposed on the basis of historical data/results. An organisation can learn much from customer contacts. One condition is that the communication with the customer is logged as far as possible via a wide range of distribution channels, contact moments, for various customer segments and propositions. Analysis of the contact data shows which desired changes can be implemented by the marketer. A number of levels are available here: strategy, contact and model level. In the first instance, the strategy is modified. For example, the respective priorities between the strategies are changed, the influence of general business rules weakened/strengthened, or strategies halted or started. At contact level, distribution channels are removed or added, response options changed, more specific contacts deferred or advanced. At model level the definitions of the predictive models are altered. Generally by producing a new predictive model which forecasts who responds, with which offering, via which distribution channel and when. After implementing the changes, a new balance is achieved in communication with the customer, which takes account of previous propositions.
Conclusion
Analytical CRM is gaining a place for itself as the brains in the centre of any CRM business strategy. It is an essential link in establishing, developing and maintaining customer relationships by the real-time flagging of opportunities and threats, and directly exploiting these moments. Mainly by generating the best propositions automatically and distributing them by a central control of the distribution channels. Interactions with the client are initiated dynamically, dealt with and promoted, everywhere and at all times.
The central position is needed to achieve real competitive advantage with Customer Life Time Marketing. ACRM is needed to identify who the (potentially) profitable and loyal customers are. Furthermore, activities can be initiated with aCRM which are designed to maximise the customer’s lifetime value. This is achieved by anticipating expected customer behaviour by analysing historical customer behaviour and predicting future behaviour. But what the new generation of aCRM most achieves is that it puts the marketer (back) in control.
Online Scoring; missing link in Customer Relationship Management
Gert Haanstra en Melbert van Emmerik, Journal of Database Marketing, Henry Stewart Publications, Londen, Vol 7, number 3, 1999, pages 275 – 279
Printversie: Online scoring; a missing link in customer relationship management
In this paper we describe a new kind of decision support technique for cross selling clients in interactive media. This so called ‘online scoring’ predicts client behavior real-time as opposed to pre-scoring, and suggests the call centre agent to sell a specific product. In addition, we evaluate an implementation in a customer relationship management solution and provide a framework for implementing online score models.
Introduction
The combination of data mining and campaign management has rapidly emerged as a powerful technique in customer relationship management. However, one-to-one marketing is not possible/feasible yet because of the lack of interactivity based on information in the database. In a already advanced setting clients who contact a call centre or the internet get an offer based on profile information. Different customer profiles fit into different campaigns. When a client is identified the contact management system will match his profile to the different campaigns. The customer gets an offer associated with the campaign the best suits his profile. Up to this moment the offer is based on past interactions and knowledge available in the marketing database. But people search contact for a reason. He wants to withdraw money, files a complain or requests information. This very relevant information is not available at the time of the profile scoring process. So the in advance suggested campaign might not be the best move anymore. With online scoring not only the pre-available information will be the basis for profiling, but also the newly acquired information during the contact at present. Therefore online scoring is indispensable for optimising the effectivity of different client contacts and increasing the efficiency of using interactive distribution channels.
In essence, using online scoring means that the communication with clients will move from a monologue to a dialogue. If necessary information about a client is available, an effective dialogue can be established. Such a dialogue means that the client will get insight in one’s production process, and will get the feeling he can influence it directly. Result will be an increase of customer satisfaction, en strongly related to that, a long time relationship with your clients. Therefore using online scoring gives one the opportunity to acquire customer effectively, and building successful relationships with most profitable customers, and to maintain their loyalty and profitability.
On-line scoring and CRM
Generally, customer relationship management is about building close relationships with current and potential customers. Companies try to know their (potential) customers, and want to understand what their customers want. As a result companies can offer products or services that best match the needs of their clients. Data mining is used for understanding customer behaviour by analysing data, identifying ways to serve customers better by testing and evaluating a range of possible (personalised) campaigns. As best they can, companies try to satisfy their needs through personalised service. As a result, they expect to earn loyalty and a large share of their customers’ share of wallet. Campaign management is used to develop, execute, monitor and evaluate customer campaigns in order to encourage customers to develop a better relationship with the company.
Online scoring has the potential to immediately identify and use new cross sell opportunities based on predefined business goals and model definitions. It has therefore additional advantages compared to existing customer relation management solutions. Advantages of this method include the ability to (a) implement predictive modelling in interactive media (e.g., call centre, internet, voice-response media), (b) increase convergence rate by presenting most prioritised product offer, (c) focus on business objectives evoked by relevant score models, and (d) directly relate decision outcomes to business objectives.
An example
If the client uses the internet, he will receive a suggestion on what to buy. If he contacts a call centre, the agent will get a signal that suggests him what to sell. The suggestion for the agent consists of the product name (of the most likely product the client will buy), an indication of the expected success ranging from 0% (low) to 100% (high). In addition, the agent will be shown the reasons why the client would be interested in the suggested product (insight in the model). An agent decides what to do with the suggestion and also can use a predefined script and a description of the key features of the product.
More specific, a client contacts his bank. The moment the agent identifies the client, a first suggestion is calculated and presented immediately based on the current situation of the client. Then the new information acquired during the conversation is incorporated. If somebody withdraws his money from his saving account, obviously the score model should provide a different score compared to the case where the same person deposits money into his account. Therefore, during the conversation with the client, the models should be recalculated and the most appropriate action should be presented online within several seconds. So, by using on-line score models even the latest changes in the clients (financial) situation, his behaviour and preferences are taken into account when offering a new product to the client.
Of course not everybody is evenly suited for action. The best campaign for a specific customer might not have a very high success expectation. “Bad leads” for call-center agents should be avoided. In the end they might sabotage your system. Even worse, you don’t want to bother your customers with all sorts of offers all the time when you don’t expect them to respond. So in addition to scoring, you will want to set thresholds to scores below which no suggestions will be made. An experienced agent will get suggestions for offers with an expected change of success above 20%, whereas less experience agents only get suggestions for the most potential offers.
Development of online scoring
Online scoring is a result of the way data mining has developed itself in the marketing & sales process over the years. The ‘Exploring phase’ is used to find data mining opportunities, to learn about the processes involved, and to overcome the initial scepticism in using data mining by showing very impressive results. Companies become aware of the profits it can generate. They do some ad hoc research in order to find out about costs, profits, and expected organisational changes. Based on the results they will decide whether data mining can increase the effectivity of marketing and, in addition, if data mining is useful for finding new opportunities/segments in the database.
The next step is to bring continuity in using data mining; continuity in developing, using and evaluating score models. As a result, structural improvements of campaigns are established which provide companies a maximum of gains. This will often lead to building data warehouses (DWH) to offer quick and consistent input for data mining. In this stage all effort will be put into optimising the process of using data mining.
On the other hand, companies can prefer to enlarge the use of data mining for application to other fields instead of bringing continuity in their processes first. In this enlargement phase, on more fields results are gathered, which will prove that data mining has many opportunities.
As the process of data preparations is very time consuming, organisations choose ideally first the stage of optimising before they think about enlarging the possibilities of data mining. But sometimes the stage of enlargement will have to be preferred to get broader attention and hence more resources. All relevant information has to be gathered from operational databases, then data will have to be cleaned and merged. After that, new information will be derived through data manipulation and finally many fields will have to be recoded. Then the analysis file is ready for use. After the data mining process you will have to translate the outcomes into business rules, score models or just plain scores. This will then be used for different kinds of campaigns.
Either way, the next phase is to integrate data mining into the daily marketing & sales process (’Integration phase’). Therefore, (on-line) score models are brought to places where the cross selling actually takes place. E.g. marketers will use them in selecting prospects or selecting campaigns that best fit their customer’s needs. Call centre agents will use them in their interaction with customers. So, decisions about what to offer to whom, via what channel and when, will be primarily based on data mining results.
Implementing online scoring in a CRM environment
Most companies have the operational data stored on a platform separated from their data warehouse. The reason lays in the fact the data warehouses are primarily used to offer raw, derived and summary data for analysis and reporting. By offering people this information on a separate platform, you don’t have to concern yourself about the performance of operational databases. Beside the information did not have to be up-to-moment and therefore updates could easily be run through batch processes. When implementing online scoring this construction leaves us a challenge. A predictor in a certain model might be the ‘number of deposits a customers has made during the last three months’. This predictor has been calculated by counting the number of deposits in that time frame from operational data. A change in operational data during an interaction could not be input for online scoring during that interaction with the regular up-date routines. An option could be that you don’t use aggregated operational data that could change during the interaction session. This would largely reduce the amount of predictors that you could use in the score models. Another solution is to directly tap the newly acquired data into the data mining system so you could use it for recalculating the predictors and the models.
For building a score model, the following functionalities are needed in a data mining tool:
• ‘data access’ for flexible and dynamically updating tables from the data warehouse or/and transactional databases,
• ‘data preparation’ for aggregating data tables and creating user defined tables and variables,
• ‘behavioural scoring’ for creating and using score models for prospect selections
Score models are transferred back into the DWH, and used in the campaign management for prospect selections.
Additionally, a campaign manager should have the following functionalities:
• ‘event triggering’ for detecting client life-cycle events,
• ‘targeting system’ for choosing / evaluating the best way to contact a client,
• ‘scheduling’ for atomising prospect selection,
• ‘channel management’ for distributing and co-ordinating several, multi-channel campaigns,
• ‘decision support’ for prioritising the use of score models and the use of distribution channels,
• ‘reporting’ for determining (financial) consequences of several campaigns and its influence on customer value.
Results of the campaigns are distributed to different channels. In a multi-channel situation clients can be contacted:
• Directly (e.g. mailings, door-drops, coupons),
• Differently (e.g. campaign A for client X and campaign B for client Z)
• Personally (e.g. franchise, brokers, retail),
• Massively (e.g. radio/tv-commercials, newspapers),
• Electronically (e.g. telephone, voice-response, sms),
• Interactively (e.g. e-mail, internet).
The client scores and campaigns for outbound contacts (mail, leads, calls) are calculated in batch processing. In batch, a scheduler is running jobs on historical data from the data warehouse. Automatically, the system will allocate customers to different campaigns.
For inbound contacts we use online data processing and online scoring, which means the data is up-to-moment and the scoring is done real time. In order to get the best prediction, actual information from the conversation taken place should be incorporated in the scoring. Therefore, if somebody withdraws money from his saving account, data should be transferred immediately to environment where the scoring takes place. And the scoring is done, starting the moment a client is identified.
Opportunities for on-line scoring
Expanding the possibilities of online scoring will contribute to building close relationships with your best customers. Key feature to success remains identifying and immediately using new opportunities.
• Acquiring new business: Instead of identifying a current client by his client-number stored in the DWH, a potential customer can be identified by his postal-coding. One can predict what product to suggest, based on geographical information.
• Customer selection: For example, models for credit scoring can be implemented for selecting customers that suit the product characteristics, instead of offering a product to everyone.
• Customer extension: In order to increase the loyalty and the profitability of your customers, the most profitable customers can be identified. For example; these customers could get a VIP-treatment from your call centre agents.
• Customer retention: Optimising payment collection, solving complaints, or tracing possible quitters are part of monitoring subscriber loyalty for keeping the customers for as long as possible.
Conclusion
Knowing what to sell the moment a (potential) client contacts you. In interactive media, the time between knowing what to do and the actual selling has to reduce from several days, or even weeks, to seconds. Online scoring provides such a solution, and is therefore indispensable for optimising the effectivity of different client contacts and increasing the efficiency of using interactive distribution channels. In addition, it has the opportunity in supporting building successful relationships with profitable customers, and to maintain their loyalty and profitability.
‘Database Marketing bij Spaarbeleg zal niet meer hetzelfde zijn’
Interview met Gert Haanstra, InterAegon, Den Haag, jaargang 2000, nummer 2
Printversie: Database Marketing bij Spaarbeleg zal niet meer hetzelfde zijn
Het afgelopen jaar is er bij Spaarbeleg hard gewerkt aan het project Octopus. Een uniek project waarmee een belangrijke en noodzakelijke stap is gezet in de verdere ontwikkeling van (database) marketing bij Spaarbeleg. ‘We hebben moderne marketing technieken als datamining en campagne management, volledig geïntegreerd in ons marketing- en verkooproces. Een behoorlijke klus die maar weinig bedrijven zo goed kunnen doen.’, zegt Gert Haanstra projectleider van Octopus. Inclusief voorbereiding heeft het project anderhalf jaar geduurd. In die periode werden de plannen gemaakt, werden de twee leveranciers geselecteerd en de systemen geïnstalleerd. Vervolgens moesten de mensen ermee leren werken, en konden de eerste resultaten worden behaald. ‘De eerste resultaten zijn boven verwachting en beloven veel voor de toekomst’, aldus Haanstra.
Met de nieuwe technieken wordt optimaal gebruik gemaakt van de mogelijkheden die Spaarbeleg heeft. Bijvoorbeeld, een grote database waarin een hoop gegevens over de klant liggen opgeslagen. En natuurlijk de verschillende distributiekanalen waarover Spaarbeleg beschikt. Spaarbeleg benadert de markt zowel via massa communicatie, zoals met de radio en tv commercials, als ook rechtstreeks. Dit laatste gebeurt veelal met gepersonaliseerde mailings als ook met de bekende huis-aan-huis brochures. Bovendien werkt Spaarbeleg met een eigen franchiseformule (de Spaarbeleggers), en doet zij zaken met het onafhankelijke intermediair. Verder is er een eigen call centre in huis met een aparte verkoopafdeling, en is er een druk bezochte internet-site.
De filosofie achter Octopus sluit prima aan op het streven van Spaarbeleg om enerzijds meer te verkopen aan bestaande klanten en, anderzijds, om de beschikbare distributiekanalen nog beter te benutten. Een belangrijk argument hierbij zijn de verkoopkosten. Een product verkopen aan een nieuwe klant is veelal duurder dan een product verkopen aan een bestaande klant. Bovendien kunnen we veel makkelijker leren van onze bestaande klanten. Door goed te kijken naar wie welke producten koopt, is het beter te achterhalen welk product we de klant moeten aanbieden. Dit geldt ook voor de distributiekanalen. Sommige klanten doen liever zaken via de telefoon, anderen reageren sneller op een advertentie. Door hiermee rekening te houden, worden de distributiekanalen beter benut. En dus worden bepaalde groepen klanten nagebeld, en krijgen andere groepen klanten een aanbod te worden bezocht door een intermediair of franchiser. ‘Uiteindelijk wordt de klant hier alleen maar beter van. We leren veel van onze klanten. En die informatie gebruiken we om betere aanbiedingen te kunnen doen. Deze tools stellen ons in staat om dat op een zo goed mogelijke wijze te doen.’
Maar wat gaat er veranderen op gebied van database marketing?
‘Alles draait om het effectiever maken van onze verkoopinstrumenten, en het beter op elkaar aan laten sluiten van de verschillende distributiekanalen. Een mooi voorbeeld is het call center. Vroeger belde een klant voor een overboeking en moest de call center medewerker zelf achterhalen of deze klant interesse had in een bepaald product. Nu voorspelt de database welke aanbieding we de klant moeten doen. Op moment dat de klant belt, wordt direct berekend welk aanbod geschikt voor hem/haar is. Hierbij wordt ook rekening gehouden met recente aanbiedingen richting de klant. De naam van het product wordt aan de call center medewerker gepresenteerd. De medewerker neemt het mee in het verkoopgesprek. Voorzover mij bekend is dit nooit eerder gedaan. En daarmee hebben we met Octopus opnieuw een mooie voorsprong op de concurrentie.’
Hoe zijn jullie tot de conclusie gekomen dat het anders moest?
‘Het is een verdere ontwikkeling in databasemarketing. Spaarbeleg heeft zeer frequent en op allerlei manieren contact met haar klanten. Als je wilt dat iedereen die namens Spaarbeleg in contact komt met de klant kan profiteren van de informatie in de database, is dit een logische stap. In het verleden hadden we veel tijd nodig om de informatie uit de database te ‘vertalen’ naar concrete campagnes. Door er een automatisch proces van te maken, en er zoveel mogelijk distributiekanalen op aan te sluiten, hebben we er maximaal profijt van. Los daarvan krijgen we de mogelijkheid om de relatie met de klant te verdiepen. Duidelijk is dat databasemarketing bij Spaarbeleg nooit meer hetzelfde zal zijn.‘
Maar wat betekent dit concreet, bijvoorbeeld voor het onafhankelijke intermediair?
‘Op korte termijn zal er weinig veranderen. Op lange termijn is het de bedoeling dat het intermediair toegang krijgt tot deze verkoopsuggesties. Zodat ook zij direct kunnen profiteren van de Spaarbeleg database. De komende periode werken we dit uit. Overigens zijn er nu al intermediairs die vergelijkbare technieken kopen. En dus op eenzelfde manier hun klanten willen gaan bedienen als Spaarbeleg.’
En wat brengt de toekomst?
‘Zo’n investering is natuurlijk bedoeld om meer geld te verdienen. Het systeem biedt daarvoor vele mogelijkheden. Het komend jaar willen we bijvoorbeeld mensen direct inzicht geven in de verwachte kosten en verwachte opbrengsten van hun beslissingen. Zodat we drie duidelijke doelstellingen kunnen realiseren; meer omzet, meer winst en minder kosten.‘
De menselijke kant van analytics
Een aantal artikelen die gaan over de inrichting van een Intelligence afdeling en de ontwikkeling van een analist. Ze benadrukken het belang van de ‘zachte’ kant van analyse en voorspelmodellen. Welgemeende aandacht voor de menselijke kant als achilleshiel voor de transformatie naar een meer datagedreven manier van werken.
Impactvolle Marketing Analist
Gert Haanstra & Jaap Wieringa, MOA Jaarboek 2010, 2010
Printversie: Impactvolle Marketing Analist
Samenvatting:
Uit diverse onderzoeken blijkt dat het vakgebied marketing invloed aan het verliezen is. In dit artikel zetten wij uiteen dat voor het terugwinnen van een deel van die invloed een bijzondere rol weggelegd is voor de kwantitatief ingestelde analisten en de marktonderzoekers. Een voorwaarde daarvoor is wel dat zij erin slagen om de impact die zij hebben in hun werkomgeving te vergroten. We ontwikkelen in dit artikel een methode waarmee een analist of onderzoeker zijn impact kan vergroten aan de hand van vier rollen: de operationele, de adviserende, de bewakende en de innoverende rol. Bij deze rollen horen verschillende vaardigheden en analytische tools die de analist en de onderzoeker daarbij behulpzaam kunnen zijn. De voorgestelde methode is getoetst in de praktijk, met positieve resultaten.
Trefwoorden: de invloed van marketing, accountability, marketing intelligence, de impact van analisten en onderzoekers, proactief handelen.
1. Inleiding
Uit onderzoek van Verhoef en Leeflang (2009) blijkt dat de invloed van marketing binnen het Nederlandse bedrijfsleven sterk is teruggelopen, en dat haar rol in de boardroom dreigt nog verder te worden gemarginaliseerd. Inmiddels heeft marketing al twee van de vier p’s verloren. Zo liggen beslissingen over ‘prijs’ en ‘plaats/distributie’ vooral bij financiën en verkoop, en minder bij marketing. De onderzoekers stellen dat de mate waarin een marketing afdeling werkt met ‘accountability’ en een ‘fact-based aanpak’ bepaalt of ze invloed heeft binnen het bedrijf. Daarnaast blijkt uit het onderzoek dat marketing beter moet presteren op gebied van ‘innovatie’. Uit onderzoek van de Rijksuniversiteit Groningen en VODW
Marketing blijkt dat marketing- en verkoopafdelingen (nog) te weinig gebruik maken van de strategische inzichten van Marketing Intelligence (MI), terwijl een goede samenwerking tussen MI en de andere afdelingen direct bijdraagt aan het bedrijfsresultaat (Verhoef et al., 2009). Uit deze onderzoeken kan worden afgeleid dat de meer kwantitatief ingestelde analisten/onderzoekers/database marketeers de mogelijkheid in zich hebben om (een deel van) de verloren invloed van marketing weer terug te winnen.
De vraag is hoe zij hun invloed binnen en buiten de marketingafdeling kunnen vergroten? Ofwel, hoe zij doelgericht en welbewust een zichtbare en merkbare verandering kunnen realiseren in het handelen, gedrag, opvattingen of werkzaamheden van collega’s, management en klanten. Het doel is dat er meer en vaker naar de analisten wordt geluisterd, dat de mening wordt overgenomen, de conclusies en aanbevelingen worden opgevolgd en het gedrag wordt aangepast. De belangrijkste uitdaging daarbij is om oplossingen te vertalen naar de dagelijkse praktijk, en om blijvende veranderingen te realiseren.
Gebaseerd op de diverse onderzoeksresultaten hebben wij een oplossingsrichting geformuleerd waarmee analisten/onderzoekers/database marketeers hun invloed binnen het Nederlandse bedrijfsleven weer kunnen terugwinnen. Bovendien hebben we ervaring opgedaan met het implementeren van de oplossing. Onze oplossingsrichting en onze ervaringen zijn beschreven in dit artikel.
2. Een veranderende omgeving
De omgeving waarin marketing opereert is de laatste jaren sterk veranderd. ICT ontwikkelingen als internet, email, mobiele telefonie, etc hebben er toe bijgedragen dat de hoeveelheid klantgegevens sterk is toegenomen (Blattberg, Kim & Neslin, 2008). Grote groepen klanten zijn vaker en makkelijker te benaderen. Ze laten zelf op allerlei manieren sporen na, en zijn bovendien snel bereid om persoonlijke informatie te geven. Gelijktijdig hebben consumenten meer keuzemogelijkheden gekregen. Dat geldt voor het aantal aanbieders en de hoeveelheid productvarianten, maar ook voor de diversiteit aan informatiebronnen, communicatiemedia en distributiekanalen. Switchen is ermee eenvoudiger, makkelijker en sneller geworden. Bovendien lijken consumenten pas tevreden met een relevant en een op de persoon toegesneden aanbod. Daardoor is de houding van veel bedrijven ten aanzien van marketing veranderd. Veel managers en directeuren vragen zich af welke bijdrage marketing levert aan het primaire proces en stellen eisen aan de bijdrage van marketing aan het uiteindelijke bedrijfsresultaat (Verhoef & Leeflang, 2007). Ze zijn kritischer geworden op marketing budgetten/uitgaven/investeringen (Ruijs, 2008). En ze vragen zich af waar de nieuwe producten/ideeën blijven (Frambach & Leeflang, 2009).
Het nemen van effectieve marketingbeslissingen is daardoor steeds relevanter geworden. Het ontwikkelen en aanbieden van de juiste propositie voor de juiste klant op het juiste moment via de juiste media/kanalen is belangrijker dan ooit (Davenport, 2006; Reinartz et al., 2006). Als reactie hierop zien we dat marketing probeert grip te krijgen op haar eigen proces, op haar uitgaven en op haar resultaten (Frambach & Leeflang, 2009). Nu is het niet zo dat marketing afdelingen afstappen van hun gebruikelijke manier van werken, en massaal overgaan op een meer op feiten gebaseerde, meer accountable marketingbenadering. Wel zien we dat er een grotere interesse ontstaat in het meetbaar en inzichtelijk maken van de marketing prestaties (Verhoef et al., 2003; Verhoef et al., 2009) waarbij ook wordt gekeken naar de bijdrage die de analist, de onderzoeker en de database marketeer daarin kunnen leveren (Davenport, 2006; Mitchell, 2007).
De bovengenoemde ontwikkelingen stellen ook andere eisen aan de analist, de onderzoeker en de database marketeer. Van oudsher zijn het functies die ondersteuning bieden aan bijvoorbeeld het management & directie, de campagne/verkoop teams, de marketing medewerkers, etc. Deze opdrachtgevers vroegen de analist/onderzoeker/db marketeer om een analyse, marktonderzoek en selectie uit te voeren met als resultaat een overzicht van cijfers, grafieken met klantmeningen dan wel een lijst met namen/NAW. De opdrachtgevers waren tevreden als er op tijd werd geleverd, tegen het afgesproken bedrag en het product inhoudelijk in orde was. De ‘uitvoerders’ waren professionals die vooral geïnteresseerd waren in ontwikkelingen binnen hun vakgebied en die zich druk maakten over erkenning van hun eigen werk. Slechts een enkeling dacht mee met wat de opdrachtgever daadwerkelijk met het resultaat wilde bereiken. Voor zover er blijvende eisen aan hun kennis en vaardigheden werd gesteld, lagen die op het gebied van het bijhouden van hun academische statistische kennis en het uitbreiden van de onderzoeks- en analysevaardigheden (Mitchell, 2007; Davenport & Harris, 2007).
Tegenwoordig ligt dat anders. Van analisten, onderzoekers en database marketeers wordt verlangd dat ze effectiever en efficiënter omgaan met hun tijd, geld en middelen. Bedrijven verwachten bovendien dat ze naast hun operationele taken zich ook gaan bezig houden met meer tactische en strategische vraagstukken. Opdrachtgevers tolereren niet langer een afwachtende en reactieve houding, maar verlangen een proactieve benadering waarin kansen gesignaleerd én gericht benut worden. Het principe van ‘u vraagt, wij draaien’ en het tijdig en juist opleveren van het rapport of analyse, is niet meer voldoende. Analisten, onderzoekers en database marketeers hebben niet alleen een haal-, maar inmiddels ook een brengplicht als het gaat om commercieel interessante klantinformatie (Davenport, 2006). Het accent ligt nog vaak op het produceren van het gevraagde en het opleveren van een zichtbaar resultaat, terwijl er verwacht wordt dat analisten en onderzoekers er ook voor zorgen dat er daadwerkelijk iets met de analyse- of onderzoeksresultaten gebeurt. Analisten blijken vaak prima in staat zijn om hun analyse-, onderzoeks- en selectietaken uit te voeren: de meeste analisten/onderzoekers/databasemarketeers zijn slim, worden bij voorkeur inhoudelijk uitgedaagd, en zoeken de oplossing vaak in technische middelen. Ze zijn uitermate consciëntieus, en uitermate gedreven om een oplossing te vinden en de opdracht tot een goed einde te brengen.
Helaas hebben ook wij moeten constateren dat de meeste analisten, onderzoekers en database marketeers zich nog niet kunnen meten met de bovengenoemde verwachtingen (Verhoef et al., 2009). Met name de meer ‘softe’ communicatieve en adviserende competenties lijken achter te blijven bij hetgeen er wordt gevraagd. Een herkenbaar voorbeeld is de contracteringsfase. In deze fase proberen opdrachtgever en opdrachtnemer overeenstemming te bereiken over de opdracht. In essentie is dit een interactie waarbij opdrachtnemer (in dit geval de analist of de onderzoeker) luistert vanuit een oprechte interesse, waarbij hij niet alvast vragen voor de opdrachtgever beantwoordt maar doorvraagt over wat hem opvalt. Om vervolgens aan de opdrachtgever gestructureerd voor te leggen wat hij als opdrachtnemer als de kern van het probleem of de vraagstelling ziet. Idealiter is de opdrachtnemer ook in staat om naast het inhoudelijke, verbale, verhaal, het emotionele, non-verbale, verhaal te registreren en het gevoel dat dit bij hem oproept terug te geven aan de opdrachtgever. Hiermee wordt dan het gehele (contracterings)proces gestuurd op basis van het eigen gevoel, en worden eventuele emotionele blokkades snel gesignaleerd. In de praktijk zijn deze competenties bij analisten en onderzoekers nog onvoldoende ontwikkeld, en leunt men vooral op de meer analytische en technische skills (Frambach & Leeflang, 2009).
Onze conclusie is dat van de analist/onderzoeker/dbmarketeer wordt verwacht dat hij zijn ondersteunende rol voor de marketeer, de afdeling en het bedrijf wat vaker loslaat loslaat, en dat hij een meer volwaardige rol gaat innemen als het gaat om het nemen van marketingbeslissingen door zelfstandig uitzoeken en signaleren en de verantwoordelijkheid nemen met het geven van richting dan wel energie het voortouw om de noodzakelijke verandering te realiseren.
3. Een grotere effectiviteit: vier rollen
Onze veronderstelling is dat analisten, onderzoekers en database marketeers sneller en makkelijker grotere en blijvende veranderingen weten te realiseren in het handelen, gedrag, opvattingen of werkzaamheden van collega’s/ management/klanten, als ze zelf gaan denken, handelen en werken vanuit een viertal rollen die we hieronder zullen beschrijven. De effectiviteit van een analist/onderzoeker/databasemarketeer neemt aan de hand van de vier rollen toe omdat hij:
• De opdrachtgever beter leert begrijpen. De analist verdiept zich in de opdrachtgever en leert begrijpen wat hem bezighoudt. Hij ervaart waar een opdrachtgever tegenaan loopt, wat hem bezighoudt en met welke vragen en problemen hij rondloopt.
• Beter gaat communiceren met de opdrachtgever. Doordat een analist zich de leefwereld van de opdrachtgever eigen maakt en de taal aanleert die de opdrachtgever gebruikt, zal het contact gaan verbeteren waardoor een analist beter zal aanvoelen wat belangrijk is, waar de opdrachtgever warm van wordt en hoe hij de opdrachtgever echt kan raken.
• Nieuwe analyse/onderzoeks/selectie vaardigheden gaat ontwikkelen die passen bij zijn rol. Gestuurd vanuit de vraag van de opdracht leert een analist te achterhalen waar zijn toegevoegde waarde ligt, en hoe hij de probleemstelling vertaalt naar een passende analyse-/onderzoeksmethodiek. Om vervolgens zich de technieken te leren toe te passen. Om uiteindelijk met een product/resultaat te komen dat past bij de wensen van de opdrachtgever en waarmee de opdrachtgever direct aan de slag kan.
De vier rollen onderscheiden zich op een aantal punten. De namen geven al een goede, eerste indruk; operationele rol, adviserende rol, bewakende rol en innoverende rol. De operationele rol is de basis: het fundament waarop de andere drie rollen een uitbreiding zijn. Onze hypothese is dat er een logische volgorde zit in de ontwikkeling van de rollen, maar dit nog een punt voor verder onderzoek.
De operationele rol
De operationele rol is de meest uitvoerende rol. Een analist voert zijn analyses uit, stelt zijn rapportages op en maakt zijn scoremodellen. Een onderzoeker voert kwalitatief en kwantitatief onderzoek uit, schrijft zijn bevindingen en presenteert zijn conclusies. En een database marketeer bepaalt zijn doelgroep, draait zijn selecties en zorgt dat de campagnes volgens afspraak de deur uitgaan. Allen hebben in deze rol met name te maken met directe (marketing) collega’s die voor hun werk gebruik maken van de input en de resultaten van de analisten of onderzoekers. Zoals in veel operationele functies geldt ook hier dat de belangrijkste uitdaging van deze rol ligt in het verkrijgen van grip; grip op de kwaliteit van het eigen werk, grip op de (tijds)druk vanuit de omgeving om je werk naar behoren te doen, grip op wat er met de analyse/onderzoeksresultaten gebeurt. Gelijktijdig zijn een perfecte kwaliteit en voldoende tijd (lees: juiste prioriteit) ook de belangrijkste richtlijnen voor de operationele analist/onderzoeker. De kracht van de rol zit in het opleveren van overtuigende resultaten waarmee anderen hun werk beter kunnen doen. Het is de kwaliteit en de hoeveelheid werk waarom de analist/ onderzoeker in deze rol zo sterk wordt gewaardeerd (Jayachandran et al., 2005). Vandaar de vraag om daadkracht en om productieve analisten/onderzoekers. In de operationele rol zijn analisten en onderzoekers in vergelijking met de andere rollen het meest bezig met klanten van het bedrijf. In deze rol worden de meningen, gedragingen en gegevens van klanten onderzocht, geanalyseerd en geselecteerd.
De adviserende rol
De adviserende rol is de richtinggevende rol. Op basis van feiten en resultaten uit analyses/onderzoeken/selecties komen analisten en onderzoekers met inzichten en met ongevraagd advies over de te volgen richting. Gedreven door nieuwsgierigheid en interesse proberen ze relevante ontwikkelingen te duiden en te verklaren, en de opdrachtgever daarvan te overtuigen. Allen hebben in deze rol vooral te maken met het hogere management en directies. Directie en management hebben met name behoefte aan langetermijnprognoses gebaseerd op feiten en cijfers, en ze zoeken voor hun meer strategische vraagstukken goed onderbouwde aanbevelingen. De belangrijkste uitdaging voor de adviserende rol is niet de juistheid van de aanbevelingen (dat moet als een gegeven worden beschouwd), maar het ontwikkelen van een duidelijke opdrachtgever-opdrachtnemer relatie. Adviseurs kunnen niet via een lijnverantwoordelijkheid veranderingen afdwingen, maar zijn daarvoor afhankelijk van wat een opdrachtgever met hun advies doet. Daarom is het noodzakelijk om een open, vertrouwde relatie te ontwikkelen, zodat de opdrachtgever de adviseur ziet als zijn vertrouwenspersoon waarnaar hij luistert en wiens advies hij in overweging neemt. De belangrijkste vaardigheiden in de adviserende rol zijn dan ook (1) het oprecht en aandachtig kunnen luisteren waarmee hij laat zien dat hij de situatie van de opdrachtgever volledig begrijpt, en (2) de vaardigheid om het eigen onderbuikgevoel waar te nemen en te zien als belangrijke bron van informatie, en deze informatie te delen met de opdrachtgever. In tegenstelling tot de operationele rol die vooral te maken heeft met klanten van het bedrijf, is de adviserende rol veel meer bezig met het (bij)sturen van processen, met politieke verhoudingen, met krachten die op de gewenste verandering inwerken.
De bewakende rol
De bewakende rol heeft een toetsende en controlerende aanpak. Analisten, onderzoekers en database marketeers helpen in deze rol enerzijds bij het opstellen van de targets, de verdeling van de middelen/budgetten, bij bijvoorbeeld bij het segmenteren van het klantenbestand. Anderzijds bewaken ze de naleving ervan door klantperformance indicatoren te ontwikkelen, (voortgangs)rapportages te verstrekken, en vroegtijdig verandering in de klantendatabase te signaleren (Ruijs, 2007). CFO’s, controllers en budgethouders zijn de belangrijkste opdrachtgevers (Frambach & Leeflang, 2009). Zij zijn uiteraard vooral geïnteresseerd in de financiële kant van de zaak, waarbij ze zich concentreren op de (product)omzetcijfers, budgetten, cashflow, kostenbesparingen, debiteuren, crediteuren, etc. Ofwel: alles behalve klanten, want dat is het domein van de analist/onderzoeker. Ook kijken financiële mensen vooral achteruit naar hetgeen er in het verleden is gebeurd. Zij zetten de doelen af tegen de behaalde prestaties, zoeken naar verklaringen en roepen de budgeteigenaren ter verantwoording. De belangrijkste uitdaging voor de bewakende rol is om aan te sluiten op de methodiek en de denkwereld van de financiële mensen. Dit kan door de commerciële (klant)prestaties inzichtelijk te maken (Ravichandran & Lertwonsatien, 2005), hiervoor verklaringen te bedenken en klant/budget eigenaren hiervoor verantwoordelijk te houden, en door het gehele proces te bewaken (Frambach & Leeflang, 2009). Met andere woorden, door accountability te implementeren. De bewakende rol houdt zich derhalve met name bezig met het financieel meetbaar maken van de commerciële activiteiten en met het achterhalen van het rendement van marketingacties, de diverse media, de verschillende distributiekanalen, de klantsegmenten, individuele klanten, etc. Waarbij analisten en onderzoekers de mogelijkheid hebben (veelal in tegenstelling met financiële mensen) om vooruit te kijken en voorspellingen te doen over verwachte ontwikkelingen.
De innoverende rol
De innoverende rol is vooral een rol die zorgt voor verassing. Analisten/onderzoekers/ database marketeers stellen zich de waarom-vraag, volgen hun intuïtie, en ontdekken middels een datamining analyse, een trendonderzoek of bijvoorbeeld klantsafari’s iets dat mogelijk tot een commercieel succes kan leiden (Li & Calantone, 1998; Frambach & Leeflang, 2009). In deze rol heeft de analist met name te maken met commercieel verantwoordelijken als productmanagers, klantmanagers en kanaalmanagers. Deze verantwoordelijken zoeken in het dagelijks leven naar nieuwe wegen om hun klant-/kanaal-/marktaandeel uit te breiden (Verhoef & Leeflang, 2009). De verkregen inzichten helpen daarbij, want innovaties gebaseerd op marktkennis leiden uiteindelijk tot betere bedrijfsprestaties (Li & Calantone, 1998). In de innoverende rol geldt dat de analist/onderzoeker een belangrijke uitdaging heeft in het inspireren van de opdrachtgever en het meekrijgen van zijn omgeving voor zijn nieuwe ideeën. Opdrachtgevers zitten niet te wachten op goedbedoelde, maar energievretende opmerkingen als ‘ja, maar…’. Ze willen enthousiasme en energie. En ergens in kunnen geloven, een goed verhaal, een flitsend betoog. Men zit niet te wachten op een uitgebreide (misschien een wat saaie) wetenschappelijk uiteenzetting. Een innoverende analist/onderzoeker verschuilt zich dan ook niet achter cijfers en grafieken, maar houdt een begrijpelijk en inspirerend verhaal waarmee hij laat zien dat opdrachtgevers hun werk beter kunnen doen. Zijn verrassende (klant)inzichten zorgen ervoor dat opdrachtgevers hun beleid, producten en klantbenadering kunnen aanpassen. Niet de volledigheid en uitgebreidheid, maar snelheid en verrassing maken dat de analist/ onderzoeker in deze rol zo sterk wordt gewaardeerd. Vandaar de vraag om energieke en inspirerende analisten/onderzoekers. Tot slot, zijn innoverende analisten/onderzoekers vooral bezig met productinnovatie, merkinnovatie, met witte vlekken in het klantenbestand en binnenhalen van nieuwe klanten (Chaston, 2004).
4. Inzicht in het succes van de vier rollen.
Voordat we ingaan op de inzichten die nodig zijn om succesvol te worden in elk van de vier rollen, bespreken we eerst wat we verstaan onder de term ‘succes’.
Een analist/onderzoeker in een operationele rol is succesvol als hij in staat is om zijn werk efficiënt en effectief uit te voeren. Dat wil zeggen, een hoge productie te halen, binnen de gemaakte tijdsafspraken, met een uitstekende kwaliteit en tegen een betaalbare prijs.
In de adviserende rol heeft een analist succes enerzijds als hij serieus wordt genomen en er naar hem wordt geluisterd. Anderzijds als hij in staat is om zichtbare en merkbare veranderingen door te voeren ten voordele van de klant en het bedrijfsresultaat.
In de bewakende rol heeft een analist/onderzoeker succes als hij in staat is om juiste verklaringen te geven, oorzaken te achterhalen en op basis daarvan acties, processen, afdelingen, etc. te helpen verbeteren door minder geld uit te geven danwel meer rendement binnen te halen.
En in de innoverende rol is een analist/onderzoeker succesvol als zijn (klant)inzichten en ideeën leiden tot commercieel succesvolle (product/merk)introducties, het aanboren van nieuwe markten, of het succesvol inzetten van nieuwe media maar ook bijvoorbeeld flinke kostenbesparingen.
Om die grotere effectiviteit te behalen is het nodig dat een operationele analist of onderzoeker bij zichzelf te rade gaat en inzicht krijgt in wat ‘verborgen bestuurders’ zijn. Dat wil zeggen, het achterhalen van zijn emotionele blokkades die belemmeren dat hij duidelijk kan aangeven waar zijn grenzen liggen en die ervoor zorgen dat hij niet zijn prioriteiten durft aan te geven zonder zich schuldig te voelen. Eenmaal bewust van deze patronen zal het hem lukken om grip te krijgen op zijn eigen houding ten aanzien van tijd, zijn gekozen prioriteit en de druk vanuit omgeving, zonder het contact met de omgeving te verliezen en in een stresssituatie te komen.
Een adviserende analist/onderzoeker zal een grotere effectiviteit te bereiken door bewust het adviesproces in al zijn verschillende fasen te doorlopen (Block, 2006). Gelijktijdig zal hij moeten leren ‘varen’ op zijn eigen innerlijke, emotionele kompas. Doel van dit laatste is vooral om het vertrouwen van de opdrachtgever te verdienen door gericht contact te leggen, aandachtig te luisteren, de vraagstelling in te kaderen, gezamenlijk visies ontwikkelen, en door toezeggingen te doen (Maister et al., 2005). Hierbij worden de innerlijke waarnemingen van wat er gezegd wordt, maar ook van wat er non-verbaal wordt gecommuniceerd teruggegeven aan de gesprekspartner, met als doel om het bespreekbaar te maken en om inzicht te krijgen in de oorzaak ervan. Het bewust doorlopen van het adviesproces maakt dat de adviserende analist/onderzoeker regie houdt over het gehele proces en bewust de instemming van de opdrachtgever krijgt om een volgende stap te nemen.
De bewakende rol bereikt een hogere effectiviteit door inzicht te verkrijgen in het belang van het budgettaire proces en het bijbehorende politieke krachtenspel. Het betekent leren hoe het is om personen/afdelingen ‘accountable’ te maken door het toekennen van budgetten gekoppeld heldere doelstellingen. Vervolgens zaak om met behulp van de juiste metrices/rapportages/kpi’s de voortgang te volgen (Ravichandran & Lertwongsatien, 2005), zonodig specifieke activiteiten te evalueren en de verantwoordelijken te wijzen op hun afspraken. Eenmaal bewust van hoe het politieke krachtenspel werkt, is het zaak om als analist/onderzoeker de verhoudingen naar in je eigen voordeel te laten werken. En in te schatten op welke belangrijke momenten steun te zoeken/krijgen van de financiële mensen.
Een innoverende analist/onderzoeker wordt effectiever als hij in staat is de juiste klantinzichten te genereren. Daarvoor zal hij zich moeten verdiepen in het onderwerp. En hij zal zichzelf vooral de ruimte moeten creëren om nieuwe dingen uit te kunnen proberen, en een werkwijze moeten introduceren om van de experimenten te leren. Uiteindelijk gaat het erom dat de analist/onderzoeker snel en goed leert inschatten welke trends goed vallen bij zijn opdrachtgevers en bovendien een grote kans maken om commercieel succesvol te worden. Daarvoor zal hij moeten samenwerken met anderen om ook van hen te leren waarom iets wel/niet een succes is geworden (Davenport, 2006; De Luca & Atuahene-Gima, 2007). Eenmaal bewust van de kracht van samenwerken, is het vooral zaak om los te komen van zijn natuurlijke kritische (ja-maar) houding en te beseffen dat met relatief eenvoudige middelen een hoop positieve energie wordt gegenereerd.
5. Kennis en kunde voor elk van de vier rollen
Voor de vier verschillende rollen is ook specifieke kennis & kunde nodig. In een operationele rol wordt van een analist/onderzoeker verwacht dat hij leert om grip te krijgen op zichzelf en leert om zaken in zijn omgeving gedaan te krijgen. Daarvoor zijn zaken als time management, presentatie- en schrijftechnieken en het overtuigen/manipuleren van de omgeving, van belang. Leren reflecteren helpt om bij het identificeren van de vraag achter de klantvraag, en om te kunnen varen op het eigen, interne kompas. Om in de operationele rol de gevraagde selecties, analyses en onderzoeken goed te kunnen uitvoeren is voldoende kennis en vaardigheid nodig van de standaard technieken als SQL, Excell en klassieke (beschrijvende en toetsende) statistiek als (log) lineaire regressie, de beslisboom technieken en de traditionele respons- en conversie analyse. Daarnaast is aandacht nodig voor basis onderzoeksmethodieken als het opzetten van een split run (om bijvoorbeeld twee proposities tegen elkaar af te testen) en het inrichten van een experimenteel design (geschikt om bijvoorbeeld de effecten van allerlei ingrepen vast te stellen).
In de adviserende rol wordt van de analist/onderzoeker verwacht dat hij kennis en ervaring heeft met advies- en veranderingstrajecten. Daarvoor zijn zaken als het aangaan en onderhouden van een relatie van belang, en het leren winnen van vertrouwen, en het leren communiceren gerelateerd aan het overwinnen van weerstand. Voldoende ervaring opdoen om een business vraag op de juiste manier te vertalen naar een analyse vraag waarmee waardevolle inzichten worden opgeleverd, is eveneens een belangrijk leerpunt in deze rol. Om in de adviserende rol de gevraagde selecties, analyses en onderzoeken goed te kunnen uitvoeren is voldoende kennis en vaardigheid nodig van technieken als longitudinaal onderzoek, forecasting en dashboards/matices (Frambach & Leeflang, 2009). Om de veranderingen te kunnen meten en om relevante ontwikkelingen te kunnen signaleren is het zaak dat een analist in staat is om methodiek op te zetten waarmee hij bijvoorbeeld een begin- en eindsituatie met elkaar kan worden vergeleken, en het verschil (delta) kan verklaren.
In de bewakende rol wordt van de analist/onderzoeker verwacht dat hij kennis en ervaring heeft met accountability en performance management. Daarvoor is het nodig dat hij een mate van politieke sensitiviteit ontwikkelt, inzicht heeft in strategische besluitvormingsprocessen en de geldstromen binnen een organisatie leert kennen (Verhoef & Leeflang, 2009). Ook zijn vaardigheden nodig op gebied van het opstellen en controleren van kpi’s/meters, het toewijzen van verantwoordelijkheden en mensen aanspreken op hun verantwoordelijkheden/gemaakte afspraken (Ruijs, 2007). Om in de bewakende rol de gevraagde selecties, analyses en onderzoeken goed te kunnen uitvoeren is voldoende kennis en vaardigheid nodig van toetsende statistiek en voorspellende technieken, en ook van het opstellen van matrices/rapportages. Om oorzaken te kunnen achterhalen en om verklaringen te kunnen geven is het nodig dat de analist onderzoeksmethodieken leert toepassen waarbij hypotheses worden getoetst en causale verbanden inzichtelijk worden gemaakt.
In de innoverende rol wordt van de analist/onderzoeker verwacht dat hij een directe bijdrage levert aan het succes van innovaties. Daarvoor is kennis nodig van innovatie-processen. Een analist zal zich nog meer in zijn doelgroep en concurrenten moeten verdiepen (De Luca & Atuahene-Gima, 2007). En hij zal bovenal de vaardigheid moeten ontwikkelen om inzichten te selecteren die potentieel commercieel succesvol zullen zijn. Verder is het leren aangaan van een constructieve samenwerking een belangrijk leerpunt. Om in de innoverende rol de gevraagde selecties, analyses en onderzoeken goed te kunnen uitvoeren is voldoende kennis en vaardigheid nodig van relatief snelle onderzoekstechnieken als beschrijvende statistiek, datamining en OLAP-tooling. En misschien daarnaast ook van technieken die meer ingaan op verklaren van zoals kwalitatief onderzoek en trendwatching. En natuurlijk de meer recente technieken als web analyse, behavioral targeting, realtime modeling en customer value analysis. De te leren onderzoeksmethoden zijn vooral hypothese genererend, exploratief en gericht op het zichtbaar en begrijpelijk maken van het (klanten)profiel.
6. De praktijk
De in dit artikel beschreven oplossingsrichting voor het vergroten van de impact van een analist/onderzoeker is in de praktijk getest onder deelnemers aan een serie workshops waarin de vier rollen aan de orde kwamen. De serie bestond uit totaal 12 (vrij)dagen, verspreid over een periode van 6 maanden. Naast een kennismakings/introductiedag, een dag Persoonlijke Effectiviteit en een dag Basic Modeling waren er 8 dagen gereserveerd voor rolspecifieke workshops. Voor elke rol waren er twee dagen gereserveerd: een dag voor de interpersoonlijke & adviesvaardigheden en de andere dag voor de meer technische analyse vaardigheden. De serie eindigde met een slotdag met evaluatie en uitreiking van certificaten.
De negen deelnemers hadden verschillende achtergronden. Twee waren afkomstig van een bedrijf uit de energiesector, drie deelnemers werkten allen bij eenzelfde bedrijf in de zakelijke dienstverlening, drie waren afkomstig van verschillende verzekeraars, en een deelnemer werkte bij een webwinkel. De leeftijd van de meeste deelnemers was tussen 30 en 40 jaar. Het ging om drie vrouwen en zes mannen. Allen hadden een midcareer stadium. Er was 1 leidinggevende en de rest waren operationeel analisten en database marketeers. De oudste en jongste deelnemer hadden een relatief zware statistische achtergrond. De overige deelnemers hadden latente interesse en minder ervaring met statistiek en het maken van modellen.
Geheel in de fact-based traditie is er een nulmeting gehouden aan het begin van de workshopreeks op basis van een inventarisatie onder directe leidinggevenden en opdrachtgevers. Deze werden gevraagd naar hun oordeel over de kwaliteiten van de analist en naar de verwachtingen ten aanzien van het resultaat van de workshopreeks. Aan het eind van de serie is aan de deelnemers gevraagd wat zij zelf van de workshops vonden, maar ook welke reacties zij tot nu toe op hun ‘nieuwe manier van werken’ hadden gekregen. Na een periode van 6 maanden is opnieuw een enquête verstuurd naar de leidinggevenden waarin opnieuw hun oordeel gevraagd werd over de kwaliteiten van de deelnemer. Bovendien werd gevraagd in hoeverre de verwachtingen ten aanzien van de serie workshops waren ingelost.
De verwachtingen van leidinggevenden en opdrachtgevers ten aanzien van de workshopreeks laten zich samenvatten als: ‘Na de workshops moet de analist een verbetering laten zien op gebied van:’
• Kennis van data, systemen, markt, statistiek, modellen
• Business problemen naar analyse vragen kunnen vertalen; problematiek onder woorden kunnen brengen; de kern/essentie eruit kunnen halen
• Analyses goed kunnen uitvoeren; analyse als een middel en niet als een doel op zich zien
• Resultaten begrijpelijk en overzichtelijk rapporteren/presenteren; zien waar verbanden liggen en deze kunnen interpreteren; ongevraagd informatie delen; opleveren relevante insights; een heldere en krachtige communicatie
• Op maat advies kunnen geven; bevindingen omzetten tot advies; analyses weten te vertalen naar commerciële kansen
• Tot actie doen aanzetten; aangeven wat de consequenties zijn en wat een bedrijf er mee kan doen; doelstellingen van de opdrachtgever goed voor ogen houden,
• Zelf signaleren van/op zoek gaan naar nieuwe mogelijkheden en innovaties.
Na afloop is de workshopreeks geëvalueerd en is de deelnemers gevraagd om opnieuw reacties van leidinggevenden en opdrachtgevers te verzamelen.
• Hij heeft nu meer begrip voor zijn omgeving dankzij de workshops. Er is een betere samenwerking met collega’s en opdrachtgevers ontstaan. Hij is een zelfbewustere analist geworden.
• De analist is veel beter in staat om de opdracht goed af te bakenen. En om duidelijke deadlines te communiceren en deze zelf te halen.
• Hij is een meer complete analist geworden. De koppeling tussen de rol van adviseur en tool marktanalist is heel nuttig geweest.
• De analist heeft veel meer inzicht in het vak gekregen. Begrijpt zijn functie/rol binnen de organisatie. Heeft meer inzicht in zijn eigen verbeterpunten.
• De analist is beter in staat om te luisteren en weg te blijven van het direct geven van een oplossing.
• Hij toetst nu eerst de gemaakte afspraken hetgeen veel onnodig werk voorkomt.
• De adviesvaardigheden zijn sterk verbeterd. Ik heb verbeteringen waargenomen bij het innemen van een standpunt en het overtuigen van collega’s.
• De analist heeft meer inzicht gekregen in het gebruik van tools en analysemogelijkheden. Zijn groei in skills om de communicatie (verhaal en presentaties) met collega’s en opdrachtgevers te optimaliseren uit zich in zelfbewustere, heldere klantgerichte presentaties.
• Een collega marketeer zag hem een presentatie begeleiden en gaf aan hij krachtiger en positief optrad. Als leidinggevende heb ik ook het verschil gemerkt. Dankzij de cursus is hij uitgenodigd om mee te werken aan een nieuw aanvalsplan.
Vanwaar de zichtbare veranderingen? Gebleken is dat gedurende de serie workshops in ieder geval is voldaan aan de gebruikelijke voorwaarden om te veranderen (Den Boer 1999):
• Er was druk van buitenaf om te moeten veranderen. Oftewel, er was sprake van een zekere noodzaak om de oude manier van werken los te laten en iets nieuws te leren. De meeste aanmeldingen voor de reeks waren geïnitieerd door de directe leidinggevenden, of waren tot stand gekomen in overleg met de leidinggevende. En dus moet er gesproken zijn over de noodzaak voor het volgen van de workshops. De druk van buitenaf was nog het meest zichtbaar in de resultaten van de enquête waarin de verwachtingen ten aanzien van de cursusresultaten voor bijna iedere deelnemer expliciet waren vermeld.
• De deelnemers wilden zelf veranderen: ze waren bereid de oude werkwijze los te laten en in te ruilen voor een andere. De deelnemers waren erg gemotiveerd gedurende workshops, en zeer geïnteresseerd in elkaars ervaringen. De oefeningen en de rollenspelen werden met zeer veel plezier uitgevoerd. Feedback werd op een constructieve manier meegenomen in de vervolgsessies.
• De deelnemers konden veranderen. In de werkomgeving van elk van de deelnemers werd voldoende ruimte gegeven voor veranderingen.
• De deelnemers durfden te veranderen. Tijdens de workshops lieten de deelnemers in oefeningen, rollenspelen en huiswerk zien dat ze het lef hadden om hun oude werkwijze los te laten. Maar ook buiten de cursus hebben de deelnemers laten zien de aandachtspunten bewust te willen verbeteren door ze in de praktijk uit te proberen. Dit leverde hen regelmatig positieve reacties op.
7. Conclusies
In dit artikel hebben we een mogelijke oplossingsrichting uiteengezet voor het vergroten van de impact van een analist of onderzoeker. We onderscheiden vier rollen die ertoe moeten bijdragen dat de analist of onderzoeker:
• De opdrachtgever beter leert begrijpen;
• Beter gaat communiceren met de opdrachtgever;
• Nieuwe analyse/onderzoeks/selectie vaardigheden ontwikkelt die past bij zijn rol.
De aanpak is in de praktijk getoetst onder negen deelnemers aan een workshopreeks. De belangrijkste conclusie is dat de cursus een voor de buitenwereld zichtbaar en een voor de deelnemer een merkbaar effect heeft. We zien dat deelnemers na het volgen van de cursus sneller en makkelijker (grotere en blijvende) veranderingen weten te realiseren in het handelen, gedrag, opvattingen of werkzaamheden van collega’s/ management/klanten, dan dat ze dat deden voor de cursus. Geconcludeerd mag worden dat het zelf gaan denken, handelen en werken vanuit de vier rollen er toe bijdraagt dat de impact van de deelnemers in hun werkomgeving is toegenomen.
Referenties
Boer, C., den (1999). Woorden én daden. Amersfoort: Twynstra Gudde Management Consultants.
Blattberg, R. C., B. Kim & S. A. Neslin (2008). Database Marketing: Analyzing and Managing Customers. New York: Spinger.
Block, P. (2006). Feilloos Adviseren, een praktische gids voor adviesvaardigheden. Academic Service, Den Haag.
Davenport, T. H. (2006). Competing on Analytics. Harvard Business Review, 99-107.
Davenport, T. H. & J. G. Harris (2007). Competing on Analytics: The New Science of Winning. Boston: Harvard Business School Press.
De Luca, L. M. & K. Atuahene-Gima (2007). Market Knowledge Dimensions and Cross-Functional Collaboration: Examining the Different Routes to Product Innovation Performance. Journal of Marketing, 71, 95-112.
Frambach, R. & P. S. H. Leeflang (2009). Marketing aan de top: 10 problemen, 10 oorzaken, 10 oplossingen. Amsterdam: Pearson Education Benelux.
Jayachandran, S., S. Sharma, P. Kaufman & P. Raman (2005). The Role of Relational Information Processes and Technology Use in Customer Relationship Management. Journal of Marketing, 69, 177-192.
Li, T. & R. J. Calantone (1998). The Impact of Market Knowledge Competence on New Product Advantage: Conceptualization and Empirical Examination. Journal of Marketing, 62, 13-29.
Maister, D. H., C. H. Green & R. M. Galford (2005). De Vertrouwde Adviseur. Den Haag: Academic Service.
Mitchell, I. (2007). Operationalizing Customer Intelligence in The Contact Center. Business Communications Review, 37, 34-39.
Ravichandran, T. & C. Lertwongsatien (2005). Effect of Information Systems Resources and Capabilities on Firm Performance: A Resource-Based Perspective. Journal of Management Information Systems, 21, 237-276.
Reinartz, W., M. Krafft & W. D. Hoyer (2004). The Customer Relationship Management Process: Its Measurement and Impact on Performance. Journal of Marketing Research, 16, 293-305.
Ruijs, P., 2007. Vijf handvatten om accountability aan te pakken. Tijdschrift voor Marketing, Week 6, 44-47.
Verhoef, P. C., P. N. Spring, J. C. Hoekstra & P. S. H. Leeflang (2003). The commercial use of segmentation and predictive modeling techniques for database marketing in the Netherlands. Decision Support Systems, 34, 471-481.
Verhoef, P. C., J. C. Hoekstra, H. van der Scheer & VODW Marketing (2009). Competing on analytics: Status quo van Customer Intelligence in Nederland. Onderzoeksrapport CIC 200902. Groningen: Customer Insights Center.
Verhoef, P.C. & P. S. H. Leeflang (2009). Understanding the Marketing Department’s Influence Within the Firm. Journal of Marketing, 73, 14–37.
English summary:
Various studies conclude that the marketing discipline is losing its influence within the firm. In this article we argue that quantitative marketing analysts and market researchers can help regaining at least a part of this influence. However, a necessary requirement for this to happen is that they succeed in improving their own impact within the firm. In this article we develop a method that aims to improve the impact of a marketing analyst or a market researcher using four roles: the operational role, the advisory role, the accountability role and the innovative role. Each of these roles are associated with specific soft skills and analytical tools that can help the marketing analyst and the market researcher reaching that goal. The proposed method is tested in practice, with positive results.
Key words: the influence of marketing within the firm, accountability, marketing intelligence, the impact of marketing analysts and researchers, acting pro actively.
Vliegt de marketeer de deur uit?
Gert Haanstra, CustomerBase Jaarboek 2002, F&G Publishing, Amersfoort, Februari 2002, blz 68 t/m 70.
Printversie: Vliegt de marketeer de deur uit?
Customer Relationship Management ligt onder vuur. Voor een deel is dat terecht. Want er is teveel beloofd, en er wordt (nog steeds) te weinig waargemaakt. De cijfers en publicaties over mislukte CRM-software implementaties zijn hier een voorbeeld van. Maar ondanks deze negatieve verhalen, blijft CRM als business strategie een belangrijke schakel bij het creëren, ontwikkelen en onderhouden van levenslange klantrelaties. In dit verhaal proberen we deze positieve kanten te belichten. We gaan in op wat CRM-technologie ons de afgelopen jaren aan functionaliteit heeft opgeleverd. En hoe CRM het marketingvak heeft beïnvloed.
Wat functionaliteit betreft, heeft de CRM-technologie zich sterk ontwikkeld. Vroeger ondersteunde de technologie de marketeer in het uitvoeren van marketingacties. Alles wat men nodig had, was een database, een analyse tool om prospects te selecteren en een campagne manager om de marketingacties uit te kunnen voeren. De huidige CRM- technologie neemt gedeeltelijk de marketingfunctie over door zelf beslissingen te nemen, buiten de marketeer om. Het is sterk gericht op het voorspelbaar en controleerbaar maken van de interactie met de individuele klant, waarbij kansen en bedreigingen continu worden gemonitored en realtime wordt gesignaleerd, om vervolgens direct te worden benut.
De marketingfunctie is onder invloed van de CRM-technologie sterk veranderd. Product- en communicatiemanagers hielden zich sterk bezig met de communicatie richting klanten. Hun werk leunde sterk op creativiteit en onderbuikgevoel, en het halen van verkooptargets. Nu willen bedrijven klantsegmentmanagers met een winstverantwoordelijkheid die ernaar streven om een beheersbaar en controleerbaar marketingproces neer te zetten met bewezen, meetbare financiële resultaten. Met een effectieve CRM-organisatie willen bedrijven hun (marketing) investeringen beter afstemmen op de (te verwachten) opbrengst van de klant en de (te verwachten) duur van de relatie met de klant.
We beschrijven de veranderingen aan de hand van een drietal fasen: database marketing, one-to-one marketing en realtime marketing. De fasen markeren een breekpunt in nieuwe CRM-functionaliteit en een veranderende marketingfunctie.
Database marketing, marketing in control
Met de komst van database marketing midden/eind jaren tachtig, kregen marketeers hun greep terug op de marketinguitgaven. Eindelijk was er een instrument om de effectiviteit van de marketinginspanningen te achterhalen. De doeltreffendheid van (uitgaande) marketing- activiteiten werd verbeterd door te kijken welke klant het beste past bij een bepaald product of dienst. Of anders gezegd, door te voorspellen wie in alle waarschijnlijkheid het specifieke product zal kopen.
Het leidde tot een directe kostenbesparing doordat hetzelfde commerciële resultaat of zelfs een beter resultaat kon worden bereikt met aanzienlijk minder marketingcampagnes en/of contactfrequenties. Analytische CRM was nodig voor de “vertaalslag” van het ontdekken van interessante verkoopkansen in het klantenbestand, naar het concreet verbeteren van marketingacties.
Marketeers verloren terrein. Intuïtie maakte plaats voor techniek. En hoewel ze nog steeds eindverantwoordelijk bleven voor het commerciële resultaat, waren ze voor het grootste deel van hun prestaties afhankelijk van het werk van analisten en selecteurs. Ze moesten leren luisteren naar de klantendatabase die (on)gevraagd kwam met commercieel interessante en verrassende klantengroepen. En onder druk van het moeten behalen van commercieel succes, was dat vaak minder vrijblijvend dan het in eerste instantie lijkt.
One-to-One Marketing, klanten voeren de regie
Na de fase van database marketing nam de klant zelf de regie in toenemende mate over. Vooral met de opkomst van de nieuwe, interactieve distributiekanalen als e-mail, Internet en mobiele telefonie, zocht de klant vaker zelf het contact met het bedrijf en ging, vaak goed geïnformeerd, actief op zoek naar het geschikte product. Bedrijven reageerden hierop met One-to-One Marketing. Hierbij wordt met een persoonlijk aanbod ingespeeld op de wensen van individuele klanten, op een moment dat zij hiervoor ontvankelijk zijn.
Met One-to-One marketing nam de verkoop zichtbaar toe. Inkomende klantcontacten boden prima cross-selling en up-selling mogelijkheden die, aangestuurd door onderliggende business-regels of voorspellingsmodellen, onmiddellijk konden worden benut.
Technisch gezien moest relevante klantkennis, op het moment dat de klant contact had met het bedrijf, onmiddellijk leiden tot een gerichte marketingactiviteit. Op zich is dat geen probleem. Voorwaarde is wel dat relevante informatie tussen de verschillende applicaties, databases en systemen onderling moet worden uitgewisseld. Daarnaast moet het aanbod van het bedrijf een reactie zijn op de informatie die de klant zelf heeft gegeven.
Marketeers konden zich met One-to-One marketing volledig gaan richten op het onderhouden van de relaties met hun eigen klantgroep of klantsegment. In feite hoefden ze niet meer perse één product te pushen, maar konden ze de klant laten ‘beslissen’ welk product werd aangeboden. Overigens hoefde dit niet perse een productaanbod zijn, maar kon het bijvoorbeeld ook een retentie aanbod of kennismakingsaanbod zijn. Belangrijk voordeel was de mogelijkheid om het aanbod te prioriteren op basis van de hoogste winstmarge of winstbijdrage. Zodat daadwerkelijk begin werd gemaakt met het vergroten van de totale winst over de klantgroep.
Realtime Marketing, techniek aan de macht?
In de huidige fase van Realtime Marketing proberen bedrijven vooral beter grip te krijgen op het dynamische interactieproces met de klant. Doel is om de uitkomsten van klantcontacten te beïnvloeden, om zo een optimaal (commercieel) resultaat te kunnen behalen.
Duidelijk is wel dat dit met de moderne CRM-technologie zou kunnen lukken. De technologie stelt de bedrijven in staat om resultaten van de klantinteracties te voorspellen, en kan de uitkomsten rechtstreeks beïnvloeden. Het is in staat zich snel aan te passen aan de veranderende situatie van de klant en stelt bedrijven in staat om er continu van te leren. Uiteraard om hiermee het totale rendement op klantrelaties te maximaliseren over een langere periode van elke individuele klant.
Voor marketeers betekent dit een pro-actieve voorbereiding op de kansen en bedreigingen die zich plotseling kunnen voordoen. Verschillende proposities staan klaar om te worden gebruikt, maar de feitelijke afweging tussen de proposities gebeurt tijdens het klantcontact. Een marketeer zal dan ook een deel van zijn beslissingsbevoegdheid moeten inleveren ten gunste van ‘intelligente’ CRM-software. Het aansturen van de interacties met het berekenen van de juiste propositie wordt automatisch uitgevoerd. Hierbij wordt constant bekeken of er kansen/bedreigingen zijn, om op basis van de complete (inclusief de meest recente) klantinformatie, met een passende propositie te komen. Waarbij de technologie is gericht op de maximale verwachte winstbijdrage, en de dynamische afweging tussen verschillende doelstellingen zoals cross-selling, up-selling, kostenbesparingen, trouw en klantwaarde.
Deze manier van interacties aansturen betekent technisch gezien de introductie van Realtime Monitors en Realtime Alerts als aanvulling op de bestaande CRM-functionaliteit. Ze worden ingezet om interactie strategieën voortdurend te evalueren, met als doel de marketeer te informeren en hem de gelegenheid te geven de proposities te verbeteren. In de ultieme situatie komt de software zelf met een voorstel ter verbetering van de interactie strategie.
Conclusie
Bedrijven willen met CRM het rendement op hun (potentieel) meest winstgevende en trouwe klantrelaties vergroten. Maar wat heeft CRM ons tot nu toe opgeleverd?
Ooit bracht database marketing de marketeer terug in het zadel. Het hielp de marketeer de kosten in de hand te houden en de effectiviteit van zijn inspanningen te vergroten. Door gericht de klanten met het juiste aanbod te benaderen en door de marketingacties op elkaar af te stemmen werd marketing een stuk efficiënter en effectiever.
Vervolgens verschoof de macht naar de klant, die bepaalde wat en wanneer er contact werd gezocht met een bedrijf. Gelukkig hielp One-to-One marketing de marketeer effectief te reageren op de wensen van de klant; vanaf elke locatie en op elk willekeurig moment. Daarnaast stimuleerde het een meer brede klantbenadering gericht op het vergroten van de totale klantwaarde in plaats van het verhogen van de productverkoop.
Na de marketeer en de klant, krijgt tegenwoordig de technologie de overmacht. Het ontneemt zelfs de marketeer het recht van ‘spreken’ op het moment dat een beslissing wordt genomen over welke propositie de klant moet krijgen. De technologie is erop gericht om effectief de kansen tijdens klantinteracties te signaleren en direct te benutten, zonder ingrijpen van de marketeer.
En dus lijken we ons streven om de (marketing)investeringen beter af te stemmen op de (te verwachten) opbrengst van de klant en de (te verwachten) duur van de relatie met de klant, heel goed te kunnen halen als we eenmaal de marketeer buiten de deur hebben gezet. Tijd om als marketeer iets terug te doen.
Call centers tussen droom en daad
Gert Haanstra en Martijn Jansen, Call Center Magazine, F&G Publishing, Amersfoort, Nr. 3, 2002
Printversie: Call centers tussen droom en daad
Ondanks alle mooie beloften van de afgelopen jaren is er een grote kloof tussen de CRM-dromen van veel call centers en hun CRM-prestaties tot nu toe. Directe oorzaak is dat bedrijven flink hebben geïnvesteerd in de aanschaf van CRM-software, maar dat ze bij de implementatie te weinig aandacht hebben besteed aan het noodzakelijke, onderliggende ontwikkelingstraject voor management en medewerkers. In dit verhaal beschrijven wij hoe de kloof tussen CRM-droom en CRM-daad kan worden gedicht. Wij laten zien hoe groot de kloof is, en op welke wijze die kan worden overbrugd, en wie dat zou moeten doen.
Wat is de kloof tussen droom en daad?
Veel call centers dromen van tevreden en loyale klanten die goed en op een goede manier worden geholpen. Ook dromen de call centers van lachende medewerkers die met plezier hun werk doen. En van een optimaal commercieel resultaat dat wordt verkregen met passende, winstgevende proposities, en dat kan worden benut op het moment dat zich kansen voordoen. Contacten met klanten worden optimaal benut en er is voldoende inzicht in de klant- en bedrijfsprestaties.
De dagelijkse realiteit is echter dat sinds de invoering van het nieuwe CRM-systeem wel kosten worden gemaakt, maar dat daar slechts in enkele gevallen extra opbrengsten tegen overstaan. De medewerkers hebben stress omdat zij per dag verplicht een aantal uren met het pakket moeten werken. Zij voelen zich gecontroleerd door de gedetailleerde rapportages. En de klanten blijven geïrriteerd omdat zij nog steeds te pas en te onpas aanbiedingen krijgen waarop zij niet zit te wachten.
Wil men droom en daad bij elkaar brengen, dan zullen de verwachtingen van het management (beter) moeten worden afgestemd op de belevingswereld van de medewerkers. Het call center management droomt van het effectief en efficiënt afhandelen van de klantcontacten. En het CRM-systeem werd aangeschaft om deze doelstellingen te realiseren door ze direct en inzichtelijk te koppelen aan de doelen en prestaties van de afdelingen en die van de individuele medewerkers. En dus stuurt het call center management haar medewerkers aan op de te behalen beltijden, de gewenste serviceniveaus en de aan te bieden klantproposities, etc.
Medewerkers voelen dit anders. Zij beantwoorden voor zichzelf de vragen: Moet ik ermee werken? Wil ik dat wel? En kan ik ermee werken?
Is het noodzakelijk om met CRM te werken?
Hierbij gaat het vooral om of de medewerkers inzien dat CRM als een klantgerichte manier van werken (nog steeds) een geschikte oplossing is voor het probleem waar het call center mee worstelt. En dus of zij de noodzaak voelen om zelf met het CRM-systeem mee aan de slag te gaan.
De meest overtuigende argumenten hiervoor komen vaak van klanten zelf. Laat dan ook de klant duidelijk maken wat hij van het bedrijf verwacht. Medewerkers worden bijvoorbeeld geconfronteerd met klanten die klagen en uiteindelijk ‘weglopen’. Dus waarom niet een klant of een ex-klant aan de (bestuurs)tafel zetten en hem de agenda laten bepalen door het management (letterlijk) te confronteren met zijn wensen en klachten. Vervolgens kan het management aan de medewerkers duidelijk maken hoe zij op een CRM-manier van werken en ondersteund met de software, het probleem kunnen oplossen.
Ben ik bereid om met CRM te werken?
Of eigenlijk, ‘what’s in it for me?’ Medewerkers zullen bij zichzelf te rade gaan en zich afvragen welke voordelen het hen gaat opleveren als zij met CRM aan de slag gaan. Helpt het mij om mijn werk beter te doen, en om het leuker te maken? Het beste is om medewerkers zelf de gestelde bedrijfs- en klantdoelen te laten ‘vertalen’ in persoonlijke doelstellingen. Op die manier wordt het voor de medewerkers direct duidelijk hoe zij een bijdrage kunnen leveren aan de doelen. Bovendien helpt dit de medewerkers om zich het CRM-gedachtegoed eigen te maken.
Individuele medewerkers kunnen extra worden geprikkeld met een direct financieel voordeel zoals een salarisverhoging, een bonus of extra vrije dagen. Hiermee wordt een deel van de winst voor het bedrijf doorgegeven aan de medewerker, misschien om de laatste twijfel weg te nemen of ter compensatie voor mogelijke extra inspanningen.
Kan ik überhaupt met CRM werken?
Medewerkers vragen zich af of zij met behulp van de software de nieuwe manier van werken eigen te maken. Het vraagt flexibiliteit en aanpassing van de medewerkers. Het management kan hierop inspelen door voldoende aandacht te besteden aan de optimale match tussen wat de klant en het call center vragen én wat de medewerkers willen en kunnen.
Onze ervaring is dat een goede training de medewerkers helpt om zelfstandig met klantinformatie om te gaan. Immers, met de introductie van CRM valt de directe aansturing door call center managers weg en krijgen medewerkers meer verantwoordelijkheden. Ook moeten ze meerdere rollen aankunnen (bijvoorbeeld verkopen, servicen, of klanten behouden). Medewerkers zullen de vaardigheid moeten hebben om goed met de CRM-software om te gaan, en om bovendien flexibel te kunnen inspelen op allerlei wensen van de klant. Eigenlijk worden de medewerkers ‘zelfstandige’ ondernemers, die hun eigen kansen moeten leren zien en risico’s moeten durven nemen.
Hoe de kloof te overbruggen?
Op welke manier kunnen management en medewerkers bij elkaar worden gebracht? Voor CRM is het nodig dat het management en de medewerkers gezamenlijk een ontwikkeling doormaken. Het call center moet leren om de (potentieel) meest winstgevende en loyale klanten te werven, te onderhouden en te behouden. Niet alles wordt in één keer gedaan, maar gebeurt stap-voor-stap. De tussentijdse resultaten zijn bepalend voor de richting van het vervolgtraject. Daarbij wordt voldoende tijd ingeruimd voor evaluatie en reflectie. De momenten van ‘rust’ moeten ervoor zorgen dat de ervaringen ook beklijven en dat het behaalde resultaat als zodanig wordt geaccepteerd, en dat, als het nodig is, wordt bijgestuurd. Een belangrijk voordeel van deze werkwijze is dat medewerkers en management voldoende gemotiveerd blijven voor de volgende stap. En dat er sprake is van een leereffect.
De medewerkers leren gedurende het ontwikkelingstraject om de (potentieel) meest winstgevende en loyale klanten te werven, te onderhouden en te behouden. Dit betekent dat zij van het management voldoende ruimte moeten krijgen om iets uit te proberen, zodat ze niet bang zijn om fouten te maken. Daarnaast is een cultuur vereist die gericht is op samenwerking. Elkaar helpen en er samen beter van worden. Verder is een tijdige en heldere communicatie over de (gezamenlijke) ambities/intenties van belang.
Een voorwaarde voor succesvol leren is de terugkoppeling naar het management en de medewerkers over de prestaties. Daartoe wordt gewerkt met een business case die als een besturingsmethodiek fungeert. Via periodieke managementrapportages worden de prestaties van medewerkers teruggekoppeld naar de oorspronkelijke doelstellingen/targets. Zo ontstaat een duidelijk beeld met een duidelijke fasering in de tijd van de wijze waarop de commerciële prestaties zich verhouden tot de gewenste doelstellingen.
De ‘leerbenadering’ heeft als voordeel dat management feeling houdt met de dynamische werkelijkheid. Door stap-voor-stap te werk te gaan en momenten van bezinning in te bouwen, is het call center voldoende flexibel om te kunnen inspelen op de meest recente ontwikkelingen. Het meest belangrijke is daarbij dat de tussenresultaten cumuleren. En dat de CRM implementatie niet beperkt blijft tot het eenmalige succes van een eerste pilot. CRM beklijft op zo’n manier en het call center ontwikkelt zich daadwerkelijk in de goede richting.
Trekker nodig om kloof te overbruggen
Als eenmaal duidelijk is welke kloof moet worden overbrugd, en hoe dit het beste kan worden gerealiseerd, is het zaak dat iemand het voortouw neemt. Iemand die voor de verschillende onderdelen de juiste richting aangeeft, en die het juiste tempo weet aan te geven. Ofwel, een trekker.
Globaal onderscheiden wij vier typen CRM-trekkers: Onrustige reiziger, Gezaghebbende gids, Vrolijke verkenner, en Winnende coach. Welke type het meest effectief is, hangt af van de situatie.
De Onrustige Reiziger wordt ingezet wanneer het call center geen duidelijke richting heeft waarin het zich wil ontwikkelen. En bovendien het call center ook geen enkele ambitie om met CRM te beginnen. Wil men toch met CRM aan de slag dan is ‘bewegen’ de beste optie. De trekker zal zich flexibel en zelfverzekerd moeten opstellen. Hij zal af en toe maar iets moeten proberen, en kijken of het aanslaat. Iteratief op zoek gaan naar iets dat mogelijk interessant is.
De Gezaghebbende Gids wordt ingezet wanneer het call center wel de ambitie heeft om met CRM aan de slag te gaan, maar het management heeft geen duidelijk doel voor ogen. Dit houdt in dat de Gids de aanwezige energie zoveel mogelijk moet kanaliseren, en misschien zelfs moet temporiseren in de richting van een mogelijk concurrentievoordeel. In deze situatie moet de trekker het call center een (toekomstig) probleem aanpraten, dat de richting aangeeft waarin wordt gewerkt. Dit betekent anticiperen op een toekomstige situatie door een gezaghebbende visie neer te leggen die medewerkers en management aanspreekt. De visie trekt de aanwezige energie aan die in eerste instantie kan worden gebruikt om alvast ‘te werken aan de toekomst’.
De Vrolijke Verkenner wordt ingezet als er wel een dreiging wordt gevoeld, maar het call center heeft nauwelijks ambitie om met CRM aan de slag te gaan. Medewerkers zijn niet of nauwelijks gemotiveerd. De timing is dan ook cruciaal; aanvoelen wat aanslaat, het kleinste vonkje energie laten groeien tot een volwaardig vuur. Om dit te bereiken, moet de trekker alvast maar op pad gaan. Zelf het voortouw nemen, en misschien (ongevraagd) zaken in gang zetten. Op verschillende plaatsen in het call center vragen neerleggen. Om de uitwerkingen/oplossingen onder te brengen in een samenhangend programma met een aantal what if scenario’s die laten zien wat CRM (voor de medewerkers en het call center) kan betekenen.
De Winnende Coach wordt ingezet in de situatie waarin het call center een een duidelijke richting heeft, gecombineerd met een sterke ambitie om met CRM aan de slag te gaan. De Winnende Coach is een trekker met autoriteit. Hij is in staat om management en medewerkers voor zich te winnen, voor hen een CRM-droom te schetsen en hen er vervolgens van te overtuigen dat ze deze droom kunnen waarmaken. In het ontwikkeltraject houdt hij op afstand vinger aan de pols en hij grijpt in alleen als het nodig is. Zaak is te doseren, en te anticiperen op toekomstige situaties bij het vinden van de juiste oplossing. Effectieve communicatie is belangrijk voor het bevorderen van de samenwerking en om management en medewerkers actief aan het denken te krijgen.
Door gebruik te maken van de wil binnen het bedrijf om met CRM aan de slag te gaan, en door de energie in de juiste richting te kanaliseren, kan een trekker het CRM ontwikkelingstraject sturing en/of tempo geven. Hiermee weet hij de kloof tussen de CRM-droom en de CRM-daad te overbruggen.
Conclusies
Na veel geld te hebben geïnvesteerd in allerlei mooie CRM pakketten, komen call centers nu met kritische vragen over de achterblijvende resultaten, en het geringe enthousiasme waarmee met het nieuwe systeem wordt gewerkt. Inmiddels is wel duidelijk dat de dagelijkse praktijk nog ver verwijderd is van de dromen die het management heeft bij CRM. De winst op klanten, en de relatie met klanten verbetert namelijk niet door alleen maar een CRM software pakket te installeren. Bovendien is er te weinig aandacht besteed aan het management en de medewerkers die moeten leren de beste klanten te werven, te onderhouden en te behouden.
In dit verhaal beschrijven wij hoe de belevingswereld van medewerkers en de doelstellingen van het management bij elkaar kunnen worden gebracht. De kloof moet stap-voor-stap worden overbrugd. Daarbij is het van belang dat medewerkers zich kunnen ontwikkelen en kunnen leren hoe zij de (potentieel) meest winstgevende en loyale klanten moeten werven, onderhouden en behouden. Daarnaast is het vinden van de juiste trekker een vereiste. De trekker kan afhankelijk van de situatie, het voortouw nemen en indien nodig gas geven en/of de richting bijstellen.
Duidelijk is wel dat medewerkers en management het vertrekpunt zijn in het CRM-ontwikkelingstraject voor het bij elkaar brengen van droom en daad. En niet, zoals zo vaak binnen het CRM-gedachtegoed de technologische mogelijkheden. De functionaliteit van CRM-software pakketten mag een sterke invloed hebben op de strategische opties van een call center, maar de houding en het gedrag van medewerkers bepalen het uiteindelijke succes. De CRM-software blijft tenslotte een hulpmiddel, en is geen doel op zich. De meerwaarde die CRM als bedrijfstrategie het call center kan opleveren, wordt dan ook voor een belangrijk deel bepaald door de mate en de snelheid waarmee de medewerkers in staat zijn om te leren hun (potentieel) beste klanten te werven, te onderhouden en te behouden. Het ontwikkelingstraject bepaalt de mate waarin alsnog de CRM-doelstellingen kunnen worden gerealiseerd. Ofwel, het dichten van de kloof tussen CRM-droom en CRM-daad.
Samenwerken in een customer contact center. Hoe haal ik het maximale resultaat?
Gert Haanstra, Call Center Magazine, F&G Publishing, Amersfoort, Nr. 9, 2001, blz 44 t/m 47
Printversie: Samenwerken in een customer contact center
Succesvolle bedrijven hebben het altijd al geweten: internet en call centers gaan hand in hand. Traditionele bedrijven met een multichannel strategie kunnen niet zonder een stevige internetpoot. En een dotcom-bedrijf dat nog wil overleven, heeft inmiddels een functioneel call center.
Samen met e-mail, voice response en mobiele telefonie brengen grote banken en verzekeraars hun internet- en callcenteractiviteiten onder in een customer contact center of customer care center. Het streven is gerichter met de klant te communiceren middels het effectief beïnvloeden van klantinteracties.
Effectieve communicatie staat niet gelijk aan het kopen van nieuwe technologie. Even belangrijk, zo niet belangrijker, zijn het op elkaar aan laten sluiten van de bedrijfsprocessen, en het laten samenwerken van de organisatie. We laten zien dat bedrijven in staat zouden moeten zijn om de verwachtingen van de klant – ten aanzien van het serviceniveau, de kwaliteit van de producten en de persoonlijke aandacht die hij krijgt – af te stemmen op hun eigen bedrijfsdoelstellingen. Zo moet men snel en goed kunnen inschatten wat de klant wil en hoe ze de klant kunnen bedienen. Ze staan klaar voor elke onverwachte ‘klant’ die langskomt, maar beoordelen alle klantinteracties – ongeacht kanaal, medewerker, tijdstip – direct op hun waarde voor het bedrijf. Bovendien kunnen bedrijven (via hun distributiekanalen en medewerkers) onmiddellijk en flexibel reageren op de klantvragen. Ze weten goed in te spelen op mogelijke verandering in de situatie van de klant, binnen de gegeven context, en gericht op het behalen van de eigen bedrijfsdoelstellingen. Uiteindelijk zullen klanten ervaren wat ze verwachten, en kunnen bedrijven hun aandacht richten op hun (potentieel) winstgevende en loyale klanten en deze eens goed en gepast bedienen.
Customer process re-engineering
Leidraad bij het inrichten van het bedrijfsproces is de doelstelling die men als bedrijf wil bereiken. Vervolgens gaat het over:
• Achterhalen wat klanten belangrijk vinden. Wat waarderen klanten het meest: product, service of klantkennis? Heeft een bepaalde activiteit van het bedrijf hoge impact? Hoe vaak krijgen bedrijven de kans om zich te bewijzen ten opzichte van de klant?
• Meten wat het bedrijf met het contact kan bereiken. Hoeveel draagt het bij aan de winst? Wat is de kans op succes? Kan het goedkoper? Kan het nog meer opleveren?
• Herkennen wat de klantwensen en veranderingen in het klantgedrag zijn. Wat zijn interessante events? Past dit in een klantprofiel? Wat is er in het recente verleden gebeurd? Kan ik het de klant zelf vragen?
• Afwegen wat de klant aan te bieden. Welke propositie? Via welk kanaal? En wanneer? Wat is een goed alternatief?
• Sturen op maximale klantwaarde. Hoe bied ik het aan? Wat is een geschikt moment in de interactie? Is het vrijblijvend of dwingend? En wat is het resultaat?
Structuur van de organisatie
Een klant komt binnen via een herkenbaar ‘loket’ (zelfbediening, gestandaardiseerde bediening of persoonlijke bediening). De medewerker achter dit loket voldoet aan de eisen om de klantvraag te kunnen beantwoorden en de commcerciële doelen te halen.
De eerste stap is de medewerkers indelen op basis van hun (product)kennis, hun (sociale en communicatieve) vaardigheden en hun (empathische) houding. Dit wordt gecombineerd met interesses en ervaringen van de medewerkers zelf. Medewerkers zijn in te delen in de volgende types:
• Een verdediger fungeert als back-up achter het ‘zelfbedieningsloket’, in het geval er iets mis mocht gaan. Hij is in staat om veelal schriftelijk te communiceren via e-mail, chat-internet, sms, etc. Vaak over technische onderwerpen als passwords, modems en firewalls. Daarnaast heeft hij de kennis en vaardigheden om een groot aantal problemen en vragen snel op te lossen. Het gaat hierbij om simpele, routinematige problemen van een oppervlakkig niveau.
• Een verdedigende middenvelder is in staat om de hoeveelheid service te beperken, ook al vragen klanten om meer. Klanten die continu informatie willen en vragen stellen, worden bewust ‘kort gehouden’. De medewerker van de ‘snelkassa’ is in staat om snelle en betrouwbare service te leveren. Ook hij heeft de kennis en vaardigheden om een groot aantal simpele, routinematige problemen van een oppervlakkig niveau op te lossen met gerichte vragen en goede samenvattingen.
• Een regisseur op het middenveld is in staat om in te schatten hoe winstgevend klanten en prospects kunnen zijn voor het bedrijf. Hij inventariseert daarvoor de wensen en behoeften, in veel gevallen door het stellen van relevante vragen. Een regisseur kent de weg in het bedrijf. En is vervolgens in staat om prospects en klanten duidelijk maar dwingend de richting op te sturen van het zelfbedieningsloket of de persoonlijke bediening.
• Een rechts- of linksbuiten is in staat om een klant binnen te houden. Een echte probleemoplosser met een open houding en een luisterend oor. Vaak meeverend in de argumentatie of de kritiek van de klant, altijd vanuit het contact met de klant. Deze medewerker van de ‘persoonlijke bediening’ communiceert helder en maakt concrete afspraken, intern én met de klant. Hij bedenkt een passende oplossing en draagt deze aan. Mocht het tot een confrontatie komen, dan weet hij een juiste inschatting te maken van het afbreukrisico of zal hij een alternatieve oplossing voorstellen.
• Een spits is degene die gaat voor het commerciële resultaat. Veelal krijgt hij verkoopkansen van anderen aangedragen. En dan is het zijn taak om het contract te sluiten. Als adviseur heeft hij overzicht en doseert hij zijn informatie. Hij geeft deskundig advies waar nodig en gewenst. De spits heeft mensenkennis. Hij gebruikt zijn vaardigheden om servicegesprekken om te buigen tot verkoopkansen. Met geduld en flexibiliteit pareert hij tegenargumenten en discussies van tegenspartelende klanten. Klanten die voor het bedrijf minder interessant zijn (maar wel behoefte hebben aan persoonlijke bediening) worden ‘netjes afgehouden’.
De juiste technologie
Hoe zet je de tools zodanig in dat ze de medewerkers voldoende ondersteunen en niet gaan tegenwerken? En niet onbelangrijk, hoe implementeer je de software in de bestaande IT-infrastructuur om te voorkomen dat alles overhoop wordt gehaald.
Globaal ondersteunt de technologie het bedrijf en haar medewerkers in een viertal activiteiten:
Het opvangen van alle klantcontacten.
Een bedrijf wil overal en altijd bereikbaar zijn, zodat een klant relatief eenvoudig en snel contact kan leggen met het bedrijf. Internet, maar ook moderne telefoontechnologie als interactive voice response (IVR), automatic call distribution (ACD) en computer telephone integration (CTI) zijn ontworpen om de klant overal en altijd toegang te geven tot het bedrijf. Klanten kunnen zelf informatie opvragen. Ze kunnen bestellingen opgeven en producten kopen, of wijzigingen aanbrengen in hun geregistreerde gegevens. Voor betalingen, authorisatie, spaar- en loyaltysystemen belt een klant in met smartcard en krijgt directe verbinding met de juiste afdeling of krijgt loyaltypunten. Eenmaal geïdentificeerd verbindt de onderliggende techniek de klant door via telefoon/modem met de betreffende systemen en databases. In het algemeen gebruiken bedrijven deze technologie om grote hoeveelheden klantcontacten snel, goed en relatief goedkoop af te handelen, zodat het bedrijf zijn productiviteit kan verhogen.
Prioriteren van klantcontacten.
De technologie kan worden gebruikt om selectief te werk te gaan in het afhandelen van klantcontacten. Een simpele oplossing is een klant niet automatisch bij het gekozen toestel te laten komen indien die niet bereikbaar is. Een stap verder is de call flow routering waarbij met een integratie van ACD en CTI inkomend (telefoon)verkeer binnen een afdeling of bedrijf is te sturen. Hiervoor moet expliciet de prioriteit worden aangegeven tussen de distributiekanalen én binnen een distributiekanaal. Beslissingen over routering kunnen worden genomen op basis van bijvoorbeeld het klanteigenaarschap (kantoren, het directe kanaal, de adviseurs hebben hun eigen klanten), het tijdstip of aantal beschikbare medewerkers. Als de klant eenmaal in een wachtrij zit, kan het bedrijf onderscheid maken in de benadering van deze klant. Het is mogelijk de klant voortdurend op de hoogte te houden van de veranderende wachttijd. Bovendien is het mogelijk actief de wachtrij te beïnvloeden. Klanten krijgen de keuze te willen wachten, te worden teruggebeld of het ‘probleem’ te willen oplossen via een ander kanaal (internet, voice response). Een bedrijf kan de wachtrij ook actief beïnvloeden door belangrijke klanten te bevoordelen en hun gesprek met voorrang toe te wijzen aan een agent.
Het is commercieel interessant om de prioriteit in het afhandelen van het klantcontact te laten afhangen van de winst die het bedrijf verwacht te gaan maken op basis van het klantcontact. Technologie als mobiele telefonie, internet, IVR, ACD en CTI zijn, in combinatie met aCRM-technologie, bij uitstek geschikt om kansen snel te signaleren en direct te benutten. Op het moment dat de klant zich bekend maakt, wordt uitgerekend wat het bedrijf aan hem kan verdienen, en hoe belangrijk hij is voor het bedrijf. De verschillende proposities worden doorgerekend en beoordeeld op kans van slagen en winstbijdrage. Vervolgens wordt de propositie met de hoogste winstbijdrage en kans van slagen aangeboden aan de klant.
Het klantcontact sturen op klantwaarde.
Het is mogelijk om de vraag van de klant soepel af te stemmen op de kwaliteiten van de medewerker. Als eenmaal bekend is welke propositie moet worden aangeboden, vindt de afstemming plaats met het meest geschikte distributiekanaal en de meest geschikte medewerker.
Bijvoorbeeld: het bedrijf rekent uit dat de familie Woutersen relatief veel gebruikmaakt van de geboden diensten, zonder dat daar inkomsten tegenover staan. Eigenlijk weet het bedrijf dat ze lang klant zullen blijven, maar niet echt winstgevend zullen worden. Dus op het moment dat meneer Woutersen belt, krijgt hij een lage prioriteit, en wordt doorgeschakeld naar een IVR of een verdedigende middenvelder die snel en pittig het contact kan afhandelen.
Een tweede voorbeeld is meneer Jager. Stel, u weet dat meneer Jager een klant is die in potentie geld kan gaan opleveren. Door middel van aCRM analyseert u dat hij, gezien zijn achtergrond, geïnteresseerd lijkt in de nieuwe internet service, die u hem bij een volgend klantcontact dan ook gaat aanbieden. Meneer Jager belt het call center op. Hij haalt echter vijfduizend euro van zijn rekening. De aCRM-technologie signaleert dit en beoordeelt meneer Jager als potentiële wegloper (i.p.v. de potentieel winstgevende klant). Het contactmoment van meneer Jager krijgt nu de hoogste prioriteit. Op basis van de laatste transactie, in combinatie met zijn achtergrond, komt de aCRM-software met een suggestie om meneer Jager een speciale aanbieding te doen met als doel hem te behouden als klant.
In dit geval wordt meneer Jager direct doorverbonden met een persoonlijke adviseur of een rechtsbuiten die in staat is een goed gesprek te voeren om hem als klant te behouden. De medewerker krijgt de suggestie om meneer Jager een incentive aan te bieden bij een product dat hem een jaar lang bindt aan het bedrijf. Meneer Jager gaat in op het aanbod en een belangrijke klant is voorlopig behouden.
Leren van eigen ervaringen.
Bedrijven moeten de reacties van klanten op voorgaande aanbiedingen meenemen in de propositie voor de volgende interactie. Zo ontstaat een communicatie over de diverse contactmomenten heen en bouwt zich een ‘gesprek’ op, dat in een volgende interactie wellicht een concreet resultaat oplevert. In ieder geval wordt de band tussen de klant en het bedrijf versterkt.
Klantinformatie kan worden doorgegeven van het eerste contact met een prospect tot het verzenden van de factuur of het leveren van het product. Dit is te realiseren met een sterke integratie tussen (a)CRM-systemen en ERP-systemen.
Daarnaast is er de bedrijfs interne tracking & tracing van de klant. Dit is te realiseren met een campagnemanagementsysteem of workflowsysteem, waarbij klanten voor de verschillende activiteiten naar de juiste afdelingen/medewerkers worden gestuurd. Ook hiervoor geldt dat de klant wordt herkend en via workflowfunctionaliteit wordt doorgestuurd, bijvoorbeeld op basis van het herkende telefoonnummer of een in IVR ingegeven contractnummer of het klantnummer op de webpagina.
De verschillende (marketing) activiteiten worden op elkaar afgestemd door contacten vast te leggen in een database, en alle medewerkers de informatie te laten gebruiken. Voor iedere medewerker is dan zichtbaar wie welke actie (eerder) heeft ondernomen.
De intelligentie om de verschillende systemen gericht aan te sturen, zit in de aCRM-software. Voordeel is dat er een consistente, effectieve communicatie ontstaat met de klant. Een tweede voordeel is dat in elk volgend contact duidelijker wordt op welke aanbiedingen een klant wel of niet zit te wachten. Een organisatie leert van deze reacties op klantcontacten en verbetert zich door de proposities aan te passen of de keuze van het distributiekanaal te veranderen. Met als doel het realiseren van de winstdoelstellingen.
Conclusie
Met de uitbreiding van het aantal distributiekanalen vragen bedrijven zich af hoe ze de verschillende distributiekanalen kunnen laten samenwerken om de geboden commerciële kansen optimaal te benutten. Het perfectioneren van de service werd het speerpunt: zoveel mogelijk klantcontacten snel en goed afhandelen. De klant heeft overal en altijd toegang tot zijn gegevens en het bedrijf. Hiertoe wordt de technologie uit de verschillende kanalen geïntegreerd. Met als doel het opbouwen van een volledig en consistent klantprofiel.
Uit dit artikel blijkt dat het gaat om het effectief managen van de klantcontacten over de verschillende kanalen heen, en niet om de technische integratie alleen. De klant wil het beste product, de beste service, of een bedrijf dat hem goed begrijpt. Een bedrijf staat klaar om op elke onverwachte ‘klantvraag’ (via zijn distributiekanalen en medewerkers) onmiddellijk en flexibel te reageren. Het weet snel en goed alle interessante kansen direct te signaleren en effectief te benutten door de klant perfect te bedienen, binnen de gegeven context. Mits goed ondersteund, kunnen medewerkers het contact effectief beïnvloeden en, bijvoorbeeld, meer verkopen. En vooral de (potentieel) winstgevende en loyale klanten goed en gepast verwennen. De service die wordt geboden is afgestemd op de potentiële winstgevendheid van de klant. Interessante kansen krijgen hoge prioriteit, worden geselecteerd, en verder benut met de juiste technologie en de juiste medewerkers. Op deze manier kan een bedrijf haar doelen bereiken, mits de bedrijfsprocessen, de organisatie en de onderliggende technologie op elkaar zijn afgestemd.
Samenwerken met een Marketing Analist
Een volgende stap in het beter laten renderen van uw Intelligence afdeling
Gert Haanstra, 2010; adviseur en interim manager op gebied van Intelligence en Klantwaarde Marketing vraagstukken
Printversie: Samenwerken met een Marketing Analist
De lente is eindelijk begonnen. De economie lijkt zich te herstellen. Misschien ook een goed moment om uw Intelligence afdeling eens tot volle bloei te laten komen? Onderzoek laat zien dat organisaties en managers hoge verwachtingen hebben van hun Intelligence afdelingen, maar dat die verwachtingen nog niet (volledig) zijn ingelost. Dezelfde onderzoeken laten ook zien dat er de afgelopen jaren flink is geïnvesteerd in Intelligence en dat de benodigde mensen en (ict) middelen op de meeste afdelingen wel aanwezig zijn. Dus is het nu een kwestie van profijt halen uit deze eerder gedane investeringen.
Verbetering is mogelijk door onder andere het opgeleverde analyse resultaat (=selectie, model, advies, etc.) beter te laten aansluiten bij wat een opdrachtgever/marketeer verwacht. En verbetering is ook mogelijk door een zodanig resultaat op te leveren dat een organisatie er ook daadwerkelijk mee aan de slag gaat. Dit lukt niet door nog meer van hetzelfde te gaan doen. Ofwel, niet nog meer verzoeken om lijstjes, en niet nog meer vage analyse opdrachten, en zeker niet meer ‘u vraagt, wij draaien’. Onze ervaring is dat deze directieve benadering het beste kan worden losgelaten. In plaats daarvan zou er sprake moeten zijn van een meer gelijkwaardige samenwerking gebaseerd op een besef dat Intelligence en Marketing/Verkoop/ Sservice wederzijds van elkaar afhankelijk zijn om te kunnen presteren en groeien. In dit artikel laten we zien dat met een paar stappen (terug) deze samenwerking tussen de Intelligence afdeling en haar directe (marketing/verkoop) omgeving eenvoudig kan worden verbeterd.
Een voorbeeld. Een marketeer vraagt aan een analist via de mail om een selectie voor de nieuwe campagne. De analist levert hem na twee dagen een lijst met klanten op. Dan realiseert de marketeer zich dat dit eigenlijk net niet het bestand is wat hij wil, en vraagt om een nieuw bestand. De analist is geprikkeld, omdat hij waarschijnlijk 2 dagen voor niets heeft zitten werken, en geeft aan dat hij nog ander werk te doen heeft. In de vriendelijke variant wordt er alsnog een excuus gemaakt en komt er snel een nieuw bestand. In de extreme variant escaleert dit, gaat er iemand via de bovenweg op zijn strepen staan, en wordt er met veel gesputter een nieuw bestand opgeleverd.
Weinig niet-analisten zullen volledig begrijpen van wat het betekent om analist te zijn. Zoals er waarschijnlijk ook weinig analisten zullen zijn die begrijpen wat hun opdrachtgevers meemaken. Is het dan vreemd dat de wederzijdse verwachtingen dan niet goed op elkaar aansluiten? De dagelijkse praktijk is dat de meeste opdrachtgevers, interne klanten en de marketing collega’s wel een verzoek doen om een specifieke selectie, bestand of telling, maar dat ze helemaal niet weten wat ze willen. Eigenlijk verwachten ze van een analist dat hij hen daarbij helpt. Opdrachtgevers en leidinggevenden geven dat ook aan als ze worden gevraagd naar de punten waarop een analist zich nog kan verbeteren.
Een ideale analist helpt bij het structureren van het probleem. Hij begrijpt wat een opdrachtgever bedoelt, en is in staat om zijn probleem te vertalen naar een passend analyse resultaat. En terecht. Dergelijke competenties horen bij een goede analist. Kortom, opdrachtgevers zien het liefst een analist die hen helpt, en hen verlost van een prangende vraag en/of een urgent probleem. Maar in plaats van om expliciet om hulp te vragen en open te staan voor suggesties van een analist, komen de meeste opdrachtgevers met een directief verzoek of een opdracht. Hulp vragen en opdrachten geven, dat is niet hetzelfde.
En de analist? Die denkt vooral; snel en efficiënt. Maar helaas denkt hij vaak niet mee met een opdrachtgever, maar hij denkt vooral voor een opdrachtgever. Dus in plaats van te luisteren, is hij al bezig met het oplossen van hoe de opdracht gaat uitvoeren. Gevolg is wel dat de eigenlijke vraag achter de klantvraag niet wordt gevonden. En dat braaf wordt opgeleverd hetgeen een opdrachtgever heeft gevraagd. En dat een opdrachtgever pas kritisch gaat nadenken als hij het resultaat opgeleverd krijgt… Hoe dan wel?
Fase 1: Loslaten & Opnieuw beginnen
Een logische eerste stap is om weer met elkaar in contact te komen. Dat kan door elkaar letterlijk weer op te zoeken. Dat kan door een collega te vragen hierin te bemiddelen. Dat kan ook door externe begeleiding in te schakelen. In ieder geval moet iemand het voortouw nemen, zijn bezwaren/schroom overboord gooien en als eerste de stap zetten om weer tot elkaar te komen.
Vervolgens is het de bedoeling om de lucht te klaren. Dat betekent de tijd nemen en met elkaar in gesprek gaan. Welgemeende aandacht geven, door te luisteren en te vragen naar hoe de ander het contact en de onderlinge communicatie ervaart. Maar ook door zelf alle gevoeligheden uit te spreken. En door duidelijk te maken hoe een goede samenwerking er uit zou moeten zien.
Tot slot, is het zaak om nieuwe en betere afspraken te maken. Beide partijen hebben elkaar gevonden en de verhoudingen zijn weer redelijk normaal, dan ligt het voor de hand om concrete afspraken te maken over hoe de samenwerking in de toekomst zou moeten verlopen. Wat erg goed helpt is het gebruiken van een opdrachtbrief. In een opdrachtbrief staat bijvoorbeeld hoe het (intake)proces gaat verlopen; wie wat gaat doen, en welke zaken wanneer worden opgeleverd? Vaak wordt er achtergrond informatie opgenomen over het eigenlijke probleem, de gekozen oplossingsrichting, de methodiek/aanpak, de eisen aan het analyseproduct, en wat een opdrachtgever ermee wil gaan doen. Voor zware, riskante opdrachten kan een opdrachtbrief uit meerdere A4tjes bestaan. Voor de meer eenvoudige (herhalings)opdrachten is vaak een e-mailtje met een heldere terugkoppeling voldoende.
Een voorbeeld. De opdrachtgever geeft dat hij de analist star en inflexibel vindt, omdat deze erg moeilijk doet als de opdrachtgever op het laatste moment nog iets wil wijzigen in de selectie of analyse. Hij heeft het gevoel dat analist niet meedenkt en alles afhoudt om het zichzelf makkelijk te maken. De analist vindt dat de opdrachtgever te weinig kennis van zaken heeft en vaak geen idee heeft van wat hij nu werkelijk wil hebben. Daarnaast totaal geen zicht en begrip heeft voor het grote hoeveelheid werk dat er voor verricht moet worden.
Na elkaar de waarheid te hebben gezegd, wordt afgesproken dat circa 80% van de bestaande, terugkerende aanvragen gaan verlopen een vaste, eenmaal afgesproken procedure. En dat in de overige 20% meer ruimte is voor interactie en tussentijdse aanpassingen.
Fase 2: Uitproberen & Discipline handhaven
Eenmaal opnieuw begonnen is het zaak om de samenwerking verder vorm te geven. Daarbij is het belangrijk om vast te houden aan de gemaakte afspraken. Dit betekent dat iedereen zijn bijdrage levert om elke keer weer tot een goede opdrachtbrief te komen. Het betekent ook dat de afspraken zoals die in de opdrachtbrief worden vastgelegd, worden nagekomen. Analisten moeten bijvoorbeeld niet ongevraagd andere analyseproducten gaan opleveren dan afgesproken. En opdrachtgevers moeten bijvoorbeeld niet andere producten gaan vragen dan is afgesproken. Discipline in het maken en nakomen van afspraken is dan ook de leidraad.
Daarnaast zal de analist met externe hulp moeten leren niet direct te beginnen met het uitwerken van een klantvraag/verzoek. Maar te luisteren naar wat een opdrachtgever nu werkelijk bedoelt. Hij zal moeten leren door te vragen, en de verkregen informatie kritisch te beoordelen. Om daarna te proberen het probleem te ordenen, de eigen indrukken te spiegelen en de bevindingen door de opdrachtgever te laten bevestigen. Om daarna de opdrachtgever te adviseren met effectieve oplossingen. Na een tijd zo te hebben gewerkt is het goed om stil te staan bij vragen als: Hoe heeft zich de samenwerking ontwikkeld? Is iedereen nog blij met zijn nieuwe rol en taakverdeling? Bedoeling is om onder begeleiding de samenwerking samen verder te laten groeien. Dat betekent die zaken waarderen die goed gaan, en verbeteringen doorvoeren op de punten die beter kunnen. Evalueren en het uitspreken van de eigen ervaringen is daarbij belangrijk.
Een voorbeeld. De opdrachtgever geeft bij de analist aan dat hij nu even geen tijd heeft voor het (mede)opstellen van de opdrachtbrief, en dat de analist de opdrachtbrief van de vorige keer maar moet gebruiken. De analist toont begrip voor de drukke situatie van de opdrachtgever, herinnert hem nog even aan de gemaakte afspraken, en herhaalt nog een keer de voordelen van het maken van een gezamenlijke opdrachtbrief. Als oplossing stuurt de analist een opdrachtbrief waarin de ‘zekerheidjes’ al zijn ingevuld door ze van de vorige opdrachtbrief over te nemen. Maar waar nog een aantal cruciale vragen openstaan die door de opdrachtgever nog wel op tijd beantwoord moeten worden.
Fase 3: Waarderen & Ongevraagd helpen
Uiteindelijk ontstaat er een situatie waarbij de samenwerking tussen opdrachtgever en analist als het ware behoorlijk ingesleten raakt, en waarbij beide partijen beseffen dat ze elkaar nodig hebben om het beste resultaat te bereiken. Daaraan voorafgaat nog een geleidelijk proces waarin opdrachtgever en analist elkaar steeds makkelijker weten te vinden. Ze hebben elkaars sterke punten leren waarderen, en hebben oog en begrip gekregen voor elkaars minder sterke punten. Men ziet en erkent de inbreng van de ander, en men heeft geleerd om de waardering voor elkaar ook uit te spreken. Er is voldoende vertrouwen om te kunnen leunen op de ander. En er is het besef dat er sprake is van een wederzijdse afhankelijkheid.
Uiteindelijk zal de samenwerking steeds prettiger gaan verlopen. En ontstaat er een situatie waarbij iedereen elkaar helpt en ongevraagd adviseert om iemand verder te helpen. Ook de onderlinge verhoudingen verschuiven; een opdrachtgever – opdrachtnemer situatie maakt plaats voor een situatie waarin de analist zich opstelt als ‘trusted advisor’. Hierbij treedt de analist veel meer pro-actief en regisserend op om het gezamenlijk belang van een effectievere en rendabele marketing beter, sneller, makkelijker te realiseren.
Kortom, met behulp van bovenstaande stappen is het mogelijk om de samenwerking tussen analist en opdrachtgever, en tussen de Intelligence afdeling en haar directe (marketing/ verkoop) omgeving, sterk te verbeteren. In een aantal eenvoudige stappen lukt het om een vastgelopen situatie weer los te krijgen, nieuwe afspraken te maken en zich daaraan te houden omwille van het gezamenlijk belang. Eenmaal in beweging ontwikkelt de samenwerking zich verder en lukt het steeds beter om elkaar te helpen. Waarbij beide partijen leren beseffen en leren waarderen dat een goede samenwerking leidt tot de beste resultaten voor alle betrokkenen.
Geld verdienen met uw contact center (boek)
Auteur: G. Haanstra
Co-auteur: E. Vierbergen
Taal: Nederlands
Afmetingen: 8x211x149 mm
Gewicht: 190,00 gram
Druk: 1
ISBN10: 9075432534
ISBN13: 9789075432534
De winst van analytics
Artikelen over de (financiële) voordelen van het gebruik van analyse en voorspelmodellen.
Van Share Holder Value naar Customer Value
Gert Haanstra en Reinier Schut, Banking Review, Tijdschrift voor financiële professionals, Weteringgroep Uitgevers BV, Amsterdam, Nr. 7, 2000
Printversie: Van Share Holder Value naar Customer Value
Een financiële instelling wordt gewaardeerd om haar winstgevendheid. Maar in de dagelijkse praktijk worden commerciële onderdelen niet vaak rechtstreeks aangestuurd op het maken van winst. Wel op omzet of kosten. De vertaling van winstdoelstellingen in concrete activiteiten richting de klant wordt als lastig ervaren. Tot nu toe.
Kostenbesparing is eindig
Financiële instellingen hebben hun winstgevendheid vergroot door voornamelijk kostenbeheersing: automatiseren van processen, sluiten van kantoren en meer gebruik maken van efficiëntere kanalen als call center en electronic banking. Deze aanpak van kostenbeheersing is reeds lange tijd ingezet en lijkt eindig, dat wil zeggen dat er nauwelijks meer financieel voordeel lijkt te worden behaald. Maar er zijn mogelijkheden voor financiële instellingen om direct te sturen op de individuele klant en daarmee de winstgevendheid van de klant en het bedrijf te vergroten.
Voorwaarden
De achtergronden en mogelijkheden van Customer Relationship Management worden regelmatig besproken in Banking Review. Financiële instellingen hebben veel gegevens over hun klanten. Deze gegevens bieden volop mogelijkheden om meer inzicht te krijgen in het klantenbestand. Een voorbeeld daarvan is een verfijnde segmentatie van het bestand op basis van de huidige klantwaarde, maar ook van de potentiële klantwaarde.
Daarnaast hebben de gegevens voorspellende waarde. Zij zijn immers een reflectie van het gedrag van de klant. Een reflectie die met behulp van gedegen analyses is te gebruiken voor het verkopen van (nieuwe) producten. De voorspellende kracht kan worden benut om klantgerichte aanbiedingen te doen. Zaak is de data-analisten de resultaten van hun analyses interpreteerbaar te laten maken voor marketeers en managers en de resultaten vervolgens direct te kunnen toepassen in een – al of niet gepland – contact met de klant. Een voorbeeld van een uitgevoerde analyse is een klantprofiel waarmee de meest kansrijke klanten voor een koopsompolis worden geselecteerd of een concrete lijst van n.a.w.-gegevens van klanten met een verwachte hoge respons op een groenfonds.
Om de winstgevendheid te vergroten door directe sturing op de individuele klant, moet aan een aantal voorwaarden worden voldaan:
• Elk interessant contact met de klant (via elk kanaal en op elk tijdstip) moet worden gesignaleerd en benut. Kortom, een multi-channel aanpak. Immers, de huidige klant zoekt contact met een financiële instelling, vanaf elke plaats die hij kiest, en wanneer hij dat wil. Het is van het grootste belang de aandacht van de klant vast te houden door de boodschap of het advies af te stemmen op zijn persoonlijke wensen en behoeften.
• In plaats van product- of campaigngeoriënteerde managers met omzet targets op product niveau zijn klantgroep- of segmentgeoriënteerde managers noodzakelijk, met als doel de customer value van deze klantgroep te vergroten.
Vertalen kennis in activiteit
Zelfs als aan deze twee voorwaarden is voldaan, is hiermee nog geen concrete invulling gegeven aan ‘het sturen op de winstgevendheid van de klant’. Vaak ontbreekt de vertaling van kennis over klanten met een grotere kans op winstgevendheid naar concrete acties naar die klant toe. Cruciaal punt is dan ook hoe financiële instellingen in de dagelijkse sales en marketing wel concreet hun klanten individueel kunnen aansturen en daarmee hun winstbijdrage voor het bedrijf kunnen verbeteren. In de praktijk kan dit door:
• De meest winstgevende klanten te benaderen. Op het moment dat een marketeer (budgetverantwoordelijke) een beslissing neemt over het opzetten van een volgende actie, is direct inzicht nodig over o.a. de verwachte kosten van de actie, de verwachte opbrengsten, maar ook de verwachte winstgevendheid van de klant en de totale winstbijdrage van deze actie. Deze verwachte resultaten kunnen worden berekend op basis van onderliggende voorspellingsmodellen.
• De meest winstgevende producten aan te bieden. Daarvoor is het nodig vooraf het een en ander uit te rekenen en duidelijke prioriteit te geven aan de verkoop van deze producten. Dan kan op het moment dat er contact is met de klant juist dit meest winstgevende product worden aangeboden ‘ten koste’ van een ander product. Een dergelijke prioriteit moet gelden voor alle kanalen, zodat via welk kanaal het contact ook verloopt, de klant overal hetzelfde persoonlijke aanbod krijgt. Daarbij moet wel rekening worden gehouden met bijvoorbeeld de regel dat er maar één keer per maand een aanbod wordt gedaan.
• De inbound contacten in het call center pro-actief te benutten. Te vaak wordt het call center als service kanaal gezien waarbij het afhandelen van de vraag van de klant voorop staat. Met de juiste aCRM technieken is het mogelijk om het service gesprek om te zetten in een verkoopgelegenheid. Zo’n verkoopgelegenheid levert geld op, waarbij de tijd die nodig is voor het verkoopgesprek wordt doorberekend aan de marketeer om vervolgens te worden geïnvesteerd in extra call center mensen. Ofwel, de service blijft op peil, en het kanaal levert een winstbijdrage dankzij het genereren van omzet.
Winstgevende 1-op-1 relaties
De geschetste mogelijkheden laten zien dat de kloof tussen share holder value en customer value kan worden overbrugd. Daarmee wordt een belangrijk deel van de belofte ingelost om een winstgevende 1-op-1 relatie met de klant te kunnen onderhouden. Bovendien laten de voorbeelden zien dat de directe sturing op klantniveau waardevolle kansen biedt en zeer winstgevend kan zijn.
Voorbeeld 1: Outbound mailing naar meest winstgevende klanten
Stel, per jaar verstuurt een bank voor een specifieke spaarverzekering 150.000 mailingen aan haar klanten. De gemiddelde conversie is 1,5%. Waarbij de gemiddelde verwachte winst 3.000 gulden (over 10 jaar) per contract is. In totaal worden 2.250 polissen verkocht. De totale winstbijdrage bedraagt 6,8 miljoen gulden.
Door een model te maken waarmee de meest kansrijke en meest winstgevende klanten worden geselecteerd, zal de totale winstbijdrage stijgen. Bijvoorbeeld, bij een conversie van 2,5% op 100.000 mailingen, zullen 2.500 contracten worden verkocht. De winstverwachting ligt hoger, namelijk op 3.500 gulden. De totale winstbijdrage is daarmee 8,8 miljoen gulden. Een extra winst van 2 miljoen gulden (over een periode van 10 jaar).
Voorbeeld 2: Inbound benutten van verkoopkansen in het call center
Stel, op het call center worden a-CRM technieken gebruikt waarmee de meest kansrijke contacten worden gesignaleerd, en waarmee vervolgens de agent een duidelijke suggestie krijgt (welk product hij kan aanbieden en waarom). Dan geldt:
• 100.000 inbound contacten per maand.
• 5.000 contacten zijn potentieel interessant voor verkoop (=5%)
• 500 contracten gesloten (=10% van 5.000)
• 3.500 gulden winstmarge (over 20 jaar)
• totale winstbijdrage: 1,8 mln per maand (=500 contracten * 3.500 gulden)
Analytische Customer Relationship Management
Analytische Customer Relationship Management technieken zijn een apart onderdeel van het totale CRM-concept. De a-CRM is erop gericht de relatie met de klant te versterken en de winstgevendheid (customer value) te vergroten door op basis van analyse van individuele klantgegevens, proposities te genereren, deze in het directe contact met de klant aan te bieden en het resultaat van de acties te evalueren.
Hoe u geld kunt verdienen aan uw internet-klanten
Gert Haanstra, MEC, Marketing Executive Center, Den Haag, Nr.8, juli 2001, blz 28 t/m 30
Printversie: Hoe u geld kunt verdienen aan uw e-klanten
Uw klanten worden steeds veeleisender, veranderen continue hun voorkeuren en willen vooral zelf bepalen wanneer, waar en hoe zij uw producten of diensten kopen. Internet is bij uitstek het kanaal waarbij de klant ‘in control’ is. Hoe kunt u hierop inspelen? En ervoor zorgen dat dit bijdraagt aan de winstgevendheid van de relatie? Analytische CRM, of kortweg aCRM, maakt het mogelijk ieder klantcontact te personaliseren en in kaart te brengen wie uw meest winstgevende klanten zijn. Door aCRM te integreren met uw website en andere kanalen wordt het mogelijk snel te reageren op de grillige behoeften van uw klanten, op ieder moment van de dag.
Geld verdienen aan uw website
Een ‘flashy’ site met aantrekkelijke aanbiedingen is één manier om klanten naar uw site te trekken. En natuurlijk speelt een gebruiksvriendelijke navigatie ook een belangrijke rol. De ‘gevorderden’ onder u houden zich misschien al bezig met affiliate marketing. Of analyseren de effectiviteit van banners, pagina’s en content of brengen ‘click’-stromen van klantgedrag in kaart. Maar is dit voldoende? Uw e-business activiteiten helpen u wel om uw prospects te interesseren, hen naar uw site te krijgen en de gewenste informatie te geven. Maar helpen zij u ook om de juiste (meest winstgevende en de meest loyale) klant te vinden? Analytische CRM (aCRM) software helpt u om uw internet applicaties te vullen met intelligentere selectiecriteria om te bepalen welk aanbod geschikt is voor een specifieke klant.
Analytische CRM software analyseert uw klantendata en presenteert de resultaten in inzichtelijke klantprofielen. Met aCRM kunt u achterhalen wie u als (potentieel winstgevende) klant moet binnenhalen. Uw marketingacties zullen dus veel gerichter zijn. Meer effect en minder waste dus. Het gebruik van aCRM levert u de juiste klanten op en verlaagt de kosten van marketing, sales en serviceverlening. De software identificeert welke klanten een verhoogde interesse zullen hebben voor een bepaald aanbod, waardoor u minder klanten hoeft te benaderen voor hetzelfde –of zelfs een beter- resultaat. Door aCRM in te zetten binnen één kanaal kunt u al winst boeken. Maar het maximale resultaat behaalt u door alle kanalen met elkaar te integreren. Op deze manier kunt u uw klanten optimaal bedienen waardoor de klantloyaliteit wordt verhoogd.
Integratie met traditionele kanalen
Integratie met de bestaande distributiekanalen is noodzakelijk voor een effectieve marketingstrategie. Interessante (verkoop)kansen en ernstige bedreigingen kunnen hierdoor op ieder moment en via ieder kanaal worden gesignaleerd zodat geen enkele kans verloren gaat. Of de klant u belt of uw website bezoekt, met behulp van analytische CRM kunt u de klant onmiddellijk een gepersonaliseerd aanbod doen.
U kunt alleen een goed beeld vormen van uw klant als alle inkomende en uitgaande gegevens over uw klant goed bijeen worden gebracht, worden geïntegreerd. Dan kunt u uw activiteiten laten aansluiten op elkaar, zonder te kannibaliseren tussen de kanalen, dubbele aanbiedingen te doen of aanbiedingen die niet gewenst zijn, en voorkomen dat de klant u gaat verlaten. Maar dat werkt alleen als alle data op een geïntegreerde manier worden geconsolideerd, geanalyseerd en gedistribueerd. Een goede aCRM-oplossing is gebaseerd op een open architectuur waardoor zij gemakkelijk met de verschillende front-end systemen zoals call center en internet, en bestaande back-end systemen is te integreren.
Integratie zorgt er ook voor dat medewerkers via alle kanalen op de hoogte zijn van wat er in andere kanalen is gebeurd met een klant. Een klant heeft actief naar hypotheekinformatie gezocht op uw website. Eén van uw marketeers heeft hem diezelfde dag een folder gestuurd over autoverzekeringen en dat doorgegeven aan een medewerker van het call center. Nu belt die klant om zijn saldo op te vragen. De call center medewerker vraagt hem naar de autoverzekering. Uw klant hoeft geen autoverzekering en zegt dat ook. De kans om terug te komen op de hypotheek waar de klant naar op zoek was, wordt echter niet benut, want zijn internet-gedrag was niet bekend bij de call center medewerker. Als niet alle contactpunten met uw klant een consistent en volledig beeld hebben van uw klant mist u dus kansen.
Personaliseren van uw aanbod
Verkoop genereer je door de klant die de site bezoekt, een aanbieding te doen. Cruciaal is het doen van een juiste aanbieding, dat wil zeggen een aanbieding ‘waar de bezoeker op zit te wachten’. Daarvoor moet op moment dat een klant de site bezoekt, in de database worden gekeken of er wel een aanbod voor deze klant is. En zo ja, welk aanbod interessant is voor deze klant. Op de achtergrond worden, specifiek voor deze klant, allerlei scoremodellen uitgerekend. Vervolgens wordt het meest kansrijke aanbod geselecteerd en klaargezet om te worden gepresenteerd in een pagina van de site. Dit noemen we ook wel real-time recommendations. Deze recommendations kunnen worden verwerkt in een banner, grafiek, pagina, etc. Dit alles gebeurt op het moment dat de klant aanwezig is op de site.
Hoe persoonlijker de aanbieding richting de klant, hoe effectiever de beïnvloeding. Zeker voor het web geldt dat er weinig gelegenheid is om de klant te sturen. Immers, er is veel afleiding en er zijn voldoende alternatieven. Een bezoeker zal in korte tijd oppervlakkig waarnemen of de pagina interessant is om te bekijken en verder te lezen. Vervolgens zal hij weinig moeite willen doen om de informatie volledig te begrijpen. Het betekent voortdurend een gevecht om de aandacht van de bezoeker vast te houden. Door de aanbieding persoonlijker te maken, lukt het om aandacht van de bezoeker langer vast te houden, en hem in een bepaalde richting te sturen.
Een persoonlijke benadering houdt ook in dat de klant kan kiezen met welk distributiekanaal hij zaken wil doen. Het is mogelijk dat de klant via het web nog te weinig uitleg kan krijgen op zijn vragen, of onvoldoende mogelijkheden heeft om zijn persoonlijke voorkeuren duidelijk te maken. In dat geval zou hij moeten kunnen ‘uitwijken’ naar bijvoorbeeld een call center. Veel grote dotcom bedrijven hebben naast hun sites ook een uitgebreid call center om hun klanten deze mogelijkheid te geven. Bijkomend voordeel is dat klanten die via meerdere kanalen aankopen doen gemiddeld aanzienlijk meer besteden.
Snelheid
Waar het om gaat is de klant en zijn voorkeuren als uitgangspunt te nemen en dit als basis te nemen voor de keuze van het product en het moment van aanbieden. Het moment waarop het aanbod gedaan wordt is hierbij misschien nog wel belangrijker dan het aanbod zelf. We kunnen iemand bijvoorbeeld een mooi aanbod doen voor een nieuw beleggingsfonds maar als de klant zijn geld net voor een langere periode heeft vastgezet in een koopsompolis zal de kans op succes erg klein zijn.
Snelheid is daarom een bijna allesbepalende factor voor het slagen van uw e-business aanpak. Kansen en bedreigingen moeten direct als zodanig worden herkend zodat u geen gelegenheid verliest om de kans te benutten of de bedreiging te counteren. Bijvoorbeeld; een belangrijke klant informeert naar de waarde van zijn huidige verzekering, of naar de mogelijkheden zijn verzekering te veranderen. Misschien heeft hij veel klachten of vragen of zelfs een betalingsachterstand. Dit zijn allemaal mogelijke aanwijzingen dat hij overweegt een product dat u kunt leveren bij een andere aanbieder zal gaan afnemen. Alleen als uw infrastructuur dit signaleert kunt u binnen seconden reageren en voorkomen dat u die klant verliest.
De beste combinatie
Internet biedt naast de traditionele kanalen zoals call centers en persoonlijke service een uitgelezen kans om veel kennis over klanten op te bouwen. Bovendien leveren deze directe contacten ook nog eens een goede en zeer goedkope mogelijkheid om de klantwaarde te verhogen. Ieder contact geeft een organisatie de gelegenheid de klant een propositie op maat te doen, die concreet bijdraagt aan de relatie met die individuele klant. Het is de uitdaging de beste combinatie van de verschillende kanalen te vinden: uitgebreide service bieden via bijvoorbeeld persoonlijk contact, of goedkoop en snel werken en reageren via bijvoorbeeld de internetsite.
Met behulp van aCRM kunt u zelfs de distributiekanalen die u gebruikt analyseren op effectiviteit en efficiëntie. U kunt achterhalen welk distributiekanaal het meeste effect zal opleveren, rekening houdend met de kosten van het kanaal en de belasting die het kan verdragen. Misschien blijkt wel dat bijvoorbeeld gebruikers van prepaid kaarten een grotere voorkeur hebben voor het kopen via internet dan via het postkantoor. Dan kunt u daar uw acties op richten. En zult u de kanaalkosten zien dalen.
Verdien uw investering terug
De hype rond e-business is voorbij, en toepassingen moeten weer winstgevend zijn. aCRM is geschikt om uw e-business strategie te verbeteren door het signaleren en benutten van kansen en bedreigingen bijvoorbeeld op het web. Het neerzetten en uitvoeren van allerlei internet activiteiten is vaak al een flinke investering (in mensen en machines). Maar pas een investering in een goede aCRM implementatie levert u op korte termijn een flinke winst op.
Het succes van aCRM binnen een internet omgeving wordt voor een belangrijk deel bepaald door een volledige integratie met traditionele distributiekanalen, en door de klant op een intelligente en persoonlijke manier aan te spreken, rekening houdend met zijn persoonlijke voorkeuren en individuele historie. Vandaar dat aCRM waardevolle kansen biedt en zeer winstgevend is gebleken. En u daarmee in staat stelt uw investeringen (en zelfs gedane CRM investeringen) snel terug te verdienen.
Mobiel bankieren, een commercieel succes?
Gert Haanstra, Banking Review, Tijdschrift voor financiële professionals, Weteringgroep Uitgevers BV, Amsterdam, November 2001, blz 17, 19 en 20
Printversie: Mobiel bankieren
Mobiel bankieren is hot. Er wordt regelmatig over gepraat, veel over geschreven, en aanbieders beloven gouden bergen. Ook de consument zelf heeft er overduidelijk interesse in. Want nog meer dan bij internet, geeft mobiel bankieren de klant de mogelijkheid om op elke plaats en op elk gewenst moment (bank)zaken snel en makkelijk te doen.
Banken zien dit ook en hebben hun eerste initiatieven op dit gebied inmiddels ontplooid. Hierbij springt de actie van Postbank en Telfort om vijfhonderdduizend pre-paid telefoons cadeau te doen nog het meest in het oog. Maar hebben deze aanbieders zich wel afgevraagd hoe het geld wordt (terug)verdiend? Doordat klanten steeds vaker zelf het initiatief nemen, lijken ze ongrijpbaar te worden voor banken en verzekeraars. Het wordt moeilijker om producten op de traditionele manier te verkopen. Analytische Customer Relationshop Management (aCRM) software biedt hier uitkomst. In dit artikel laten we zien hoe banken en verzekeraars in contact kunnen blijven met de klant, door mobiel bankieren te combineren met aCRM-technologie. En wat de (technische) voorwaarden zijn om mobiel bankieren zo tot een (financieel) succes te maken.
Mogelijkheden
De mogelijkheden van mobiel bankieren zijn bekend. Gebruikers kunnen met hun mobiele telefoon afrekenen, overal en op elk moment van de dag. Of het nu in de supermarkt is of op het terras. ‘s Ochtends vroeg of midden in de nacht. Met hun elektronische portemonnee willen klanten ook andere financiële zaken kunnen regelen, zoals overschrijven en het betalen van rekeningen. De mobiele telefoon of Personal Digital Assistent (PDA) is tevens geschikt voor beleggen, het plaatsen van beursorders en het beheren van de effectenportefeuille. En uiteraard, voor het makkelijk opvragen van saldo-informatie en de waarde van de effecten.
Voor banken is het mobiel bankieren interessant omdat het een mogelijke kostenbesparing betekent. Met name op het gebied van billing. Het op elektronische wijze aanbieden en betalen van facturen (al dan niet in een geconsolideerde vorm) is voor de klant gemakkelijk en aantrekkelijk. En deze selfservice door klanten is veel goedkoper dan de arbeidsintensieve data entry via een callcenter of backoffice. Evenals bij internet is ook hier de belangrijkste businessdrive ‘efficiency’. Het herinrichten van een bedrijfsproces om hetzelfde werk te kunnen doen in minder tijd, en met minder geld en mensen. En ook hierbij is het maar de vraag of het zijn belofte gaat inlossen en geld gaat opleveren.
Nieuwe uitdagingen
Pas als bedrijven leren effectief met hun klanten te communiceren, zullen ze een grote stap voorwaarts maken en wordt het implementeren van mobiel bankieren een overdonderend commercieel succes. Op dit moment communiceert de marketeer onvoldoende met zijn klanten. Hij luistert niet meer en is het contact inmiddels kwijtgeraakt. Wat je ziet is dat er gescheiden werelden zijn ontstaan, waarin marketeer en klant langs elkaar heen leven. De marketeer zit achter zijn computer en denkt de klant te kennen. Terwijl de klant het gevoel heeft dat het bedrijf hem niet begrijpt. De marketeer bij een grote, succesvolle bank of verzekeringsmaatschappij bouwt een database of datawarehouse en huurt een aantal analisten in om doelgroepen te achterhalen. Vervolgens bestookt de marketeer deze doelgroepen met grote hoeveelheden mailings, huis-aan-huis brochures en telefoontjes om iedereen een keer te hebben bereikt. De klant wil echter doelgericht met het bedrijf kunnen praten, al dan niet via de mobiele telefoon. Hij zoekt contact met het bedrijf en verwacht een passende reactie van het bedrijf. Wil het bedrijf hem iets duidelijk maken, dan staat hij daar open voor en neemt de tijd. Maar het moet wel relevant zijn en passen bij zijn persoonlijke situatie. Geen generieke boodschappen, maar to-the-point en met voor hem interessante aanbiedingen. Voor de marketeer betekent dit dat hij los moet komen van zijn ‘ivoren toren’, en zich moet openstellen voor wat de klant wil. Zijn taak is om snel in te schatten of het bedrijf kan inspelen op de wensen van de klant en hier voordeel mee kan halen. Om vervolgens interessante aanbiedingen te doen richting de klant en zo kansen te creëren voor het bedrijf. Pas dan gaat mobiel bankieren echt iets opleveren, zowel voor het bedrijf als voor de klant.
Hoe gaat het in zijn werk?
Gericht en effectief met elkaar communiceren betekent dat de medewerker de klant moet kennen. Bijvoorbeeld op het moment dat een klant via zijn mobiele telefoon een overboeking wil regelen. De medewerker luistert naar wat de klant wil en reageert in zijn eigen communicatie op het gedrag van de klant. Vervolgens wordt op de achtergrond uitgezocht of er nog interessante proposities voor deze klant zijn. ACRM-software berekent de aanwezige proposities en prioriteert deze op basis van de verwachte winst. De propositie met de hoogste verwachte winst wordt als suggestie verstuurd en gepresenteerd aan de medewerker, of rechtstreeks aan de klant.
Marketeers krijgen de kans om via mobiel bankieren hun commerciële doelen te bereiken; door het effectief beïnvloeden van klantinteracties. Alle interacties (ongeacht distributiekanaal, medewerker of tijdstip) worden beoordeeld op hun waarde voor het bedrijf. Op het moment dat een interessante kans zich voordoet (in de database, vanuit de kanalen, et cetera) is hij in staat om (via het scherm van de mobiele telefoon of de eigen medewerkers) onmiddellijk en flexibel te reageren. Met de gerichte suggestie wordt goed ingespeeld op een mogelijke verandering in de situatie van de klant, binnen de gegeven context.
Wat is er voor nodig om te slagen?
Misschien met uitzondering van het gebruikersgemak (lange opstarttijd en teveel handelingen) voor mobiel bankieren, is de techniek er helemaal klaar voor om van mobiel bankieren een commercieel succes te maken. In ieder geval de aCRM-technologie. Wel worden drie duidelijke eisen gesteld om de klantcontacten via de mobiele telefoon effectief te kunnen beïnvloeden:
• exact in kunnen schatten wat de klant wil en met welke propositie men de klant kan verleiden,
• snel reageren op het gedrag van de klant en snel kunnen uitrekenen wat de meest winstgevende propositie is voor het bedrijf,
• met een actueel aanbod dat rekening houdt met de meest recente veranderingen in de situatie van de klant.
Met de nieuwe generatie aCRM-software weten marketeers te allen tijde wie hun beste klanten zijn en wat ze deze klanten moeten aanbieden om hen tevreden te houden. Klantgegevens worden stevig onderzocht op (commercieel) interessante klantprofielen. Met als resultaat voorspellingsmodellen die de (verwachte) winstgevendheid en (verwachte) loyaliteit van klanten voorspellen.
Voorbeelden
Een voorbeeld is meneer Jager die regelmatig het callcenter belt voor informatie over zijn huidige saldo. U weet dat meneer Jager een klant is die u in potentie geld kan gaan opleveren. Door middel van aCRM analyseert u dat hij, gezien zijn achtergrond, waarschijnlijk geïnteresseerd is in de nieuwe informatieservice. Deze service voor de mobiele telefoon biedt u hem aan. En mocht meneer Jager de service kopen, dan gaat u meer aan hem verdienen dan dat hij u op dit moment kost.
Een tweede voorbeeld is de familie Woutersen. De familie maakt relatief veel gebruik van uw diensten, zonder dat daar inkomsten tegenover staan. Eigenlijk weet u dat ze lang klant zullen blijven, maar niet echt winstgevend zullen worden. Met aCRM kunt u de marketinginspanningen richting de familie Woutersen reduceren. U kunt bijvoorbeeld het aantal aanbiedingen verminderen omdat u weet dat ze deze hoogstwaarschijnlijk toch niet zullen gaan kopen. Uiteindelijk gaat deze benadering u betere (financiële) resultaten opleveren. In veel gevallen wordt er meer omzet binnengehaald tegen minder kosten, omdat er minder klantcontacten zijn. Een ander voordeel is dat de klant minder wordt ‘lastiggevallen’ met acties waarop hij niet zit te wachten.
Een andere voorwaarde voor succesvolle toepassing van aCRM is het snel kunnen reageren op het gedrag van de klant en het dynamisch kunnen prioriteren van de meest winstgevende propositie. Snelheid is nodig omdat het bij mobiele telefoons gaat om secondewerk (in tegenstelling tot internet waarbij een klantcontact vaak minuten en soms uren duurt). De nieuwe generatie aCRM-software werkt real-time. Dat wil zeggen, het combineert allerlei soorten klantinformatie, pikt de juiste momenten eruit en komt zelf met een suggestie om de klant een bepaalde propositie aan te bieden. En dat allemaal binnen twee seconden.
Meer voorbeelden
Meneer Jager bijvoorbeeld is een winstgevende klant, en hij belt het callcenter. Er liggen een aantal verschillende proposities klaar waar hij mogelijk in geïnteresseerd is. Het ene aanbod is voor een nieuw beleggingsfonds, twee andere aanbiedingen gaan over spaarrekeningen en een vierde aanbod is voor een aandelenlease product. Op het moment dat meneer Jager iets in zijn beleggingsportefeuille verandert, worden de verschillende aanbiedingen nog eens doorgerekend. Enerzijds wordt uitgerekend welke propositie de hoogste winstbijdrage heeft. Anderzijds wordt gekeken naar de kans dat meneer Jager ook daadwerkelijk op een aanbod ingaat. Rekening houdend met de kans van slagen, krijgt meneer Jager de meest winstgevende propositie aangeboden.
Belangrijk verschil met eerder ontwikkelde tools is de snelheid, de flexibiliteit en intelligentie waarmee het berekenen van het meest gunstige aanbod gebeurt. Binnen een paar seconden wordt uitgerekend wat de winst is en vervolgens wordt degene met de hoogste winstverwachting eruit gepikt en gepresenteerd. Uiteindelijk stuurt de marketeer met zijn manier om klantcontacten te beïnvloeden effectief op de maximale winst per interactie.
Volgende voorwaarde is de actualiteit van het aanbod. Omdat de situatie van een klant gedurende klantcontact of net voor een klantcontact nog sterk kan veranderen, moet in het aanbod de meest recente veranderingen worden meegenomen. Dat geldt zeker voor de informatie over de plaats waar iemand zich bevindt (op het moment van bellen) en het tijdstip.
Meneer Pietersen bijvoorbeeld, van wie vooraf bekend is dat hij winstgevend kan worden, belt het callcenter op. Hij haalt vijfduizend euro van zijn rekening. De software signaleert dit en beoordeelt meneer Pietersen plotseling als een potentiële wegloper (in plaats van de potentieel winstgevende klant). Op basis van de laatste transactie, in combinatie met zijn achtergrond, komt de tool met een suggestie om meneer Pietersen een speciale aanbieding te doen met als doel hem te behouden als klant. In dit geval krijgt meneer Pietersen een incentive bij een product dat hem een jaar lang bindt aan het bedrijf. Meneer Pietersen is tevreden en een belangrijke klant is voorlopig behouden. En mevrouw Jansen die een huis zoekt in het noorden van het land en belt dichtbij een kantoor van een bank. Deze informatie wordt meegenomen in de suggestie over het verstrekken van een hypotheekadvies. Er wordt direct een afspraak gemaakt met de lokale adviseur die haar kan gaan begeleiden, of een offerte in een e-mail naar haar huisadres kan sturen.
Samenwerking met andere distributiekanalen
De nieuwe generatie aCRM-tools ondersteunt het proces van het beïnvloeden van klantcontacten via de mobiele telefoon. Maar mobiel bankieren staat niet op zichzelf. Het is onderdeel van het algehele marketingproces over alle distributiekanalen en media heen. Vandaar dat de software onafhankelijk werkt van de applicaties in de distributiekanalen. Ze kan het gehele marketingproces verbeteren door klantcontacten effectiever af te (laten) handelen. Dit gebeurt door de kanalen centraal, met gerichte suggesties, te adviseren. Soms is dit op verzoek van de kanalen zelf (=re-actief), en soms signaleert de software zelf een interessante kans (=pro-actief). De mobiele telefoon is hiermee een onderdeel van de integrale marktbenadering. Klantinformatie wordt van de mobiele telefoon, via de aCRM -software doorgegeven naar de campaign managementapplicatie, callcenterapplicatie, of internetapplicatie en vice versa. Zo ontstaat een effectief en efficiënt communicatieproces met de individuele klant, waarbij deze het idee krijgt dat iedereen in het bedrijf op de hoogte is van zijn situatie, en ook nog in staat is om hierop in te spelen. Daarmee is de software, vanuit een financieel oogpunt, ook nog eens een gigantische impuls voor het terugverdienen van eerdere CRM-investeringen, gedaan in bijvoorbeeld campaign managementsystemen en datawarehouses.
Conclusies
De nieuwe aCRM-software geeft u de kans om van mobiel bankieren een commercieel succes te maken. Het helpt u te achterhalen wie uw beste klanten zijn en met welke propositie u ze het beste kunt ‘verleiden’. Op het moment dat de klant u belt, signaleert het de beste momenten waarop u de propositie kunt aanbieden. Ze rekent uit welk actueel aanbod het bedrijf de meeste winst oplevert, waarbij het rekening houdt met de meest recente veranderingen in de situatie van de klant. Vervolgens zorgt de software dat deze propositie snel in een pakkende presentatie aan de juiste klant of medewerker wordt gepresenteerd. Uw marketing- en verkoopproces wordt effectiever. Naast de contacten via de mobiele telefoon worden andere interacties (via web, e-mail, call center, et cetera) gericht aangestuurd, waarbij er geen tijdverlies optreedt en er is bovendien consistentie en uniformiteit in de wijze waarop met de klant wordt gecommuniceerd. In een tijd van thuiswerken en decentrale callcenters kan een bank zich niet veroorloven om iedere medewerker zelf de keuze te laten bepalen welke status een klant heeft of hoe deze moet worden benaderd. Duidelijk is dat de software een zeer gunstige Return on Investment heeft, die relatief makkelijk te realiseren is. Zelfs met een beperkt aantal doelstellingen zijn in een zeer korte tijd al mooie winstgevendheidresultaten te halen.
Kosten reduceren? Winst maximaliseren!
Edith Kanters en Gert Haanstra, Tijdschrift voor Marketing, Kluwer, Deventer, Juni 2002
Printversie: Kosten reduceren Winst maximaliseren Corona case
Zeker nu het economisch even tegenzit, grijpen veel banken en verzekeraars naar het vertrouwde instrument kostenreductie. Het is namelijk relatief makkelijk om de marketing- en verkoopkosten terug te dringen door het marketingbudget te verlagen. Toch is dit niet het beste instrument om de effectiviteit van de marketing activiteiten te vergroten. Aan de hand van een case bij de Belgische verzekeraar Corona Direct illustreert dit artikel dat winstoptimalisatie een werkbaar en beter alternatief is.
Bedrijven worden gestuurd op hun aandeelhouderswaarde. Op het moment dat het economisch minder gaat, wordt minder geld uitgegeven om de totale waarde van het bedrijf op peil te houden. Er wordt dus op de budgetten voor de verschillende afdelingen en activiteiten gekort.
Bij een krapper marketingbudget zal de effectiviteit van de marketingactiviteiten omhoog moeten, is de redenering. Het marketingmanagement zal in de praktijk het aantal marketingactiviteiten gaan verminderen. Er wordt minder gemaild en gebeld, er worden minder prospects bezocht. Een slimmere manier om de effectiviteit te vergroten is de minder geïnteresseerde prospects uit te sluiten van de marketingactiviteit en zo de variabele kosten van postzegels en brochures te drukken. Maar men verwacht wel dat het aantal contracten dat met de actie wordt binnengehaald, gelijk blijft of in ieder geval niet teveel zal dalen.
Om tot het klantprofiel van niet geïnteresseerden te komen worden marktonderzoeken gedaan en wordt Analytische Customer Relationship Management (aCRM) software ingezet. Met behulp van de aCRM software kunnen de kenmerken van klanten en het gedrag dat zij in het verleden hebben vertoond worden geanalyseerd en daaruit kunnen klantprofielen worden vastgesteld. Deze klantprofielen voorspellen welke klanten het product vrijwel zeker niet zullen kopen.
Doodlopende weg
Blijft een bedrijf zich focussen op het reduceren van het marketingbudget door uitsluiten van niet geïnteresseerden dan worden de kosten wel steeds lager maar het aantal nieuwe contracten neemt dan ook sterk af. Immers, de kanslozen zijn dan wel uitgesloten van de actie, maar de kans dat ook serieus geïnteresseerde prospects worden uitgesloten, wordt steeds groter. De vraag bij een dergelijke kostenreductie strategie is dan ook: wanneer moet het bedrijf ophouden met het terugbrengen van het aantal prospects dat men wil benaderen? Het meest optimale punt is namelijk wanneer er niemand meer wordt benaderd aangezien de variabele marketingkosten dan nihil zijn. Maar niet meer benaderen resulteert in geen contracten en dus op de lange termijn in een negatief bedrijfsresultaat en is dus een doodlopende weg.
Uiteindelijk zijn bedrijven geïnteresseerd in de netto bijdrage van een marketingactie aan de winst. Die moet bij voorkeur zo hoog mogelijk zijn. Hoewel die bijdrage zeker te berekenen valt, zie je in de praktijk dat dat maar weinig gebeurt. Aan de hand van een bij verzekeraar Corona Direct uitgevoerde marketingcampagne zullen we illustreren dat het bepalen van winstoptimalisatie met de huidige aCRM technologie relatief eenvoudig is en leidt tot een effectievere marketingstrategie.
Case
Corona Direct is een Belgische direct verzekeraar die jaarlijks 1,5 miljoen mailpacks verzendt waarmee ze nieuwe klanten wil binnenhalen voor hun uitvaart¬verzekering. Deze verzekering keert een vast geldbedrag uit bij overlijden. Daarvoor betalen deelnemers 10 of 15 jaar lang een maandelijkse premie. Geïnteresseerde prospects bellen voor offerteaanvraag of sturen een coupon terug. Dit is gedefinieerd als de respons op een mailing.
De kosten van een dergelijke acquisitie mailing zijn relatief hoog. Vandaar dat Corona Direct met behulp van aCRM software de totale mailingkosten wilde verlagen (voor de najaarsmailing van 2001). De kostenreductie wilde men realiseren door minder geïnteresseerde prospects uit te sluiten. De analyse was geslaagd als de kosten van de vervolgmailing met minimaal 20 procent zouden worden gereduceerd, met een maximaal toegestaan responsverlies van 10 procent. Als test is in de analyse ook gekeken naar de mogelijkheden voor winstmaximalisatie.
Om inzicht te krijgen in de mogelijkheden tot kostenreductie op de mailing van najaar 2001 zijn twee stappen ondernomen: zoeken naar groepen met een hoge of lage respons en berekenen van de toegevoegde waarde.
Klantprofiel
Met behulp van aCRM software hebben we gezocht naar groepen van adressen met hogere of lagere respons dan gemiddeld op de najaar 2000 mailing. Een klantprofiel dat prospects beschrijft die nauwelijks reageren zou bijvoorbeeld kunnen zijn: “Buurten met relatief weinig alleenstaanden waarvoor op het adres in de afgelopen vijf jaar ooit eens een offerte is aangevraagd maar nooit een contract is gesloten”.
Voor de bepaling van de kostenreductie worden de mailkosten bepaald bij het bereiken van 90 procent (schatting) van de maximaal haalbare respons. In het geval van de winstmaximalisatie worden voor de gehele doelgroep de kosten en de verwachte opbrengst bepaald. Wanneer kostenreductie en winstmaximalisatie in een grafiek worden weergegeven, blijkt dat winstmaximalisatie in tegenstelling tot kostenreductie het beste resultaat (lees: netto winstbijdrage) oplevert voor Corona Direct. Bij kostenreductie wordt er geen inzicht verkregen in de kosten, baten en winstbijdrage van de actie en is de winst verre van optimaal. Het risico van de focus op kostenreductie wordt hier duidelijk geïllustreerd doordat prospects met “té dure respons” gewoon zouden worden benaderd.
Uit de winstanalyse voor deze mailing bleek dat er 21.250 euro meer winstbijdrage was te behalen door minder adressen te mailen. Oftewel de kosten zijn sterker gereduceerd dan de doelstelling en daardoor is de winstbijdrage van de actie hoger!
Toegevoegde waarde
Naast deze geldelijke meerwaarde van de mailing is er ook toegevoegde waarde door het inzicht in de winstverdeling van de mailing. Dit heeft als voordeel dat op basis van deze inzichten een (budget herzienings)beslissing kan worden genomen die in lijn ligt met de gewenste marketingbenadering. Als bijvoorbeeld het bedrijf marktaandeelmaximalisatie op een kostefficiënte wijze zou willen nastreven dan hoort daarbij de keuze voor het punt waarop de winstlijn de x-as kruist (het zogenaamde break-even punt). Hierbij is het bereik zo groot mogelijk zonder daarbij verlies te lijden.
Op de langere termijn bij Corona zullen meerdere marketingcampagnes op deze manier worden bekeken. De opbrengsten hiervan zijn nog moeilijk in te schatten zonder inzicht in de winstverdeling van deze marketingcampagnes. Maar ter indicatie: bij de extra gerealiseerde winstbijdrage en kostenreductie op deze marketingcampagne van respectievelijk 21.250 euro en 200.000 euro (ongeveer 44 procent van de totale variabele marketingkosten van 450.000 euro) zal het toepassen van dezelfde werkwijze op vier marketingcampagnes over het jaar 2002 kunnen resulteren in een extra winstbijdrage plus een kostenreductie van respectievelijk 85.000 en 800.000 euro.
Geld laten liggen
De case illustreert dat winstmaximalisatie meer geld oplevert dan kostenreductie en dat het bovendien eenvoudig te bepalen is. Corona Direct wist met het analyseren en optimaliseren van een eenmalige uitvaartcampagne ruim drie keer meer winst te maken. Bedrijven die alleen maar streven naar het reduceren van het marketingbudget laten dus nog steeds veel geld liggen.
Marketeers zouden niet direct meer akkoord moeten gaan met een verlaging van hun marketingbudget maar moeten teruggrijpen op winstbepaling van hun actie. Met behulp van aCRM software is het relatief eenvoudig om de netto winstbijdrage van een actie te bepalen. De totale verwachte baten minus de totale kosten van de in aCRM analyse op responskans gesorteerde doelgroep resulteert namelijk in de netto bijdrage aan de winst. Deze is eenvoudig in een grafiek weer te geven. Dit maakt de moderne aCRM een prima instrument om te sturen op de maximale winstbijdrage van een actie.
In een breder perspectief laat de case zien dat met winstmaximalisatie het verhogen van de customer lifetime value weer een stap dichterbij is gekomen. Nu nog wachten op de first mover die deze uitdaging aandurft.
Orginele publicatie: Klik hier
Customer Relationship Management werpt vruchten af
Gert Haanstra en Martijn Jansen, VVP Weekblad voor financiële dienstverleners, Nijgh Periodieken BV, Schiedam, 11 – 13 maart 2002, blz 18 en 19
Printversie: Customer Relationship Managment werpt vruchten af
Sinds kort zien we dat Customer Relationship Management succes heeft in het intermediair kanaal. Na een periode van proberen, en misschien wel een hoop frustraties, boekt een aantal verzekeraars en intermediairs interessante resultaten. Gezamenlijk halen ze meer rendement uit hun klanten door bijvoorbeeld een besparing van de marketingkosten en een vermindering in het aantal (voortijdig) beëindigde contracten.
Meest aansprekend zijn de cross sell resultaten, waarmee verzekeraars meer omzet en intermediairs meer provisie weten binnen te halen. In dit artikel (een tweeluik) gaan we in op hoe de koplopers in de branche het hebben aangepakt. In het eerste deel laten we zien welke kansen er zijn en wat het uiteindelijk kan opleveren. En in het tweede deel besteden we aandacht aan (rand)voorwaarden om als intermediair succesvol te kunnen zijn met CRM.
Waarom Customer Relationship Management?
CRM legt de focus op het aangaan en onderhouden van de relatie met elke individuele klant. Doel is de klantrelatie zo lang mogelijk te laten duren en zoveel mogelijk in de behoefte van de klant te voorzien op een wijze die winstgevend is voor beide partijen. Maar wat is de daadwerkelijke toegevoegde waarde van CRM voor verzekeraars en intermediairs?
CRM is een effectieve aanpak om het eigen marktaandeel veilig te stellen. Hoewel het intermediair nog steeds een belangrijke pijler is waarop de verzekeringswereld rust, ondervinden intermediairs én verzekeraars in meer of mindere mate de druk van nieuwe marktontwikkelingen. Bijvoorbeeld met de komst van internet kunnen consumenten relatief eenvoudig (simpele) producten met elkaar kunnen vergelijken en ook nog eens direct afsluiten.
Een andere belangrijke ontwikkeling blijft het toenemend consumentisme. Klanten zijn mondiger en vluchtiger dan ooit. Het bieden van goede diensten is een must en gaat verder dan goede producten tegen een faire prijs. Op distributiegebied zijn er allerlei branchevreemde samenwerkingen waarbij financiële producten via allerlei verkooppunten als supermarkten, pompstations aan de man worden gebracht. Zowel verzekeraars als intermediairs hebben zich ergens gerealiseerd dat Customer Relationship Management een goed middel is om op de ontwikkelingen te reageren. Streven is de band met bestaande klanten aan te halen en te achterhalen waar de commerciële kansen liggen. De combinatie van goedgevulde klantendatabases, een geoliede marketing apparaat en een sterke loyaliteit van klanten met hun intermediair, biedt dan ook goede mogelijkheden om de winst, bijvoorbeeld met behulp van cross selling te vergroten.
Welke mogelijkheden zijn er?
Met CRM gaan zowel intermediairs als verzekeraars meer verdienen; de ene meer omzet, de andere meer provisie. Door een gezamenlijke aanpak gericht op de individuele klant zijn verzekeraar en intermediair beter in staat om nieuwe klanten binnen te halen, en bestaande klanten te cross sellen en te behouden.
Beter benutten leads. Potentiële klanten zoeken niet altijd het intermediair op om informatie in te winnen over (nieuwe) producten of voor vragen over hun persoonlijke situatie. Regelmatig komt men rechtstreeks bij de verzekeraar ‘binnen’. Maar wat gebeurt er als potentiële klanten zelf het initiatief nemen en contact zoeken met de verzekeraar via het call center of de website? Mag de verzekeraar de propositie aanbieden omdat op zo’n moment de kans zich voordoet? Of moet hij de klant doorsturen naar het intermediair met het risico dat hij in de tussentijd een ander product vindt en koopt? In een ideale situatie zou de verzekeraar op het moment dat een klant contact zoekt via een van de distributiekanalen, en er zich een kans voordoet, de klant iets mogen verkopen. Voorwaarde is dat de provisie wordt uitgekeerd aan het intermediair, en dat het intermediair ‘mede-eigenaar’ wordt van de nieuwe klant.
Gerichte cross selling. Voor het cross sellen geldt, als voorbeeld, dat in een klantenbestand wordt ontdekt dat bij de groep ‘ziektekostenverzekerden’, degene met kinderen vaker ook nog een levensverzekering hebben afgesloten. Uit analyse blijkt verder dat veel ziektekostenverzekerden nog nooit een levensverzekeringsaanbod hebben ontvangen. Door nu een inschatting te maken van de verwachte hoogte van het contract, kan men beslissen om de grootste en meest kansrijke contracten door te sturen naar het intermediair. Uit de analyse blijkt dat er al een grote kans is dat er een contract wordt afgesloten. Nu is het aan het intermediair om te proberen er een groter contract uit te slepen.
De groep waar men kleinere contracten verwacht te verkopen, geeft men een lagere prioriteit. Of ze als lead worden doorgestuurd, hangt af van de capaciteit van het kantoor (en de kosten die hiermee gepaard gaan). Soms is er al voldoende werk voor de komende weken/maanden. Doorsturen zou zonde zijn, want dan blijft de kans misschien te lang liggen. Dus kiest men voor een directe benadering met een mailing of telefoontje.
Bij de groep waarbij er een kleine kans is dat een contract wordt afgesloten, maakt men een duidelijke kosten/baten afweging. Men moet uit de kosten komen, en zet daar de kans om het contract binnen te halen tegenover. Een goedkope, eenmalige mailing is dan veelal effectiever.
Effectief behoud. Bijvoorbeeld, door activiteiten van concurrenten dreigen ziektekostenverzekerden in het zuiden van Nederland hun contract op te zeggen. Verzekeraar en intermediairs willen graag hun klanten behouden en bedenken samen een actie; een service gesprek waarin afhankelijk van de kans dat iemand wegloopt en de duur dat iemand klant is, een kortingspercentage wordt aangeboden voor het volgend jaar. Vervolgens stellen de intermediairs hun klanten ‘beschikbaar’ voor deze marketing actie die verder wordt uitgevoerd door een extern call center.
Een andere mogelijkheid is om de diverse behoudpogingen achteraf te evalueren. Uit een analyse blijkt dan bijvoorbeeld dat klanten met een autoverzekering beter reageren op een dwingende brief, en dat klanten met een levensverzekering beter reageren op telefoontje van hun intermediair. Zaak is deze segmentatie over de bestanden van de intermediair en verzekeraar heen te gebruiken om het optimale resultaat te krijgen.
Duidelijk is dat CRM ook voor intermediairs als verzekeraars allerlei kansen biedt om meer te verdienen. In praktijk ontwikkelt men een gezamenlijk proces waarbij men ontdekt waar de kansen liggen, om vervolgens in onderlinge afstemming daar met een passend aanbod gericht op in te gaan.
Welke resultaten kan men behalen?
Het gaat om meer omzet en meer provisie, omdat de meest kansrijke groepen het eerst worden benaderd. En daarnaast gaat het om een besparing op de marketing- en verkoopkosten, omdat er minder wordt verstuurd/gebeld doordat de kansloze groepen worden uitgesloten.
Mits goed aangepakt, is het mogelijk om een groot deel van de intermediairs te interesseren voor een actie. Er zijn cijfers behaald waarbij meer dan ¾ van de aangesloten, actieve intermediairs hebben meegedaan aan een outbound marketingactiviteit. Deze actie was opgezet om een pensioenproduct te verkopen via cross selling. Hiervoor werden eerst de bestanden geanalyseerd om te kijken wie het meest kansrijk was (en wie kansloos was) om het product te kopen. Vervolgens werd onderscheid gemaakt tussen klanten met een gerede kans op een hoge inleg, en kopers met een relatief lage inleg. Klanten met een goede kans op een hoge inleg werden doorgestuurd als lead naar het intermediair. Anderen ontvingen een mailing, verstuurd door de verzekeraar uit naam van het intermediair. Uiteindelijk werd op 15% tot 25% van de leads een afspraak gemaakt, met uitschieters van meer dan 40%.
Conclusies
Customer Relationship Management is commercieel gezien ook interessant voor het intermediair kanaal. Een succesvolle samenwerking tussen intermediair en verzekeraar, gebaseerd op wederzijds vertrouwen, is nodig om de winstgevendheid en loyaliteit van klanten te vergroten. Door effectief en efficiënt te klantcontacten af te handelen worden meer klanten behouden of meer cross sell bereikt. Met als directe resultaat; hogere omzet voor de verzekeraar, en meer provisie voor de intermediairs.
In het tweede artikel in het volgende nummer wordt ingegaan op hoe intermediairs en verzekeraars de succesvolle samenwerking kunnen bereiken. Randvoorwaarden worden besproken als het door de klant laten aansturen van het interactieproces, het geven van duidelijkheid over de spelregels, het maken van afspraken over het halen en brengen van klantinformatie en het verdelen van de kosten/opbrengsten.
Orginele publicatie: Klik hier
Basisvoorwaarden voor CRM succes in intermediairkanaal
Gert Haanstra en Martijn Jansen, VVP Weekblad voor financiële dienstverleners, Nijgh Periodieken BV, Schiedam, 13 – 27 maart 2002, blz 20 en 21
Printversie: Basisvoorwaarden voor CRM succes in intermediairkanaal
In het VVP van concludeerden wij dat Customer Relationship Management commercieel gezien ook interessant is voor het intermediair kanaal. Door effectief en efficiënt de klantcontacten af te handelen worden meer klanten behouden of meer cross sell bereikt. Met als directe resultaat: hogere omzet voor de verzekeraar en meer provisie voor de intermediairs.
Samenwerking en afstemming tussen intermediair en verzekeraar is nodig om met Customer Relationship Management succesvol te zijn in het intermediairkanaal. Elkaar helpen om klanten te behouden en te cross sellen, is niet af te dwingen met het kopen van louter CRM software of een project starten om iets met CRM te gaan doen. Creëren van de bereidheid om elkaar te helpen is vaak een kwestie van elkaar leren vertrouwen. De koplopers in de branche zijn hierin geslaagd en hebben een succesvolle samenwerking gerealiseerd. Wat is hun geheim? Want waarom lukt het hen nu wel, en anderen nog steeds niet? In tweede deel laten we zien hoe de koplopers in de branche, de belangrijkste voorwaarden voor succes hebben ingevuld.
Een succesformule?
Om gezamenlijk effectief en efficiënt klantcontacten af te handelen is het nodig om de klant zelf het interactieproces te laten aansturen, duidelijkheid te creeëren over de het proces, afspraken te maken over het halen en brengen van klantinformatie, en is het nodig om een verdeling te maken van de kosten/ opbrengsten.
De klant stuurt het proces aan. De voorkeuren van de klant zijn sterk leidend bij de manier waarop marketing activiteiten worden opgezet. Door goed te ‘luisteren’ naar de klantinformatie in de databases krijgt men een goed beeld van wat de klant wil en verwacht. Daarnaast kan men de klant rechtstreeks vragen wat hij wel en niet wil. Met analyses achterhaalt men dan wie het beste kan worden benaderd, met welk aanbod, via welk distributiekanaal en wanneer. Door de marketing activiteiten af te stemmen op deze klantinformatie laat men als het ware de klant ‘beslissen’.
Hiernaast vindt er ook nog steeds een bedrijfsmatige afweging plaats waarbij wordt gekeken naar de verwachte winst (ook op lange termijn) en de kosten die verzekeraar en intermediair hiervoor moet maken. Bovendien is er afstemming nodig in activiteiten tussen de verschillende distributiekanalen, want pas dan krijgt de klant het idee dat goed en consistent met hem wordt gecommuniceerd.
Transparantie in het proces. Dit betekent openheid in wat men met de klant wil bereiken en overeenstemming krijgen in hoe men dit gaat aanpakken. Duidelijk moet zijn hoe ‘het CRM spel’ wordt gespeeld. Vaak draait het hierbij om wie de klant gaat benaderen en met welke propositie?
‘Transparantie’ heeft tot doel om sluimerende kanaalconflicten voortijdig te vermijden. De eerste stap is mensen bij elkaar te brengen, en te laten praten over de (on)mogelijkheden van CRM. In een volgende stap moet zichtbaar worden hoe er wordt samengewerkt. Concreet, en niet alleen in regels, procedures, etc. Het gaat om ‘voorbeeldgedrag’; laten zien hoe jijzelf en de ander zich in een bepaalde situatie gedragen. Eenmaal aan de slag, moet het verder ontwikkelen en verfijnen van de werkwijze gefaciliteerd worden. Continue moet inzichtelijk zijn waar dingen beter kunnen en waar fouten dreigen te ontstaan. Op het moment dat er geen duidelijkheid is, kan het gevoel ontstaan dat verzekeraar en intermediair vechten om dezelfde klant en transactie. In het uiterste geval is er van samenwerking dan geen sprake meer.
Mensen doen vrijwillig mee, maar hebben wel hun verwachtingen. Duidelijk is dat samenwerken een kwestie van leren is. In eerste instantie zal er tijd moeten worden ingeruimd om relatief veilig dingen uit te proberen en hierover feedback te krijgen. Vooral over hoe het de samenwerking beïnvloedt. Dus als het samenwerkingsproces een andere kant op gaat dan verwacht, en mensen zich hierbij niet prettig voelen, moet snel een passende oplossing worden verzonnen. Kunst is om te blijven leren van de ondernomen acties. Minimale eis is dat acties goed gemeten en geanalyseerd worden. Op basis van de analyse kan bijgestuurd worden en is sprake van een lerende organisatie. In die zin is de implementatie van CRM nooit ‘klaar’.
Een haal- en brengplicht voor beide partijen. Zowel verzekeraar als intermediair moet zich inzetten om van CRM een succes te maken. Dat heeft zijn rechten en plichten. Vandaar dat verzekeraar én intermediair elkaar volledig moeten informeren. En dus een haal- en brengplicht hebben wat betreft recente klantgegevens, marketingactiviteiten, (financiële) resultaten, etc.
Bijvoorbeeld in geval van een marketing actie, geeft de verzekeraar informatie aan het intermediair over wat er met de klant is gebeurd, wat dit heeft gekost en hoeveel het heeft opgebracht. En koppelt het intermediair terug welke follow-up activiteiten zijn uitgevoerd, of er een contract is gesloten, en wat hij nog meer van de klant te weten is gekomen.
Om altijd en overal effectief met de klant te kunnen communiceren, is het nodig om informatie uit de klantcontacten consequent vast te leggen (bijvoorbeeld in een centrale database), te consolideren, en weer te verspreiden naar de kanalen/voorkant. Hierbij gaat het natuurlijk om contracten, contacten, betalingen, informatie-aanvragen, klachten, etc. Maar het geldt ook voor gemaakte afspraken, geplande activiteiten, etc.
Het halen en brengen van informatie werkt goed met de zogenaamde ‘druppeltechniek’. Verzekeraar en intermediairs kunnen ‘inprikken’ op een centrale database waarbij pas nieuwe informatie wordt verstrekt als eerder verstrekte informatie is gelezen, bijgewerkt of opgevolgd. Voordeel is dat klanten niet ergens worden ‘vergeten’ of dat er ongecoördineerde acties worden uitgevoerd. Zaak is overzicht te creëren met name afgezet tegen de gewenste/verwachte resultaten. En op basis van deze informatie nieuwe activiteiten te ontplooien.
Verdeling van kosten én opbrengsten. Wil men gezamenlijk de klanten behouden en cross sellen dan moet het financieel goed geregeld zijn. In de praktijk betekent dit dat als de verzekeraar een marketing actie opzet en rechtstreeks een contract afsluit (op het bestand van het intermediair) dan krijgt het intermediair zijn provisie (gewoon) uitgekeerd. Zo krijgt de verzekeraar zijn omzet en het intermediair zijn provisie.
Verstrekt de verzekeraar een lead dan betaalt het intermediair voor deze lead. Het is een compensatie voor gemaakte marketingkosten als het analyseren en selecteren van het klantenbestand. Het intermediair houdt de vrijheid om de klant een ander product aan te bieden dan de lead van de verzekeraar. Maar daarmee neemt hij het risico dat het aanbod een minder grote kans van slagen heeft. En dat de verzekeraar zich minder kan vinden in de samenwerking.
De financiële afspraken zijn bedoeld om gezamenlijke activiteiten te stimuleren zodat men er beide beter van wordt. Want gebeurt er niets dan lopen verzekeraar en intermediair beide omzet en provisie mis, en blijft cross sell potentieel onbenut.
Conclusie
Pas als de samenwerking tussen intermediair en verzekeraar tot stand komt, wordt Customer Relationship Management commercieel gezien ook interessant voor het intermediair kanaal. Een aantal koplopers is hierin geslaagd. Zij laten de klant het interactieproces aansturen, zijn duidelijk over de spelregels, hebben duidelijke afspraken gemaakt over het halen en brengen van klant-informatie, en verdelen de kosten/opbrengsten.
Met name voor het intermediairkanaal is CRM een manier van werken die recht doet aan de relatie met de klant. Gebaseerd op wederzijds vertrouwen, zal het de winstgevendheid en loyaliteit van klanten vergroten. Met als directe resultaat; hogere omzet voor de verzekeraar, en meer provisie voor de intermediairs.
Orginele publicatie: Klik hier
Geld verdienen met feiten en cijfers
Gert Haanstra, Telecommerce.nl, BBP bv, Woerden, Nr 10, oktober 2008
In vier stappen naar effectief klantrendement: intelligence
Printversie: Geld verdienen met feiten en cijfers
Intelligence-afdelingen van bedrijven en overheid breiden uit en/of vernieuwen. En die investeringen zijn terecht, zegt Gert Haanstra, want er is veel geld te verdienen met een op feiten en cijfers gebaseerde (klant)benadering. Om je intelligence tot volle wasdom te laten komen, helpt een stappenplan. Vooral de samenwerking met marketing en andere frontoffice-afdelingen verdient hierbij speciale aandacht, aldus de adviseur.
Intelligence raakt velerlei klantzaken in je organisatie, denk onder andere aan je CRM-, BI-, sales-, concurrentie- en fraudestrategie (lees kader). Een goed functionerende intelligence-afdeling is dan ook noodzakelijk. Die kun je in vier stappen verbeteren. Naast het werk om het een en ander te realiseren, bepalen vooral de heldere keuzes die je daarbij moet maken het uiteindelijke succes. Is duidelijk waar de toegevoegde waarde van de afdeling komt te liggen en welke rol intelligence inneemt ten opzichte van de rest van het bedrijf, is het zaak om de inrichting, de kwaliteit van de bemensing en de aansturing hierop af te stemmen. Pas dan kan de kennis van de eindklant daadwerkelijk ten gelde worden gemaakt. Het vierstappenplan ziet er als volgt uit.
Stap 1: een stevig fundament
Het is als bedrijf zinvol om te toetsen of de benodigde expertise voldoende aanwezig is én of het aanwezige potentieel wordt benut. Het lijkt zo vanzelfsprekend en het is zo logisch, maar toch lijken niet alle intelligence-afdelingen zich hiervan bewust. De afdeling zal over de juiste kennis en over voldoende ervaring moeten beschikken. Dan gaat het met name om technische expertise op gebied van analyse en onderzoek; het opzetten van onderzoek, het verzamelen van de juiste gegevens, het analyseren met behulp van (statistische) tooling, de vertaling naar concrete acties, tot aan het (laten) gebruiken van de opgeleverde resultaten door management, marketing, et cetera.
Die expertise is terug te vinden in functies als (business/marketing)analisten, (markt)onderzoekers, databasemarketeers en marketingconsultants. Dan gaat het om zaken als het selecteren van prospects, maken van klantprofielen, voorspellingsmodellen, segmentaties, rapportages, verkoopondersteuning, et cetera. Maar ook om concurrentie- en overheidanalyse en trendwatching en meer recente onderwerpen als web 2.0-analyse, behavioral targeting, social networking en fraudedetectie. Mocht specifieke expertise ontbreken, dan kan het natuurlijk worden ingekocht, ontwikkeld of tijdelijk ingehuurd. Idealiter heeft de afdeling het potentieel in zich om de stap te maken richting een implementerende rol.
In de praktijk zien we op de intelligence-afdeling van een direct writer al klantwaardemarketeers werken die event driven marketing-programma’s en klantwaardeprogramma’s ontwikkelen, uitproberen en optimaliseren voordat ze door de marktmanagers ‘in productie’ worden genomen.
Stap 2: een duidelijke positionering
De volgende stap is zelf een heldere positie te claimen. Door allerlei nieuwe begrippen als customer insights, intelligence 2.0 en dergelijke is er veel ruis bij managers, directieleden, maar ook andere collega’s in de organisatie over wat ze tegenwoordig van intelligence mogen verwachten. De termen geven de indruk dat het om een totaal nieuwe wereld gaat en creëren een verwachting dat de intelligence-afdeling er is voor al je vragen en problemen. Dat kan natuurlijk alleen maar leiden tot teleurstellingen.
Zaak is een heldere positionering te kiezen en die actief uit te dragen. Voordeel is dat de afdeling zelf wordt gedwongen om na te denken over waar hun toegevoegde waarde ligt. Is het een wetenschappelijke afdeling (geïnspireerd door product leadership) die iedere keer met de mooiste onderzoeken, de meest innovatieve methoden en met zeer verrassende inzichten komt? Is het een productieafdeling (geïnspireerd door de operational excellence-gedachte) die volgens het principe van ‘u vraagt, wij draaien’ met zoveel mogelijk standaardrapporten, selecties, onderzoeken, analyses komt? Of is het een serviceafdeling (volgens de customer intimacy-aanpak) die de interne klantvraag centraal stelt, luistert naar ‘interne klanten’ (lees: alle afdelingen en medewerkers in het bedrijf) en probeert die zo goed mogelijk te vertalen naar een op maatwerk gemaakte oplossing?
Veel afdelingen worstelen met hun positionering. Kenmerkend is de ontwikkeling bij een energieleverancier. De intelligence-afdeling aldaar heeft zich van een productieafdeling kunnen ontwikkelen naar een serviceafdeling. Resultaat was een grotere, interne klanttevredenheid en aanzienlijk meer respect bij medewerkers en management. Een tweede voorbeeld is de afdeling van een verzekeraar die van ‘u vraagt, wij draaien’ de omslag wist te maken naar ‘proactief meedenkend’.
Met een heldere positionering verduidelijk ook de eigen rol in verhouding tot de rest van het bedrijf. De eerdergenoemde operationele rol is bekend. Maar wanneer en hoe gaat intelligence de meer strategische, adviserende rol op zich nemen? Waarbij de afdeling het management met (on)gevraagd advies duidelijk maakt welke richting het bedrijf op termijn uit zou moeten, wil het optimaal kunnen inspelen op ontwikkelingen bij de klant. Dezelfde vragen gelden voor de innovatieve rol waarbij een intelligence-afdeling, energiek en zonder oordeel met allerlei verrassende inzichten en oplossingen komt om de diverse (marketing- en verkoop)plannen te laten realiseren. En er is de gewetens-, meer toetsende rol waarbij de afdeling achteraf controleert, verklaart en een oordeel velt over het wel of niet behalen van de gestelde financiële (klant)doelen.
In de praktijk worden de rollen nog nauwelijks onderkend. Bovendien missen veel medewerkers de vaardigheid om met de verschillende rollen te ‘spelen’. Overduidelijk voorbeeld is een grootbank waarbij de medewerkers zich vooral blijven opstellen als toetsende, voorzichtige, gewetensvolle analisten. Veel initiatieven van buitenaf worden beantwoord met de opmerking ‘ja, maar…’. Een gemiste kans.
Stap 3: een verbeterde samenwerking
Richting een optimaal rendement van intelligence is het dus belangrijk om de samenwerking met de rest van het bedrijf te verbeteren. Vooral ook die met de marketeers in de directe omgeving. Hier is veel te winnen. Nogal wat intelligence-afdelingen krijgen het verwijt dat ze solistisch en in een ivoren toren werken. En dat hun manier van communiceren enerzijds te inhoudelijk en anderzijds te vrijblijvend is.
Intelligence leunt vaak zwaar op de inhoud en op de bewijsvoering. De afdeling zou zich wat meer kunnen richten op het verhaal achter de cijfers en de vorm waarin de boodschap wordt gebracht. Naast vaardigheid is dit vooral een kwestie van durven. Durven te gaan voor de grote lijnen, de details even vergeten en de cijfers te zien als onderbouwing voor waar het bedrijf naar toe zou moeten. Maar bovenal gaat het om samenwerking. Proberen de afstand met opdrachtgevers en afnemers te verkleinen. Bijvoorbeeld met simpele oplossingen als door te luisteren naar een interne klant en te laten zien dat de vraag is begrepen, door hem zijn eigen inbreng te geven/gunnen, door hem te helpen met het structureren van zijn probleem, door begrip te tonen voor de uitdagingen van de opdrachtgever, door ongevraagd inzicht te geven in de eigen afwegingen, ga zo maar door.
Naast inlevingsvermogen en flexibiliteit ten aanzien van de wensen van collega’s, wordt de effectiviteit vergroot op het moment dat de intelligence-afdeling een minder vrijblijvende houding aanneemt dan nu nog gebruikelijk is. Het blijft bij intelligence gaan om het snel, goed, volledig verzamelen van gegevens en het structureren, analyseren, modelleren van de gegevens tot zinvolle informatie. Om die vervolgens op het juiste moment, in de juiste vorm, op de juiste plaats bij de juiste persoon zien te krijgen. En de reacties op de informatie vastleggen, die evalueren en verwerken tot betere proposities. Maar in de nieuwe rol worden van intelligence dwingende inzichten en richtinggevende aanbevelingen verwacht. In tegenstelling tot de ‘traditionele’ aanpak die zich beperkt tot het correct presenteren van cijfers en het juist weergeven van de werkelijkheid, gaat het bij intelligence om de scherpte van de conclusies en de reikwijdte van de aanbevelingen. En dus over het in beweging krijgen van de rest van het bedrijf.
Een mooi praktijkvoorbeeld is een telecommaatschappij. De klantwaardeoverzichten van analisten zijn leidend geworden in de bedrijfsvoering en bepalen de activiteiten richting de klant en wat voor aanbod een klant mag verwachten.
Stap 4: een effectieve aansturing
De laatste stap in het maximaliseren van het rendement van een intelligence-afdeling is die van een effectieve aansturing. Van nature zijn nogal wat bedrijven ongeduldig en gericht op kortetermijnverkoop. Gelijktijdig hebben heel wat managers de neiging om medewerkers te wantrouwen en ze te willen controleren met KPI’s, productietargets en urenverantwoording. Een dergelijke benadering is funest voor de effectiviteit van een intelligence-afdeling. Die afdeling bestaat uit professionals. Dat betekent nogal eens dat de medewerkers meer verstand (van zaken) hebben dan de manager zelf. Kenmerkend voor die professionals is hun zelfstandigheid, hun kritische houding ten aanzien van leidinggevenden en hun grote betrokkenheid bij de inhoud van het werk. Dat vraagt om een andere manier van leidinggeven, die meer gericht is op inspiratie, prikkeling en uitdaging, en minder controle en beheersing van het uitvoerende proces. Het willen afdwingen van controle leidt vaak tot onderpresteren en het onbenut blijven van veel mogelijkheden.
De vraag is wie voor een intelligence-afdeling een goede leidinggevende is? Is die een meer inhoudelijke professional of is die een generieke manager? Een internopgeleide medewerker of een extern aangetrokken persoon? Idealiter is het een luis in de pels die voldoende reputatie heeft om het uiterste van zijn medewerkers te mogen vragen. Richting zijn medewerkers is hij in staat om vertrouwen te winnen dat nodig is om ze over hun eigen grenzen heen te laten gaan. Bovendien is de leidinggevende in staat om vragen en ontwikkelingen binnen het bedrijf te vertalen naar kansen voor de intelligence-afdeling. Naar buiten toe is de leidinggevende vaak het boegbeeld die het verhaal, de visie en de positionering vertelt. En die zijn afdeling op sommige momenten uit de wind houdt om ze de rust en de ruimte te geven zichzelf te ontwikkelen en op onderdelen te experimenteren.
In mijn ervaring neemt het belang van een effectieve aansturing toe naarmate de afdeling in een fase verkeert waarbij er nog grote onduidelijkheid is over wat en hoe het werk te doen. Of dat er nog onzekerheid is over de eigen rol, de plaats in de organisatie en mogelijkheden om zaken te veranderen. Of dat er onervarenheid is met het versterken van de intelligence-rol en het vergroten van de invloed op de wijze waarop klantwaarde wordt behartigd.
Een goed voorbeeld is een direct writer waarbij een (externe) leidinggevende in staat is geweest om inhoudelijk richting te geven, een veilige omgeving neer te zetten. Die de afdeling naar buiten toe sterker wist te profileren. Resultaat was een beter renderende afdeling met medewerkers die zich sterk wisten te verbeteren.
De vertaalslag
Heb je de vier stappen doorlopen, dan kun je de klantkennis vertalen naar verbeteringen binnen het bedrijf en die zaken (laten) realiseren die nodig zijn om de beste klanten langer vast te kunnen houden en beter te laten renderen.
360 graden-benadering intelligence
In enge zin gaat het bij intelligence om een zogenoemde 360 graden-benadering, waarbij de eigen gegevens uit databaseanalyses worden gecombineerd met gegevens uit kwalitatief/kwantitatief (markt)onderzoek. Dat raakt in de volle breedte zaken als:
• customer intelligence: het benutten van kansen door het opbouwen en analyseren van klant(waarde)kennis verkregen uit de eigen databases, marktonderzoek, klantcontacten, et cetera;
• business intelligence: het systematisch verwerven, verwerken en verspreiden van informatie ten behoeve van de strategievorming van het bedrijf;
• sales intelligence: het leveren van inzichten, verkoopsuggesties en leads aan de verkoopkanalen voor het commercieel effectief en efficiënt afhandelen van de klantcontacten;
• market/competitive intelligence: het in kaart brengen en duiden van ontwikkelingen bij concurrenten en bij de overheid, met het doel hier adequaat op te reageren;
• fraud intelligence: het verzamelen en structureren van informatie uit het interne proces en uit de gedragingen van klanten, met het doel om misbruik vroegtijdig te signaleren en zoveel mogelijk te voorkomen.
Datamining en analyse
Een aantal artikelen over de betekenis van analyse en datamining. Daarnaast twee artikelen over het voordeel van ‘open software’. En verder een checklist voor het succesvol implementeren van CRM.
Datamining in direct contact met de klant
Gert Haanstra en Melbert van Emmerik. CustomerBase, customer loyalty & database marketing, F&G Publishing, Amersfoort, Nr. 3, 1999
Printversie: Datamining in direct contact met de klant
De rol van data mining binnen het marketing- en verkoopproces evolueert. In de beginfase leek data mining een proeftuin om te laten zien dat inzicht in het klantenbestand geld kan opleveren. In veel gevallen bewees het zijn meerwaarde door successen te herhalen en het marketingproces structureel te verbeteren. Tegenwoordig eist het steeds vaker zijn plaats op; midden in de organisatie en in direct contact met de klant.
Te lang is data mining buiten het marketing- en verkoopproces gehouden. Gebrek aan kennis en goede data, twijfels over de haalbaarheid van investeringen, de angst om algoritmen mee te laten beslissen, en een dosis conservatisme zorgen ervoor dat nog weinig bedrijven data mining gebruiken. Wel dragen analisten soms voorspellingsmodellen of score cards aan, maar van het structureel inzetten van data mining binnen het campagne management is nauwelijks sprake. Een klein percentage bedrijven blijkt wel in staat de eigen organisatie aan te passen op het gebruik van data mining en daarmee de mogelijkheden ervan volledig te benutten. Recente ontwikkelingen laten zien dat de mogelijkheden van data mining verder gaan dan haar oorspronkelijke analysefunctie. Het zoeken naar onverwachte patronen in de database, het verklaren van gedrag en het maken van voorspellingsmodellen, maakt plaats voor het direct en interactief inzetten van de verkregen klantinformatie. Een enkele organisatie is toe aan de uitdaging om data mining in te zetten als essentiële schakel in het onderhouden van klantrelaties. Niet alleen in het out-bound campagne management, maar ook in de directe interactie met de klant.
In dit artikel beschrijven we hoe data mining zich ontwikkelt en op welke manier het ingrijpt in het bedrijfsproces. We laten de veranderingen in doelstellingen, onderzoeksvragen en methoden zien. Daarnaast gaan we in op de nieuwe verantwoordelijkheden en de consequenties die de nieuwe positie heeft binnen de organisatie.
‘Eerste generatie data mining; vluchten of vechten!’
Data mining begint met het uitproberen van de mogelijkheden. Zo’n eerste stap heeft als functie om het bedrijf warm te maken voor data mining. Wat kan data mining voor het bedrijf betekenen? Levert het geld op? Zo ja, hoeveel? Welke investeringen gaan ermee gepaard? En op welke gebieden vereist het organisatorische aanpassingen? Bedrijven begrijpen dat klantinformatie geld oplevert, en proberen met behulp van data mining de benodigde informatie uit de databases te krijgen. Enerzijds om de effectiviteit van de marketing inspanningen te vergroten. Anderzijds om nieuwe verkoopmogelijkheden te ontdekken in het klantenbestand. Een eerste grote succes prikkelt het bedrijf om aandacht te geven aan de mogelijkheden.
Er wordt het een en ander uitgeprobeerd met het eigen klantenbestand: een grote analyse, het bouwen van een scoremodel, het vinden van nieuwe klantsegmenten. Bij een gedegen teststrategie en voldoende aandacht voor de creatie van afgeleide voorspellende klantkenmerken kan het succes van data mining niet uitblijven. In de ideale situatie werken verschillende disciplines samen om hun eerste resultaten te behalen. IT-specialisten om ruwe data uit de diverse transactie bestanden te verzamelen en vast te leggen in een eerste data warehouse. Marketeers om input te geven aan de bruikbaarheid van de klantinformatie. En analisten voor het bewerken van de data en het ontwikkelen van een voorspellingsmodel. Duidelijk is dat data mining in deze eerste fase vanaf de zijlijn meedoet aan het verkoopproces. Bij aantoonbaar succes gaat de deur open voor een structurele rol in het marketing & verkoop proces.
‘Tweede generatie data mining: continu mining, continu leren!’
Komt er een vervolg na de ‘pioniersfase’, dan staat de tweede fase de integratie van data mining in het marketing- & verkoopproces centraal. Enerzijds om data mining consequent te gebruiken en maximaal te profiteren van de opbrengsten. Anderzijds om ontwikkelde voorspellingsmodellen continu te evalueren en verder aan te scherpen. De integratie moet uiteindelijk leiden tot structurele verbeteringen in de actieresultaten.
Het streven is continuïteit. Continuïteit in het verbeteren van de kwaliteit van de modellen. Continuïteit in het creëren van meer modellen voor nieuwe doelgroepen en voor andere producten. En continuïteit in het binnenhalen van meer omzet. Ofwel, continuïteit in het optimaliseren van het commerciële proces.
In de praktijk betekent dit het opzetten van testcycli om voortdurend de juiste mix van klanten, producten, kanalen en timing te achterhalen. Wij noemen dit het data mining spel. Verschillende acties worden specifiek opgezet om nieuwe modellen te bouwen. Daarnaast worden bestaande modellen aangescherpt door ze te gebruiken en tegen oude modellen te testen. Uiteindelijk leiden deze cycli tot een set kwalitatieve goede voorspellingsmodellen voor de meeste ‘standaard’ acties.
Voorheen gaven analisten voornamelijk vrijblijvend advies aan marketeers over de te benaderen klantgroepen. Met een integratie van data mining, ontstaat een duidelijke verantwoordelijkheid in het continu vinden van nieuwe, beter scorende doelgroepen in de database. Een discipline die in eerste instantie ligt bij analisten, maar die een sterke samenwerking met data specialisten en marketeers vereist voor maximaal succes. Op basis van het profiel van gevonden klantgroepen wordt tezamen met de marketeers een geschikte campagne bedacht.
Het uiteindelijk resultaat is goed lopend campagne management proces. Een voortdurende bewaking van de kwaliteit van selecties. Een effectieve verdeling van klanten onder acties van marketeers. Kortom, een instrument om op efficiënte wijze bestaande klanten te bewerken.
Kortom, in deze tweede fase zorgt het gebruik van data mining voor een sterke leercurve in de organisatie. Het meest duidelijk is de stijging in de effectiviteit van acties. Met als voorwaarde: een procedurele aanpassing van het selectie/analyse proces, dat het gebruik van score modellen waarborgt.
‘Derde generatie: contact mining, klant aan het stuur!’
Waar in de vorige fase vooral de marketeers en analisten de touwtjes in handen hadden, neemt de klant steeds vaker het roer over. Met de komst van nieuwe en de verdere ontwikkeling van bestaande interactieve media, zoals call-centers, voice-response-units en internet, ligt het contact initiatief meer en meer bij de klant. De tijd om klanten goed voorbereid te benaderen met een specifiek aanbod is er opeens niet meer. De klant of een prospect neemt met jou contact op. Het ultieme moment om te marketen is daar. Wat moet je doen?
Of het nu om een call-center gaat of om internet, het concept blijft hetzelfde. De bestaande kennis over de betreffende klant moet onmiddellijk leiden tot een commerciële suggestie op maat. Op zich geen probleem. Een selectie van de beste benadering op basis van een batterij scores lijkt een oplossing. Maar wat als de klant zijn beklag komt doen of een product bestelt, waardoor de voorgestelde benadering zinloos blijkt? De klant neemt het initiatief tot het contact en zal daarom eerst iets van jou verlangen. Deze informatie is essentieel in het bepalen van een eventuele commerciële benadering richting die klant. Voorberekende scores op basis van data mining modellen zijn niet toereikend. Het on-line scoren van data mining modellen met zowel bestaande als zojuist verkregen klantinformatie is derhalve een must.
Data mining in het productieproces stelt hoge eisen aan de voorspellingsmodellen. Deels om rekening te houden met de dynamiek, waarbij laatste wijzigingen leiden tot nieuwe uitkomsten. En deels vanwege de directe feedback op de gekozen benadering. Het verschil tussen actie en het meten van de reactie reduceert zich van enkele weken, of zelfs maanden, tot een enkele seconden. Dit vereist een snelle doorberekening van modellen en een zeer valide en robuuste voorspelling. In de praktijk betekent dit dat, bijvoorbeeld, een agent aan het call-center het advies krijgt om de klant een aanbieding te doen. Het model geeft een kans van slagen van 80% aan. De informatie over de klant wijzigt zich tijdens het gesprek en de scoringskansen worden tijdens het gesprek binnen enkele seconden opnieuw vastgesteld. Als de scoringskans van het betreffende aanbod zakt naar bvb. 20%, zal een ander aanbod met een hogere kans van slagen op het scherm van de agent verschijnen. Uiteindelijk bepaalt de agent altijd zelf of de geboden commerciële suggestie daadwerkelijk uitvoerbaar is.
Belangrijk voordeel van het inzetten van modellen in het interactieve productieproces is dat de communicatie met de klant verschuift van monoloog naar dialoog. Beschikt men in het klantcontact over de juiste informatie dan ontstaat er een effectieve dialoog met de klant. Hierbij krijgt de klant inzicht in het bedrijfsproces, en merkt het dat het hier invloed op heeft. Directe gevolg is een vergroting van de klanttevredenheid, en daarmee samenhangend, een duurzame relatie met de klant. Dus het inzetten van data mining in het productieproces stelt een bedrijf in staat nieuwe klanten te werven, langdurige, succesvolle relaties met klanten op te bouwen en deze te onderhouden.
Datamining, de huidige stand van zaken
Gert Haanstra en Melbert van Emmerik, CustomerBase Jaarboek 2000, F&G Publishing, Amersfoort, Januari 2000, blz 64 t/m 75
Printversie: Datamining de huidige stand van zaken
De mogelijkheden van datamining worden uitgebreider en interessanter. Enerzijds worden de tools beter en krijgen de mensen die ermee werken meer ervaring. Anderzijds wordt er meer data in databases opgeslagen door de enorme groei in de hedendaagse communicatie mogelijkheden, wat meer en meer informatie over klanten, klantcontacten en transacties genereert. Het gaat zelfs zover dat alle boodschappen in de supermarkt door middel van klantenkaarten geregistreerd kunnen worden. Hetzelfde geldt voor iedere klik die klanten op jouw internet site maken. De opslag van al deze informatie vormt niet meer het grootste probleem, De opslagsystemen worden steeds goedkoper en meer geavanceerd. Maar wat moet je met al die informatie? Door middel van datamining gaan steeds meer bedrijven zoeken naar mogelijkheden om de verkregen informatie te gebruiken in hun marketing beleid. Doel van dit stuk is het geven van een overzicht van de (on)mogelijkheden van datamining, afgezet tegen de ontwikkeling die datamining doormaakt.
Datamining in een breder verband
Datamining is de zoektocht naar belangrijke verbanden en patronen binnen een analysebestand. Het is een essentieel onderdeel van een veel groter proces: het Knowledge Discovery in Databases (KDD) proces. Dit laatste proces omvat het gehele proces van commerciële vraagstelling naar de uiteindelijke marketing activiteit die op specifieke groepen klanten wordt ondernomen.
Het KDD-proces begint met het selecteren van die gegevens uit de grote hoeveelheid data die relevant zijn voor het beantwoorden van de vraagstelling. Na de selectie van relevante gegevens vindt een data manipulatie slag plaats. Hierbij worden gegevens geschoond, consistent gemaakt en gecombineerd tot nieuwe informatie. Dit is met name in het beginstadium een bijzonder tijdrovende aangelegenheid. Welke gegevens zijn überhaupt binnen een organisatie aanwezig? Waar zijn alle gegevens opgeslagen? Vaak zijn er verschillende systemen of databases waardoor informatie op verschillende manieren is opgeslagen. Het koppelen van gegevens uit deze verschillende systemen kan dan problemen opleveren. Daarom is het streven dit manipulatieproces zo veel mogelijk te automatiseren. Waarbij veelal de gemanipuleerde data in datawarehouses wordt opgeslagen. Het automatisch opslaan van informatie in datawarehouses is dus bijzonder belangrijk. Daarmee krijgt de informatie over de tijd een consistent karakter (en wordt de kans op fouten kleiner) en is de informatie voor de dataminingfase direct toegankelijk. Vervolgens begint de zoektocht naar belangrijke verbanden en patronen binnen het analyse bestand. Deze dataminingfase resulteert uiteindelijk in de kennis omtrent de initiële vraagstelling.
De term datamining wordt vaak verward met KDD (Knowledge Discovery in Databases). Strikt genomen is het zoeken van relevante informatie uit databases specifiek gestuurd door de kennis en ervaring in het bedrijf geen onderdeel van datamining. Het creatieve proces van het selecteren en manipuleren van data om nieuwe afgeleide informatie te verkrijgen zou je databasemining kunnen noemen. Het resultaat is een analysebestand dat gebruikt wordt om verbanden en patronen binnen het analyse bestand te vinden. Het analyse pakket automatisch laten zoeken naar deze verbanden en patronen heet datamining. Zowel databasemining en datamining zijn onderdelen van het Knowledge Discovery in Databases proces. In dit artikel ligt de nadruk op de rol van datamining, hoewel het onoverkomelijk is om ook andere facetten van het KDD-proces de revue te laten passeren.
Ontwikkelingen in datamining
Pioniersfase
Datamining begint met het uitproberen van de mogelijkheden. Zo’n eerste stap heeft als functie om het bedrijf warm te maken voor datamining. Wat kan datamining voor het bedrijf betekenen? Levert het geld op? Zo ja, hoeveel? Welke investeringen gaan ermee gepaard? En op welke gebieden vereist het organisatorische aanpassingen? Bedrijven begrijpen dat klantinformatie geld oplevert, en proberen met behulp van datamining de benodigde informatie uit de databases te krijgen. Enerzijds om de effectiviteit van de marketing inspanningen te vergroten. Anderzijds om nieuwe verkoopmogelijkheden te ontdekken in het klantenbestand. Een eerste grote succes prikkelt het bedrijf om aandacht te geven aan de mogelijkheden.
Er wordt het een en ander uitgeprobeerd met de gegevens uit bestaande databases: een grote analyse, het bouwen van een scoremodel, het vinden van nieuwe klantsegmenten. Bij een gedegen teststrategie en voldoende aandacht voor de creatie van afgeleide voorspellende klantkenmerken kan het succes van datamining niet uitblijven. In dit stadium staan alle stadia in het KDD-proces centraal. In de ideale situatie werken verschillende disciplines samen om hun eerste resultaten te behalen. IT-specialisten om ruwe data uit de diverse transactie bestanden beschikbaar te stellen. Marketeers en/of domein deskundigen om input te geven aan de bruikbaarheid van de klantinformatie. En analisten voor het bewerken van de data en het ontwikkelen van een voorspellingsmodel. Duidelijk is dat datamining in deze eerste fase vanaf de zijlijn meedoet aan het verkoopproces. Bij aantoonbaar succes gaat de deur open voor een structurele rol in het marketing & verkoop proces. Vaak wordt datamining voor verschillende toepassingen in een soort proeftuin setting uitgeprobeerd om een breder draagvlak te creëren om de vaak hoge investeringskosten het hoofd te kunnen bieden. De eerste fasen van het KDD-proces worden vervolgens voor een groot deel door IT-specialisten geautomatiseerd door de bouw van datawarehouses. Het datawarehouse bevat alle relevante afgeleide data die gebruikt worden in datamining. Wanneer het datawarehouse volledig geimplementeerd is zal de nadruk steeds meer op datamining komen te liggen.
Productiefase
Komt er een vervolg na de ‘pioniersfase’, dan staat in de tweede fase de integratie van datamining in het marketing- & verkoopproces centraal. Enerzijds om datamining consequent te gebruiken en maximaal te profiteren van de opbrengsten. Anderzijds om ontwikkelde voorspellingsmodellen continu te evalueren en verder aan te scherpen. De integratie moet uiteindelijk leiden tot structurele verbeteringen in de actieresultaten.
Het streven is continuïteit. Continuïteit in het verbeteren van de kwaliteit van de modellen. Continuïteit in het creëren van meer modellen voor nieuwe doelgroepen en voor andere producten. En continuïteit in het binnenhalen van meer omzet. Ofwel, continuïteit in het optimaliseren van het commerciële proces.
In de praktijk betekent dit het opzetten van testcycli om voortdurend de juiste mix van klanten, producten, kanalen en timing te achterhalen. Verschillende acties worden specifiek opgezet om nieuwe modellen te bouwen. Daarnaast worden bestaande modellen aangescherpt door ze te gebruiken en tegen oude modellen te testen. Uiteindelijk leiden deze cycli tot een set kwalitatief goede voorspellingsmodellen voor de meeste ‘standaard’ acties.
Voorheen gaven analisten voornamelijk vrijblijvend advies aan marketeers over de te benaderen klantgroepen. Met een integratie van datamining, ontstaat een duidelijke verantwoordelijkheid in het continu vinden van nieuwe, beter scorende doelgroepen in de database. Een discipline die in eerste instantie ligt bij analisten, maar die een sterke samenwerking met data specialisten en marketeers vereist voor maximaal succes. Op basis van het profiel van gevonden klantgroepen wordt tezamen met de marketeers een geschikte campagne bedacht.
Het zoeken van een goede combinatie tussen enerzijds campagne management systemen en anderzijds geavanceerde datamining tools is derhalve een pre. Centraal hierin staat een datawarehouse waarbij alle relevante informatie over klanten is verzameld. Het datawarehouse biedt zowel de informatie die nodig is om datamining toe te passen als de informatie die nodig is om klanten voor verschillende campagnes te selecteren. Afhankelijk van kanaalvoorkeuren, recente communicatie uitingen, specifieke klantinformatie en de scores op verschillende scoremodellen worden klanten voor campagnes geselecteerd.
Vereiste is dat de informatie in het datawarehouse zeer up-to-date en betrouwbaar is. Bij voorkeur worden de scores voor de verschillende voorspellingsmodellen berekend op het moment van selectie. Dit houdt in dat het campagne management niet voorberekende scores voor verschillende campagnes gebruikt, maar dat de scores dynamisch beschikbaar zijn. Het campagne management systeem roept het model aan, berekend de scores en wijst vervolgens klanten toe aan verschillende campagnes.
Het uiteindelijk resultaat is goed lopend contact management proces. Een voortdurende bewaking van de kwaliteit van selecties. Een effectieve verdeling van klanten onder acties van marketeers. Kortom, een instrument om op efficiënte wijze bestaande klanten te bewerken.
In deze tweede fase zorgt het gebruik van datamining voor een sterke leercurve in de organisatie. Het meest duidelijk is de stijging in de effectiviteit van acties. Met als voorwaarde: een procedurele aanpassing van het selectie/analyse proces, dat het gebruik van score modellen waarborgt. De invalshoek in deze fase is nog sterk gericht op outbound marketing activiteiten. Door de opkomst van meer interactieve media en ook de groeiende behoeften van klanten om zelf het initiatief te nemen om contact te zoeken met bedrijven via deze kanalen, moeten toepassingen van datamining verder uitgebreid worden om ook de meer interactieve contact momenten te benutten.
Interactiefase
Waar in de vorige fase vooral de marketeers en analisten de touwtjes in handen hadden, neemt de klant steeds vaker het roer over. Met de komst van nieuwe en de verdere ontwikkeling van bestaande interactieve media, zoals call-centers, voice-response-units en internet, ligt het contact initiatief meer en meer bij de klant. De tijd om klanten goed voorbereid te benaderen met een specifiek aanbod is er opeens niet meer. De klant of een prospect neemt met jou contact op. Het ultieme moment om te marketen is daar. Wat moet je doen?
Of het nu om een call-center gaat of om internet, het concept blijft hetzelfde. De bestaande kennis over de betreffende klant moet onmiddellijk leiden tot een commerciële suggestie op maat. Op zich geen probleem. Een selectie van de beste benadering op basis van een batterij scores lijkt een oplossing. Maar wat als de klant zijn beklag komt doen of een product bestelt, waardoor de voorgestelde benadering zinloos blijkt? De klant neemt het initiatief tot het contact en zal daarom eerst iets van jou verlangen. Deze informatie is essentieel in het bepalen van een eventuele commerciële benadering richting die klant. Voorberekende scores op basis van datamining modellen zijn niet toereikend. Het online scoren van datamining modellen met zowel bestaande als zojuist verkregen klantinformatie is derhalve een must.
Datamining in het productieproces stelt hoge eisen aan de voorspellingsmodellen. Deels om rekening te houden met de dynamiek, waarbij laatste wijzigingen leiden tot nieuwe uitkomsten. En deels vanwege de directe feedback op de gekozen benadering. Het verschil tussen actie en het meten van de reactie reduceert zich van enkele weken, of zelfs maanden, tot een enkele seconden. Dit vereist een snelle doorberekening van modellen en een zeer valide en robuuste voorspelling. In de praktijk betekent dit dat, bijvoorbeeld, een agent aan het call-center het advies krijgt om de klant een aanbieding te doen. Het model geeft een kans van slagen van 80% aan. De informatie over de klant wijzigt zich tijdens het gesprek en de scoringskansen worden tijdens het gesprek binnen enkele seconden opnieuw vastgesteld. Als de scoringskans van het betreffende aanbod zakt naar bvb. 20%, zal een ander aanbod met een hogere kans van slagen op het scherm van de agent verschijnen. Uiteindelijk bepaalt de agent altijd zelf of de geboden commerciële suggestie daadwerkelijk uitvoerbaar is.
Belangrijk voordeel van het inzetten van modellen in het interactieve productieproces is dat de communicatie met de klant verschuift van monoloog naar dialoog. Beschikt men in het klantcontact over de juiste informatie dan ontstaat er een effectieve dialoog met de klant. Hierbij krijgt de klant inzicht in het bedrijfsproces, en merkt het dat het hier invloed op heeft. Directe gevolg is een vergroting van de klanttevredenheid, en daarmee samenhangend, een duurzame relatie met de klant. Dus het inzetten van datamining in het productieproces stelt een bedrijf in staat nieuwe klanten te werven, langdurige, succesvolle relaties met klanten op te bouwen en deze te onderhouden.
Een stap richting Customer Relationship Management
Duidelijk is dat de huidige datamining technologie klaar is om ingezet te worden in het versterken en het verdiepen van de individuele relatie met de klant. Ofwel, datamining kan worden gebruikt in het Customer Relationship Management (CRM) concept. Centraal in dit concept is de interactie met de klant tijdens een contactmoment. De klantwaarde kan worden verhoogd en de loyaliteit worden vergroot door in te gaan op de eigen wensen en behoeften van iedere klant. Juist in klantcontacten ondersteunt datamining het beslissingsproces door gebruik te maken van de kennis over de klant.
Op basis van specifieke klantkenmerken vindt een differentiatie van klanten plaats op basis van gedrag, kanaalvoorkeur en specifieke wensen en behoeften. Met name in dit traject speelt datamining een kritische rol. Op het moment van interactie speelt differentiatie een grote rol. Op welke wijze moeten bepaalde klanten benaderd worden, kan ik een aanbod doen en zo ja welk aanbod en wat zijn goede argumenten om bij die specifieke klant te gebruiken? Datamining kan worden ingezet om de effectiviteit van de differentiatie en interactie te evalueren om het vervolgens te optimaliseren. In dit hoofdstuk gaan we in op de eisen voor een succesvolle implementatie van datamining in CRM.
Voor de implementatie van datamining binnen customer relationship management is het belangrijk om een onderscheid te maken tussen inbound en outbound contacten. Bij outbound contacten (mail, leads, calls) vindt het toewijzen van klanten aan verschillende campagnes plaats in batch processen. Een campagne management systeem of een scheduler met verschillende query jobs heeft bijvoorbeeld de gehele nacht de tijd om klanten te selecteren op basis van specifieke klantkenmerken. Bij inbound contacts is die tijd er niet meer. Wil je klanten gedifferentieerd aanspreken, dan is het noodzakelijk om alle relevante klantinformatie up-to-moment paraat te hebben. Sterker nog, de nieuw informatie die gedurende de interactie tot stand komt, kan zeer essentieel zijn in het vervolg van de interactie. Door middel van online scoring van voorspellingsmodellen wordt nog tijdens het gesprek de vervolgstap bepaald. Dit betekent dat binnen enkele seconden data verwerkt moet zijn en dat scores op verschillende modellen doorgerekend moet worden.
De meeste bedrijven hebben operationele systemen en datawarehouses op gescheiden platformen geïnstalleerd. De reden hiervoor is dat een datawarehouse primair gebruikt wordt voor het klaarzetten van ruwe (geschoonde en consistente), afgeleide en gesommeerde data voor analyses en rapportages. Door deze informatie op een afzonderlijk platform beschikbaar te stellen, is er geen gevaar voor overbelasting van operationele systemen. Onder andere doordat up-dates van datawarehouses periek in batch processing liep. Tegenwoordig is er een grote behoefte aan up-to-moment klantinformatie. Door het meer interactieve karakter van online klantcontacten bij call-centers of op internet, moeten bedrijven zowel beschikken over up-to-date informatie als over zoveel mogelijk afgeleide klantinformatie als over de meest geschikte actie voor dat moment. Dit heeft belangrijke consequenties voor het onderhoud van datawarehouses, aangezien datawarehouses eveneens up-to-date moeten zijn.
Welke eisen het gebruik van datamining in CRM omgeving stelt aan de infrastructuur, laten we zien in een voorbeeld. Een voorspeller in een specifiek model kan ‘het aantal stortingen over de laatste maand’ zijn. Dit kenmerk is verkregen door het aantal stortingen in het gegeven tijdinterval te tellen in de transactiesystemen. De storting moet eerst in het transactiesysteem worden verwerkt. Vervolgens moet het datawarehouse gemuteerd worden op basis van de nieuwe informatie. Daarna worden opnieuw de scoremodellen doorlopen, voordat we weten of de mutatie van invloed is op het aanbod dat we online willen gaan doen. Het zal duidelijk zijn dat dit geen haalbare kaart is tijdens hetzelfde telefoongesprek van een call center agent met een klant. Een mogelijkheid is om genoegen te nemen met informatie die een dag oud is. Wanneer een call-center agent een melding krijgt dat een bepaald product geschikt is voor die klant, dan zal die agent afhankelijk van het gesprek bepalen of de voorgestelde suggestie nog hout snijdt. Een andere optie is om voor interactieve toepassingen alleen gebruik te maken van scoremodellen die minder dynamische of kritische klantkenmerken bevatten. Een derde optie is om gegevens via triggers in de database af te tappen waardoor een snellere herberekening van scoremodellen mogelijk is.
De noodzakelijke functionaliteiten
Om CRM volledig en goed te kunnen uitvoeren, zijn een aantal tools nodig. In een z.g. contact management systeem (CMS) is campagne management naadloos geïntegreerd met datamining en online scoring.
Ten behoeve van de ontwikkeling van score modellen kan een datamining tool niet meer bestaan zonder KDD functionaliteiten als het toegankelijk maken van databases en verregaande datamanipulatie mogelijkheden. Belangrijk zijn de volgende onderdelen:
• ‘data access’ voor het flexibel en dynamisch verkrijgen van gegevens uit het datawarehouse,
• ‘data preparatie’ voor het bewerken, aggregeren en mergen van gegevens uit verschillende tabellen,
• ‘behavioural scoring’ voor de ontwikkeling van score modellen,
• ‘export’ van model definities in het datawarehouse voor gebruik door het contact management systeem.
Vervolgens zijn de volgende campagne management functionaliteiten gewenst:
• ‘event triggering’ om belangrijke events te herkennen en daar met campagnes op in te springen,
• ‘targeting systeem’ om te beslissen welke campagnes het beste passen bij welke klanten,
• ‘scheduler’ om de selecties van verschillende campagnes in de tijd te automatiseren,
• ‘channel management’ voor distributie en coördinatie van verschillende , multi-channel campagnes,
• ‘decision support’ om prioritieten te stellen aan het gebruik van score modellen en het gebruik van de verschillende distributie kanalen,
• ‘reporting’ om enerzijds vooraf de (financiële) consequenties van campagnes te bepalen en om anderzijds de campagnes te monitoren.
In een multi-channel situatie spelen vele aspecten een rol, waaronder:
• Directheid (b.v. mailings, huis-aan-huis inserts, coupons),
• Differentiatie (b.v. campagne A voor klant X en campagne B voor klant Z),
• Persoonlijk (b.v. franchise, tussenpersonen, call-centers, gepersonaliseerde internetpagina’s),
• Massaal (b.v. radio/tv-advertenties die specifieke doelgroepen aanspreken, nieuwsbladen),
• Electronisch (b.v. telefoon, voice-response, sms, internet, email),
• Interactief (b.v. internet, call-center).
Geschikte analyse technieken
De gebruikelijke analyse technieken worden bekeken aan de hand van de nieuwe eisen die vanuit CRM aan datamining techieken worden gesteld. Met datamining tools moet het o.a. mogelijk zijn om toekomstige trends en toekomstig klantgedrag te voorspellen. Waarbij het mogelijk moet zijn om pro-actieve en kennisgedreven beslissingen te ondersteunen. Een belangrijk en vaak veronachtzaamd criterium voor de keuze van de tools of technieken is de interpreteerbaarheid van de segmenten en het gemaakte model. Naast de kwaliteit van de voorspelling (b.v. het aantal keren dat de voorspelling ook daadwerkelijk uitkomt), moet duidelijk zijn wie voor welk aanbod geschikt is. En vooral de reden waarom iemand het aanbod krijgt. De meeste leveranciers van datamining tools geven aan de interpreteerbaarheid van hun oplossingen/technieken hoog in het vaandel te hebben.
De combinatie van verschillende meer traditionele statistische methoden en nieuw verkregen inzichten hebben tot een grote diversiteit aan datamining technieken en algoritmen geleid. Veelal staan datamining leveranciers in nauw contact met verschillende universitaire onderzoekscentra om snel nieuwe algoritmen in hun datamining oplossingen te integreren. In het verleden leidde dit op een gegeven moment tot steeds minder inzichtelijke methoden, omdat voortdurend op zoek ging naar de best voorspellende datamining technieken. Tegenwoordig is er het besef dat voor marketing toepassingen de interpreteerbaarheid van datamining uitkomsten zeer relevant is. Je wilt niet alleen weten welke campagnes het beste aansluiten bij welke klanten, maar je bent ook geïnteresseerd in de onderliggende redenen. Met name bij de meer marketing gerichte leveranciers is inmiddels de trend om een hoge prioriteit toe te kennen aan de interpreteerbaarheid bij een aanbieden van datamining toepassingen. Enkele technieken die door veel datamining tools ondersteund worden, zullen op basis van deze criteria worden beschreven.
Regressie analyse
Meest traditioneel zijn de regressie technieken. Het meest inzichtelijke is de multipele regressie waarbij een lineaire functie een schatting geeft van hetgeen je wilt voorspellen, bijvoorbeeld respons op een mailing. Daarnaast wordt ook wel logistische regressie gebruikt wanneer de te voorspellen variabele, zoals respons, slechts twee uitkomsten omvat (ja/nee). Logistische regressie is wat moeilijker interpreteerbaar, maar stelt minder hoge eisen aan de kwaliteit van de data.
Decision trees, decision lists of decision rules
Waarschijnlijk de techniek met de meest inzichtelijke resultaten. Bij beslissingstechnieken wordt niet zozeer een voorspelling gedaan, maar wordt de dataset in segmenten opgesplitst. Deze segmenten worden expliciet beschreven aan de hand van de kenmerken van de klant(groep). Elk segment heeft een bepaalde gemiddelde score, bijvoorbeeld gemiddeld respons. De vergelijking van de score tussen de segmenten resulteert in uitspraken over de meest kansrijke segmenten voor een bepaalde actie. Bij decision trees wordt het gehele analysebestand in segmenten opgesplitst. Bij decision rules worden de beste segmenten uit de dataset geïdentificeerd. Tussen de verschillende segmenten kan overlap ontstaan. Overlap is uitgesloten bij decision lists aangezien hier stapsgewijs naar het meest kansrijke segment wordt gezocht. Eenmaal een segment gevonden dan worden de klanten in dat segment uitgesloten bij de zoektocht naar het volgende meest kansrijke segment. Beslissingstechnieken spreken marketeers bijzonder aan, aangezien het resultaat een serie klantprofielen is met een bijbehorende score. Bijvoorbeeld, mannen onder 25 jaar met een lease auto hebben een schaderisico van, laten we zeggen, 45% op jaarbasis.
K-nearest neighbour
De K-nearest neighbour gaat uit van de gedachte dat klanten die op elkaar lijken hetzelfde gedrag vertonen. Wanneer je wilt voorspellen hoe iemand zich zal gaan gedragen, kijken we naar het K-aantal (b.v. 10) klanten dat het dichtst bij hem in de dataspace zitten, met andere woorden het meest dezelfde kenmerken bezitten. Naarmate meer van zijn buren bepaald gedrag vertonen, zal het waarschijnlijker zijn dat de klant in kwestie ook dat gedrag gaat vertonen.
Neurale netwerken
De verschillende varianten van neurale netwerken zijn geïnspireerd op het menselijk brein dat een onnoemelijk hoeveelheid neuronen bevat die allemaal tot elkaar in verbinding staan via synapsen. Het basisprincipe komt erop neer dat een neuraal netwerk eveneens neuronen (nodes) die alle mogelijke klantkenmerken weergeven (input nodes). Via een aantal tussenliggende lagen staan deze input nodes in verbinding met de output nodes (hetgeen je wilt voorspellen). De tussenliggende lagen bestaan uit een groot aantal lineaire functies waarbij input nodes via varierende gewichten de output nodes voorspellen. Gedurende het trainen worden de gewichten en de functies steeds aangepast en wordt het effect van het resultaat gemeten in termen van verschillen tussen voorspelde en werkelijk uitkomst. Het grote probleem bij neurale netwerken is dat het een ondoorzichtige black-box is, dat wel oplossingen verstrekt, maar niet de totstandkoming van de oplossing. Bovendien is het minder geschikt voor een dynamische omgeving omdat het netwerk hiervoor opnieuw getraind moet worden.
Genetische algoritmen
Genetische algoritmen zijn geïnspireerd door de evolutie theorie, waarbij oplossingen in verschillende stadia geoptimaliseerd worden. In eerste instantie worden een aantal ruwe oplossingen geboden. Het algoritme gaat verder met de beste oplossingen en maakt vervolgens combinaties van de verschillende oplossingen. Delen van de ene oplossing worden gebruikt in andere oplossingen en andersom. Dit proces herhaalt zich waarbij verschillende generaties oplossingen ontstaan totdat er uiteindelijk een bevredigde oplossing uitrolt. Het proces van voortborduren op eerdere oplossingen maakt het herleiden van het eindresultaat naar de oorspronkelijke klantkenmerken moeilijk. Hetgeen de interpreteerbaarheid van de uiteindelijke oplossing naar klantkenmerken uiteindelijk niet ten goede komt.
Association rule
Association rules zijn altijd gebaseerd op binaire kenmerken (ja/nee). Er wordt gezocht naar IF-THEN regels. Een uitkomst is bijvoorbeeld; als geslacht is ‘man’ en aankoop is ‘luiers’, dan 50% koopt eveneens bier. Zo kun je oneindig veel associaties maken, maar uiteraard ben je geïnteresseerd in de regels die een voldoende groot deel van het klantbestand identificeren en die een hoge uitkomst opleveren. Resultaten zijn makkelijk en eenduidig te interpreteren.
Conclusie
Datamining maakt een snelle ontwikkeling door. Echter, slechts weinig bedrijven weten deze mogelijkheden volledig te benutten. Bij een aantal bedrijven zijn marketing datawarehouses inmiddels operationeel, al dan niet na een lange lijdensweg. Nu is het stadium bereikt waarin de aandacht uitgaat naar de inrichting van het campagne management proces inclusief het effectieve gebruik van scoremodellen. Ondertussen gaat de ontwikkeling van datamining steeds verder. Een volledige integratie in het customer relationship management concept is inmiddels mogelijk. Meer directe kanalen, meer interactiviteit en meer initiatief van de klant. Denken bedrijven dat ze er zijn met een goed lopend campagne management systeem en een volwaardig datawarehouse, dan komen ze bedrogen uit. Want hoe kun je interactieve contactmomenten commercieel uitbuiten wanneer klanten zelf contact zoeken? Wanneer klanten een call-center bellen, zijn zij bereid tot interactie. Wanneer klanten de internetsite bezoeken staan ze open voor informatie. Dit zijn bij uitstek de momenten om de relatie met de klant te verstevigen.
Veel bedrijven zijn nu in staat om gedifferentieerde outbound marketing activiteiten te ontplooien. De toekomst ligt in de inbound contactmomenten. Inbound contactmomenten komen bij uitstek tot stand via call-centers, via email en via internet. Call-center agents moeten derhalve uitgerust worden met informatie om deze moment optimaal te benutten. De uitdaging hierbij ligt in de snelheid waarmee de informatie uit het gesprek wordt verwerkt in de score van de klant (en de aanbieding die deze klant krijgt). Bij internet ligt de uitdaging meer in de grote hoeveelheid gedragsinformatie afkomstig van het internet bezoek. Wat doen individuele klanten op de site en in hoeverre zijn wij in staat dat te beïnvloeden en commercieel uit te buiten?
De uitdaging voor de toekomst ligt initieel op een betere differentiatie van klanten op basis van de huidige kennis van klanten en op de ontwikkeling van meer gedifferentieerde klantconcepten. Daarnaast komt weer nieuwe informatie vrij over interactieve contactmomenten met klanten. Deze informatie overstijgt, wat volume betreft, waarschijnlijk zeer snel de huidige informatie over onze klanten. Het intelligent omgaan met deze nieuwe vloedgolf aan klantinformatie in het contact met klanten zal een hot issue worden. Welke informatie is relevant? Welke informatie is vervolgens af te leiden? En hoe kan die informatie worden gebruikt om de interactie met klanten te faciliteren om zodoende de customer relationship optimaal te managen.
Webmining; datamining op webgegevens
Gert Haanstra, Mnet, media voor electronisch ondernemen, ten Hagen & Stam, Den Haag, Nr. 11, 2000
Printversie: Webmining
De druk om via internet sites daadwerkelijk omzet te genereren neemt toe. Duidelijk is dat een aantal grote sites hun investeringen willen terug verdienen met behulp van bewezen technieken uit de meer traditionele economie. Bijvoorbeeld, zoals het verhogen van de bekendheid van de site met behulp van tv reclame, of het verhogen van de loyaliteit van de gebruiker met behulp van Customer Relationship Management (CRM). Data mining is ook zo’n bewezen techniek. Mits voldaan aan een aantal belangrijke voorwaarden, geeft het bedrijven met internetsites de mogelijkheid om op een effectieve manier geld te verdienen met de informatie over bestaande klanten.
Succes met web mining
Wat is web mining? Web mining is data mining specifiek op web gegevens. Hierbij gaat het om het benutten van de klantinformatie in de database. De klantgegevens worden geanalyseerd, om vervolgens te worden ingezet bij een volgende actie. Het analyseren gebeurt met allerlei technieken. Vaak is het resultaat een overzicht van bijvoorbeel wie de producten recentelijk heeft gekocht. Een stap verder is het maken van een scoremodel waarbij een voorspelling wordt gedaan over wie een grote(re) kans heeft om het product een volgende keer te kopen.
Verkoop genereer je door de klant die de site bezoekt, een aanbieding te doen. Cruciaal is het doen van een juiste aanbieding, dat wil zeggen een aanbieding ‘waar de bezoeker op zit te wachten’. Daarvoor moet op moment dat een klant de site bezoekt, in de database worden gekeken of er wel een aanbod voor deze klant is. En zoja, wel aanbod interessant is voor deze klant. Op de achtergrond wordt, specifiek voor deze klant, allerlei scoremodellen uitgerekend (=’real time scoring’). Vervolgens wordt het meest kansrijke aanbod geselecteerd en klaargezet om te worden gepresenteerd in een pagina van de site. Waarna het aanbod kan worden verwerkt in een banner, grafiek, pagina, etc. Dit alles gebeurt op het moment dat de klant aanwezig is op de site.
Web mining is succesvol als er, enerzijds, juist wordt voorspeld. Anderzijds als het opgeleverde model ook daadwerkelijk vaak wordt gebruikt. Met name het laatste wordt vaak vergeten en/of verkeerd begrepen. Bedrijven vergeten vaak dat de uitkomst van het model moet leiden tot een concrete actie richting de klant. Dit kan een specifieke pagina zijn die moet worden gepresenteerd op het moment dat de klant de site bezoekt, maar ook een suggestie naar de call center agent om een bepaald product te sprake te brengen. Om een duidelijke richting te kunnen geven aan de vervolg actie, moet het de eindgebruiker (bijvoorbeeld marketeer of call center agent) duidelijk ‘wat er moet gebeuren’ en ‘waarom dit beter is dan de huidige manier van werken’. Pas als hij hiervan overtuigd is, zal de eindgebruiker bereid zijn om te doen wat het model van hem vraagt, nl. de klant een specifiek product verkopen. Het spreekt voor zich dat deze manier van werken hoge eisen stelt aan hoe het model moet worden opgebouwd, en wat er met het resultaat moet worden gedaan.
Wat is er nodig voor web mining?
Zonder een database en een analysetool zal er weinig resultaat worden behaald. De database met klantgegevens is nodig om te achterhalen welke de kenmerken zijn van de mensen die het product kopen. Belangrijk verschil met traditionele data mining is dat er bij web mining sprake is van relatief weinig klanten, die regelmatig terugkomen naar de site. Ofwel, een kleine database met vaak dezelfde soort gegevens. Waarbij in een ideale situatie de internet database wordt gekoppeld aan reeds bestaande databases waarin meer gegevens zitten die over een langere periode zijn vast gehouden. Het beeld van de klant wordt hiermee vollediger, en de gevonden klantgroepen diverser.
Welke gegevens zijn (mogelijk) interessant voor een voorspelling: Welke pagina’s zijn er bezocht, welke functies zijn aangeroepen, welke links werden er gebruikt, op welke banners is er geklikt? Of, hoe ziet het browse patroon eruit en wat is zijn click gedrag? Hoe vaak is de site bezocht, wanneer, hoelang, wanneer kwam men binnen en ging men weer weg? Naast gedragingen kunnen voorkeuren worden vastgelegd. Met het gebruik van dynamische pagina’s is het zelfs mogelijk om ontbrekende gegevens die nodig zijn voor een goede voorspelling, aan de bezoeker zelf te vragen tijdens het bezoek. Bijvoorbeeld, voor het wel/niet aanbieden van een interessante vakantiereis, kan men vragen of men binnen een maand op vakantie wil gaan?
De web mining technieken zijn te verdelen in de meer generieke technieken (bijvoorbeeld affinity, induction, collaborative filtering), en de pure one-to-one technieken (bijvoorbeeld decision lists, association rules). Belangrijkste verschil is dat de generieke technieken de te verkopen producten centraal stellen, en dat de one-to-one technieken uitgaan van de volledige klantrelatie (waaronder de mogelijkheid van cross selling). Daarnaast baseren de generieke technieken hun voorspellingen op een algemeen klantbeeld, en geschikt is voor anonieme bezoekers van een site. One-to-one technieken gaan echter uit van de combinatie van individuele kenmerken die horen bij de klanten die het product hebben gekocht. Voorwaarde is dat de klant geïdentificeerd wordt.
Duidelijk is dat het gebruik van web mining impliciet een keuze betekent tussen het verbeteren van de directe verkoop en het verdiepen van de klantrelatie voor het genereren van (toekomstige) verkoop. Het laatste vraagt om een meer persoonlijke benadering waarvoor een uitgebreid klantbeeld nodig is, die veelal afkomstig is uit diverse databases (met historische gegevens). Bovendien zijn hiervoor andere technieken nodig om de gegevens te kunnen analyseren.
Noodzaak van een persoonlijke aanbieding
Hoe persoonlijker de aanbieding richting de klant, hoe effectiever de beïnvloeding. Zeker voor het web geldt dat er weinig gelegenheid is om de klant te sturen. Immers, er is veel afleiding en er zijn voldoende alternatieven. Een bezoeker zal in korte tijd oppervlakkig waarnemen of de pagina interessant is om te bekijken en verder te lezen. Vervolgens zal hij weinig moeite willen doen om de informatie volledig te begrijpen. Het betekent voortdurend een gevecht om de aandacht van de bezoeker vast te houden. Door de aanbieding persoonlijker te maken, lukt het om aandacht van de bezoeker langer vast te houden, en hem in een bepaalde richting te sturen.
Voor het gebruik van web mining betekent dit een vergaande differentiatie in het doen van een voorspelling. Op individueel niveau moet een suggestie worden gedaan over welk product aan te bieden. Daarnaast moet duidelijk zijn in welke woorden de suggestie wordt ‘verpakt’ en die de bezoeker prettig vindt. Verder zal de inhoud van de boodschap gebaseerd zijn op het model waarin wordt verwezen naar het gedrag en/of de interesses van de bezoeker. En verder wordt het bij voorkeur gepresenteerd in een lay-out die de bezoeker bevalt. Overigens, zonder de indruk te wekken dat de schrijver onbetrouwbaar is en/of niet geloofwaardig is.
Een persoonlijke benadering houdt ook in dat de klant kan kiezen met welk distributiekanaal hij zaken wil doen. Het is mogelijk dat de klant via het web nog te weinig uitleg kan krijgen op zijn vragen, of onvoldoende mogelijkheden heeft om zijn persoonlijke voorkeuren duidelijk te maken. In dat geval zou hij moeten kunnen ‘uitwijken’ naar bijvoorbeeld een call center. Veel grote dotcom bedrijven hebben naast hun sites ook een uitgebreid call center om hun klanten deze mogelijkheid te geven.
Het gevaar van deze ‘multi-channel aanpak’ is dat de boodschap richting de klant onnauwkeurig wordt en niet meer consistent is. In korte tijd worden verschillende aanbiedingen gedaan (bijvoorbeeld via een web pagina en later via de call center agent) die elkaar niet mogen tegenspreken. In tegendeel, ze zouden moeten voortborduren op de informatie die een klant in een eerder stadium heeft gegeven. Voor het gebruik van web mining betekent dit dat de voorspelling gebruik maakt van de klanthistorie opgedaan in een eerder, recent klantcontact. Daarnaast zou het flexibel moet zijn om direct feedback te geven op de belangrijkste bezwaren van de klant, zodat de kans op succes daadwerkelijk wordt verhoogd.
Conclusie
Tot slot, web mining is geschikt voor het signaleren en benutten van inbound klantcontacten via het web. Met behulp van analyses worden modellen gemaakt die kunnen worden gebruikt om de klant een specifiek aanbod te doen. Het suggereert ingewikkeldheid. Prettig, omdat het interesse oproept en respect afdwingt. Maar het succes van web mining wordt voor een belangrijk deel bepaald door hoe de modellen worden ingezet. Impliciet betekent het een keuze tussen het verhogen van de directe verkoop of het verbeteren van de klantrelatie voor het verkrijgen van (toekomstige) verkoop. In het laatste geval, staat de klant centraal en is er de noodzaak van real time scoring over meerdere distributiekanalen. De klant wordt op intelligente manier, persoonlijk aangesproken, rekening houdend met zijn persoonlijke voorkeuren en individuele historie. Vandaar dat personalized web mining waardevolle kansen biedt en zeer winstgevend is gebleken.
Klantkennis vanuit KennisManagement-perspectief
Gert Haanstra en Marcel Holsheimer, KM, Vakblad voor Kennismanagement, F&G Publishing, Amersfoort, Nr. 7/8, 2000, blz 23 t/m 25
Printversie: Klantkennis vanuit KM
Bedrijven worden geconfronteerd met een groeiende hoeveelheid mogelijkheden om kennis over de individuele klant te benutten. De druk om met deze kennis concreet commerciële resultaten te behalen stijgt. Geen wonder dat deze bedrijven zoeken naar manieren om effectief en efficiënt gebruik te maken van de beschikbare klantkennis en hiermee een blijvend concurrentievoordeel te behalen. De combinatie ‘data mining’ en ‘multichannel personalisatie’ lijkt hiervoor zeer geschikt.
Via data mining kunnen die klantcontacten worden gesignaleerd, die een goede kans bieden een (extra) product te verkopen. Multichannel personalisatie zorgt er vervolgens voor dat de gegevens over die veelbelovende klanten op de juiste plaats, op het juiste moment, in de juiste vorm en kwaliteit beschikbaar en toegankelijk zijn. Vanuit het perspectief van kennismanagement (KM) moet een aantal vragen worden beantwoord om van de combinatie data mining en multichannel personalisatie daadwerkelijk een commercieel succes te maken:
• Wat is ervoor nodig om de klantkennis te ontwikkelen op een manier dat deze directe toegevoegde waarde heeft voor de onderneming?
• Wat moet een bedrijf doen om klantkennis toe te passen en te verspreiden?
• Wat verandert er in de diverse bedrijfsprocessen, in de organisatie, en in de ICT-systemen en infrastructuur als men klantkennis commercieel wil benutten?
KM belangrijker door opkomst interactie
De opkomst van interactieve distributiekanalen als call center, internet, SMS en WAP, geeft bedrijven steeds meer mogelijkheden hun kennis over hun klanten te benutten om hun service te verbeteren. Deze nieuwe kanalen zijn inmiddels door veel klanten omarmd. Dat blijkt bijvoorbeeld uit de sterke groei in de afgelopen jaren van het aantal inbound contacten (inbound: het contact wordt door de klant gelegd) via deze kanalen. Hiermee lijkt een aloude klantwens te worden vervuld om anywhere en anytime in contact te komen met een bedrijf.
Interactieve kanalen zijn niet alleen in opkomst, ze worden ook steeds relevanter voor het zakendoen. De aandacht van de klant wordt steeds schaarser, dus wordt het steeds belangrijker om interactiemogelijkheden die vanuit de klant worden geïnitieerd te benutten. We zien bijvoorbeeld de effectiviteit van het ‘oude’ campaign marketing (outbound, dus vanuit het bedrijf) al een aantal jaren dalen. Eigen initiatieven van een marketeer om met klanten in contact te komen – zoals het uitsturen van een mailing of ongevraagd bellen – halen steeds minder omzet binnen. Het relatief belang van inbound klantcontacten neemt dus toe. Maar dat houdt ook in dat op het moment dat de klant contact zoekt met een bedrijf, het bedrijf er klaar voor moet zijn: het moet alle gegevens bij de hand hebben, moet snel relevante informatie kunnen vinden en moet ook direct kunnen reageren op aanvullende informatie die tijdens het klantcontact bekend wordt. Dan kan het bedrijf niet alleen reactie geven op de vraag van een klant, maar die klant ook proactief een aanbod doen en op die manier niet alleen de omzet maar ook het serviceniveau naar de klant verhogen.
Goed management van klantkennis is een van de basisvoorwaarden om dit te realiseren. Daarbij verstaan we onder kennismanagement: de manier waarop de juiste kennis op de juiste plaats, op het juiste moment, in de juiste vorm en kwaliteit aanwezig is.
Commerciële waarde klantkennis
De eerste vraag die aan het begin van dit artikel werd genoemd is: Wat is ervoor nodig om klantkennis te ontwikkelen op zodanige wijze dat die kennis directe toegevoegde waarde heeft voor de onderneming?
Maar wanneer heeft klantkennis eigenlijk voldoende commerciële, dus toegevoegde waarde? Dat hangt af van de mate waarin de informatie:
• volledig is: omdat er anders misschien cruciale informatie mist;
• consistent is: omdat de klant anders zal gaan twijfelen over de juistheid van de informatie of zich kan gaan storen aan het feit dat er geen rekening wordt gehouden met de inhoud van eerdere gesprekken;
• persoonlijk is: omdat klanten zich anders minder aangesproken voelen en er dus minder aandacht aan wordt besteed;
• afgestemd is op het moment waarop de klant ontvankelijk is: een verkeerd moment betekent dat de klant niet ontvankelijk is voor de propositie;
• aanwezig is op plaatsen waar het klantcontact plaatsvindt: als de klantinformatie ontbreekt, kan er ook geen gebruik van worden gemaakt.
Klantkennis ontwikkelen: data mining
Het probleem is bekend. Er worden ontzettend veel – en steeds meer – klantgegevens op allerlei manieren opgeslagen. Gelijktijdig geldt dat naarmate meer klanten worden binnengehaald, het beeld dat we van al onze klanten hebben steeds waziger wordt. Het beeld wordt pas duidelijker als de veelheid van gegevens wordt omgevormd tot informatie: kennis.
Vaak liggen klantgegevens opgesloten in diverse databases en even vaak worden die gegevens niet gebruikt omdat op het moment van beslissen/verkopen de gegevens niet rechtstreeks toegankelijk zijn en er specialistische vaardigheden en instrumenten nodig zijn om de ruwe data om te zetten naar kennis over de klant.
Data mining zoekt (delft) in grote hoeveelheden (klant)data en zet deze data met behulp van berekeningen en toevoeging van marketingkennis om in klantkennis, onder andere via scoremodellen. De scoremodellen voorspellen wie, wanneer, en via welk distributiekanaal positief zal reageren op een bepaald aanbod. Dit is een toegevoegde waarde die zelfs een ervaren marketeer nooit kan leveren zonder gebruik te maken van statistiek/data mining. Vervolgens worden de modellen ingezet om een beter begrip te krijgen van het klantgedrag. Op basis van deze informatie kunnen weloverwogen beslissingen worden genomen over bijvoorbeeld het wel/niet verkopen van een bepaald product (aan een bepaalde klant).
Met behulp van data mining wordt dus nieuwe kennis gecreëerd van bijvoorbeeld het verkopen van meer producten. Data miningtechnieken hebben optimaal resultaat als de klantgegevens zijn vergaard uit allerlei distributiekanalen en de kennis van marketinganalisten wordt benut bij het omzetten van de klantdata in scoremodellen. Zo maakt data mining bergen data toegankelijk voor een grote groep eindgebruikers (zoals marketeers, call center agents en adviseurs).
Klantkennis verspreiden: multichannel personalisatie
De tweede vraag aan het begin van dit artikel was: Wat moet een bedrijf doen om deze kennis toe te passen en te verspreiden? Het verspreiden van klantkennis is immers nodig om het bedrijf als geheel te laten profiteren van de ontwikkelde klantkennis (vastgelegd in scoremodellen). Bij de verspreiding van klantkennis moet rekening worden gehouden met alle verschillende manieren en momenten waarop veel medewerkers interacteren met de(zelfde) klant waarbij klantinformatie nodig is.
De volgende vragen zijn daarbij vanuit KM-perspectief relevant:
Welke klantkennis willen we verspreiden?
Los van de kwaliteit en de vorm van de klantinformatie gaat het hierbij over kennis van:
• De situatie van de klant. Bijvoorbeeld een overzicht van de verschillende gedragingen (informatiezoekgedrag, koopgedrag, betaalgedrag. . .) of de waargenomen veranderingen in het gedrag (recente contacten, gebruiksfrequentie, etc.).
• Het product dat het beste kan worden aangeboden, aangevuld met informatie over de manier waarop men dit het beste kan doen, en de onderliggende reden waarom dit product het meest geschikt is.
• De eigen organisatie. Bijvoorbeeld algemene bedrijfsafspraken over welke klanten wel of niet te benaderen en hoe vaak men klanten mag benaderen in een bepaalde periode.
Hoe verankeren we de klantkennis in het bedrijf?
In ideale situaties maakt de eindgebruiker bij elke beslissing (over een aanbod aan de klant) gebruik van de aangeboden klantkennis (haalplicht). Dit kan worden bevorderd door de eindgebruiker te belonen voor het gebruiken van de kennis (bijvoorbeeld een incentive op het behaalde resultaat). Een andere vorm van belonen is door een goede en snelle terugkoppeling van de behaalde resultaten de medewerker laten zien dat gebruikmaken van de geboden informatie resultaat heeft. De medewerker krijgt niet alleen toegang tot de informatie, maar heeft ook de plicht deze aan te vullen met zijn ervaringen (brengplicht). Dan kan de nieuw opgedane kennis worden gebruikt om scoremodellen en persoonlijke marketingprogramma’s naar de klant te verbeteren. Pas als er zowel een haalplicht als een brengplicht is, kan worden gesproken van een verankerd gebruik van klantkennis.
Welke structuur is nodig om kennis te verspreiden?
Voor de daadwerkelijke verspreiding is een centraal punt nodig van waaruit de klantkennis wordt verzameld, verstuurd en de informatie direct toegankelijk is. Wij noemen dit multi-touchpoint personalisatie. De voordelen ervan zijn:
• Dat alle klantervaringen worden samengebracht en er een eenduidig overzicht is van alle activiteiten ten behoeve van de klant (volledigheid);
• Dat men voor elke volgende interactie met de klant op de hoogte is van wat er daarvóór met de klant is gebeurd (consistentie);
• Dat bij voorkeur alle distributiekanalen direct in verbinding staan met het centrale punt. Dan kan bijvoorbeeld met behulp van routing sturing worden gegeven aan het palet van communicatiemogelijkheden als telefoon, e-mail, fax, e-mail en brief (op de juiste plaats);
• Dat informatie snel verspreid kan worden. Dit is het tijdig signaleren van kansen en het proactief voorbereiden op de interactie met de klant. Het exacte moment van het klantcontact laat zich niet bepalen. Multi-touchpoint personalisatie maakt het voor een bedrijf mogelijk zich zodanig voor te bereiden dat het op basis van de klantinformatie meer het initiatief naar zich toe trekt op het moment dat de klant belt of de webpagina bezoekt (getimed en persoonlijk).
Samengevat zorgt multi-touchpoint personalisatie er dus voor dat aan alle voorwaarden die onder het kopje commerciële waarde klantkennis werden genoemd, wordt voldaan. Dat klantkennis dus werkelijk die toegevoegde waarde krijgt voor een bedrijf.
Noodzakelijke veranderingen voor kennisgebruik
De derde vraag die we aan het begin van dit artikel stelden, was: Wat verandert er in de diverse bedrijfsprocessen, in de organisatie, en in de ICT-systemen en infrastructuur als men klantkennis commercieel wil inzetten? Om klantkennis commercieel in te kunnen zetten, zijn veranderingen nodig in de organisatie, in het marketing- en verkoopproces en in de ICT-systemen.
Veranderingen in de organisatie
Als een bedrijf data mining en multi-touchpoint personalisatie wil gebruiken om klantkennis commercieel in te zetten, hoe moet dat bedrijf zijn organisatie dan inrichten? Wie gaan met het systeem werken? Wat betekent dat voor de werkzaamheden van gebruikers?
Een verandering voor de organisatie is bijvoorbeeld dat medewerkers zelf verantwoordelijk worden voor het gebruik van klantinformatie en het aanleveren van nieuwe informatie. Zij moeten dan ook beslissingsbevoegdheid en de vrijheid krijgen over hoe zij omgaan met de klantinformatie. De verantwoordelijkheid voor het aansturen van de distributiekanalen wordt centraal neergelegd, bijvoorbeeld bij de marketingafdeling.
De nieuwe manier van werken zal ook aan een aantal functies een andere inhoud geven. Zo gaat een call center agent ook verkopen, verandert de werkwijze van de analist en krijgen marketingmanagers en marketeers een andere drive in hun werkzaamheden.
Klantinformatie voedt de organisatie doorlopend met kansen die zullen leiden tot actie. Het systeem wordt dus onderdeel van de dagelijkse werkzaamheden van werknemers. Het proces van creatief en vaak vrijblijvend klantinformatie gebruiken in acties naar de klant verandert in een productieproces.
Veranderingen in het marketing- en verkoopproces
Hoe gaat het marketing- en verkoopproces eruit zien? Hoe moet een bedrijf omgaan met zijn klantcontacten om optimaal gebruik te maken van de verkoopkansen? Het gebruik van multi-touchpoint personalisatie en data mining verandert de focus van een organisatie. Op het moment dat klantkennis het uitgangspunt is voor commerciële klantactiviteiten, verandert ook de focus van het marketing- en verkoopproces. Was er voorheen een productfocus, dan moet deze nu dus veranderen naar een klantfocus. De doelstellingen zullen dan ook niet meer op productniveau liggen maar op klantniveau.
Omdat multi-touchpoint personalisatie realtime informatie over verkoopkansen verschaft, heeft dit ook invloed op het marketing- en verkoopproces. Om die verkoopkansen te kunnen benutten, moeten immers snel beslissingen worden genomen over óf en hóe een organisatie die kansen gaat benutten. Er moet dus van tevoren worden nagedacht over hoe de klanten worden benaderd als zich bepaalde kansen voordoen.
Veranderingen in de systemen
Op welke manier kunnen data mining en multi-touchpoint personalisatie in de bestaande infrastructuur worden geïntegreerd? Aan welke technische randvoorwaarden moet zijn voldaan?
Om datamining en multi-touchpoint personalisatie te kunnen gebruiken zal software moeten worden aangeschaft. In de ideale situatie kan deze snel en makkelijk worden geïntegreerd in de bestaande ICT-infrastructuur. Ook alle front-end applicaties die een organisatie gebruikt om in contact te komen met haar klanten zullen worden aangesloten op de eerdergenoemde centrale applicatie. Dit geldt voor call centers, contact managers, sales force-applicaties, direct mailapplicaties et cetera. De integratie gaat nog verder: ook alle bestaande gegevensbestanden worden opengesteld voor en aangesloten op de centrale applicatie.
Conclusie
Hoe zet ik klantkennis in voor het verbeteren van mijn commerciële resultaten? Gebleken is dat het bij het commercieel inzetten van klantkennis voornamelijk draait om het tijdig signaleren en benutten van de interessante klantcontacten. De eisen die vanuit kennismanagement hieraan worden gesteld, worden met de combinatie van data mining en multi-touchpoint personalisatie goed ingevuld. Immers, belangrijke voordelen van deze aanpak zijn dat de klantinformatie een hoge (voorspellende) kwaliteit heeft (die consistent is over locaties, distributiekanalen en tijd), er een perfecte timing is in de presentatie (dat wil zeggen op het moment dat de kans zich voordoet), alle locaties bereikt kunnen worden waar medewerkers in contact komen met de klant, inclusief de interactieve kanalen. Daarmee biedt de oplossing goede mogelijkheden om het gehele bedrijf en zijn klanten te laten profiteren door de juiste klantkennis op de juiste plaats, op het juiste moment, in de juiste vorm en kwaliteit te verspreiden.
Analytische CRM, sturen op de winstgevendheid van de individuele klant
Gert Haanstra en Reinier Schut, Call Center Magazine, F&G Publishing, Amersfoort, Nr. 5, 2001
Printversie: Analytische CRM, sturen op winstgevendheid individuele klant
Hoe kun je als organisatie de loyaliteit bij klanten versterken en zo concreet bijdragen aan de winstgevendheid van de relatie? Analytische CRM, of kortweg aCRM, richt zich op het personaliseren van de klantcontacten rekening houdend met de winstgevendheid van die individuele klant. De focus in dit artikel wordt gelegd op het operationeel maken van het aCRM proces: het bouwen – en multichannel (via meerdere kanalen) toepassen in elk contact – van verfijnde klantprofielen, die zijn gebaseerd op de voorspellende kracht van individuele klantdata.
De opkomst van (analytische) Customer Relationship Management
Customer Relationship Management is populair. De technologie is sterk in ontwikkeling en er is veel aandacht in de media. Bedrijven, met de financiële instellingen voorop, investeren veel in CRM-trajecten. Vaak worden er gouden bergen beloofd. Logisch ook, in theorie levert het ontwikkelen van een goede relatie met de individuele klant immers veel geld op. Het lijkt profijtelijker om de relatie met de bestaande klanten te onderhouden en verder uit te bouwen, dan veel geld te steken in het voortdurend binnenhalen van nieuwe klanten. Echter, de vraag blijft of dit in de praktijk ook kan worden waargemaakt. In deze bijdrage presenteren we dat deel van CRM dat zich specifiek richt op het waarmaken van deze financiële belofte:; analytische CRM, of kortweg aCRM. In tegenstelling tot operationele CRM (oCRM) dat bedrijven in staat stelt om meer (van hetzelfde) te doen met minder resources, zorgt aCRM ervoor dat bedrijven hun marketing activiteiten beter uitvoeren. We kijken naar wat aCRM nu eigenlijk is, hoe je het inzet en welke opbrengsten je ervan mag verwachten.
De beweging naar multichannel marketing
Bedrijven zijn bezig hun service- en transactieprocessen verregaand te automatiseren. Hiervoor worden vaak operationele CRM-pakketten gekocht. Daarnaast willen bedrijven de klant beter tegemoet komen via een uitbreiding met nieuwe directe distributiekanalen, zoals callcenter, internet en mobile services. Hiervoor zet men collaborative CRM software in. CCRM software wordt ingezet om de verschillende kanalen en de diverse databases met elkaar te verbinden. Het zorgt ervoor dat informatie van de front office (=call center, web, etc.) snel en eenvoudig wordt doorgegeven aan de back office (=billing proces, transactie verwerking, etc. ), en vice versa. Ofwel, dat verschillende disciplines binnen een bedrijf makkelijker met elkaar kunnen communiceren via de verschillende systemen. De vraag die dan opkomt is: hoe kan ik mijn klant ongeacht het kanaal waarin het contact plaatsvindt optimaal bedienen met het juiste aanbod, het juiste kanaal en op het juiste moment? Oftewel: multichannel marketing met behulp van aCRM.
Als de (marketing)activiteiten over de verschillende kanalen niet goed zijn geïntegreerd zijn de gevolgen hiervan direct merkbaar. Een voorbeeld uit de financiële dienstverlening: de klant die het callcenter belt en de volgende dag zijn adviseur op kantoor bezoekt merkt met ‘meerdere instellingen’ zaken te doen: de adviseur weet niet de laatste stand van zaken zoals besproken met de callcenter agent. Deze versnippering komt pijnlijk in beeld op het moment dat besloten wordt om de nieuwe advies- en verkoopdiensten in het callcenter en op internet toe te voegen en samen te laten werken met de al bestaande diensten in het kantoor. Er is geen integraal klantbeeld over alle kanalen heen. Bovendien heeft elk kanaal zijn eigen set service-, verkoop- en adviestoepassingen en maakt daarbij (deels) gebruik van eigen klantdata. Vele toepassingen, die bij de klant tot irritatie kunnen leiden en die aan beheer- en onderhoudskosten bij de financiële instelling elk jaar tot kostenstijging leidt.
De versnippering van de kanalen staat een commercieel slagvaardige aanpak in de weg. De oplossing hiervoor ligt in aCRM. ACRM-software biedt de mogelijkheid om de verschillende kanalen op een consistente wijze te integreren met de bestaande back-end systemen. De kern van deze pakketten ligt in het inrichten – en onderhouden – van een actueel en uniform klantbeeld. Alle kanalen kunnen hiervan gebruik maken in elk contact met de klant. Om deze kern heen kan functionaliteit voor service-, marketing- en verkoopprocessen worden ontwikkeld en via het callcenter, kantoor of internet in het klantcontact aan de orde komen.
Aangezien de CRM-inspanningen worden gedreven door meer uit bestaande klanten te halen, is het niet verwonderlijk dat vele bedrijven bezig zijn met implementeren van een aCRM-pakket. Het uniforme klantbeeld vormt zowel voor de klant als voor de betreffende medewerker de basis voor de relatie en het startpunt voor het uitbouwen van de dienstverlening. De klant wordt ‘herkend’ en ‘erkend’, ongeacht het kanaal waar het contact is gelegd. Het uniforme klantbeeld is echter niet voldoende om daadwerkelijk ‘meer te doen met de bestaande klanten’.
De switch van outbound naar inbound
Als gevolg van toenemend direct contact met de klant – en tevens de irritatie van overvoering in telemarketing – is een verschuiving gaande van traditionele marketing acties voor grote doelgroepen naar het benutten van alle contacten met die ene klant. De toenemende directe, inkomende contacten in het callcenter en op internet worden benut voor zowel service als verkoop. In deze nieuwe oriëntatie verschuiven de marketing acties naar het regisseren en personaliseren van het contact. Elk contact geeft de gelegenheid om een propositie op maat te doen, die concreet bijdraagt aan de relatie met die individuele klant. Het klinkt goed, vraag is wel: hoe werkt dit nu precies?
ACRM software richt zich, in tegenstelling tot de algemene CRM software, specifiek op de vraag hoe kennis van klanten operationeel kan worden benut om ook elk contact te personaliseren en commercieel te benutten. Het is daarmee een operationalisatie van het slim inzetten van multichannel marketing: welke propositie aan welke klant in welk contact? De software is hierbij uiteraard een ondersteuning van het in te richten proces en de rollen in de organisatie.
Hoe sturing op winstgevendheid van de klant operationeel te maken
Hoe lost aCRM de uiteindelijke financiële belofte in? Door elk individueel klantcontact te beoordelen en de meest interessante contacten met de klant te benutten om de loyaliteit te versterken en de winstgevendheid te vergroten. Dat laat zich het beste verduidelijken aan de hand van het in te richten aCRM proces.
Marketingvraagstukken als acquisitie, het verbeteren van loyaliteit (cross- en upselling) en acties om de retentie te verbeteren zijn met aCRM gebaseerd op al bestaande kennis van de klant, opgeslagen in de data. In onderstaande figuur 1 wordt in drie stappen geïllustreerd en per stap toegelicht hoe deze kennis operationeel wordt vertaald naar proposities op maat.
Het aCRM proces bestaat uit drie stappen. Stap 1 vertaalt de individuele klantdata naar klantprofielen op basis van datamining technieken. Stap 2 maakt van deze klantprofielen proposities door scoring. Dit betekent dat outbound een groep klanten al kan zijn gescoord en deze de propositie bijvoorbeeld via (e)mail krijgen. Een andere groep, die bijvoorbeeld veel contact heeft via callcenter of internet, wordt online ‘gescoord’ op het moment dat het contact er is. Het voordeel is dat het actuele klantbeeld wordt benut om de regels van het klantprofiel te beoordelen. Op deze wijze wordt ook een actuele en juiste propositie aangeboden. In stap 3 wordt de commerciële actie geëvalueerd. Dit kan zowel leiden tot bijstellingen in het klantcontactproces en in de opleiding van medewerkers als tot een bijstelling van het model op basis waarvan de proposities werden gegenereerd. Daarmee is de loep gesloten en begint stap 1 opnieuw.
Stap 1: Het analyseren van data om te komen tot verfijnde klantprofielen
De data zijn een reflectie van het gedrag van de klant. Op basis van deze data kunnen er met behulp van datamining technieken klantprofielen worden gegenereerd. De profielen beschrijven nauwkeurig een kleine groep met een verhoogde kans om te reageren op bijvoorbeeld een retentie of cross-sell actie.
Geodemografische data blijken steeds minder voorspellend te zijn. Productbezit, productgebruik en frequentie en aard van de contacten zijn dat daarentegen steeds meer. In het algemeen wordt aCRM interessant als de klant bekend is (en geïdentificeerd kan worden) en er data over hem aanwezig zijn. Bij voorkeur grote hoeveelheden data (> 50.000 klanten). In zo’n situatie laten gebruikelijke analyse methoden het veelal afweten, en wordt het zinvol om met datamining technieken aan de slag te gaan. Het beschikken over deze data (afkomstig uit verschillende bronnen), het vervolgens opschonen en verrijken en tot slot met datamining technieken zoeken naar verbanden levert verrassende nieuwe inzichten op.
Een goed voorbeeld zijn de resultaten van een responsanalyse bij een bank. Hieruit bleek dat de meeste klanten positief reageerden nadat ze ongeveer zes, zeven keer waren benaderd. Er bleek duidelijk sprake van een optimum. Minder aanbiedingen leverden minder resultaat op. Ook meer aanbiedingen hadden een aflopend effect. Hoewel er een enkele uitschieter was die na 22 keer te zijn benaderd alsnog op de aanbieding inging.
Bij de vraagstelling “wat is voor deze klant de beste propositie?” kunnen meerdere proposities worden gegenereerd. De vraag is wat de beste propositie is en of deze mag worden aangeboden. Dit wordt mede bepaald door de prioriteit die het bedrijf stelt aan een bedrijfsdoelstelling (bijvoorbeeld retentie gaat voor cross-selling) en de voorkeuren die de klant zelf heeft. Bijvoorbeeld, het kan zijn dat de klant heeft aangegeven niet benaderd te willen worden voor een bepaald product. Prioriteit in de propositie wordt ook bepaald door de ‘bedrijfsregels’. Een bedrijf kan er bijvoorbeeld bewust voor kiezen om het aantal proposities in een periode te beperken, om te voorkomen dat de klant geïrriteeerd raakt.
De klantprofielen zijn een operationele vertaalslag van een groot aantal data naar commerciële resultaten. Ze hebben grote voorspellende waarde, ze zijn makkelijk interpreteerbaar en direct in te zetten. Daarmee bieden ze de voor de verantwoordelijke klantgroep marketeers een goed middel om makkelijker hun targets te halen.
Stap 2: ‘Scoring’ van de klant in contacten in de vorm van proposities
Voor uitgaande contacten, denk bijvoorbeeld aan een mailcampagne, kan planmatig het profiel ‘gescoord’ worden tegen het klantenbestand. Het resultaat is een nauwkeurige selectie van klanten voor die campagne. Voor een ‘inkomend’ contact, bijvoorbeeld in een callcenter, wordt er ‘gescoord’ op het contactmoment met de klant. Immers het klantgedrag is dynamisch. Om irritatie te voorkomen wordt de score bepaald op basis van een actueel klantbeeld. Bovendien kan de klant in het gesprek zaken aangeven, zoals de uitbreiding van zijn huishouden met een kind, die onmiddellijk aanleiding zijn om een nieuwe propositie, bijvoorbeeld een jeugdspaarplan, te genereren. Op deze wijze wordt de propositie op maat gegenereerd en aangeboden op een natuurlijk contactmoment. We noemen dit een klantgerichte propositie.
Een online scoring is één, de presentatie en de besturing op de werkplek van de agent is zeker zo belangrijk. Uitgaande van een klantgerichte propositie is het belangrijk voor de callcenter agent om ‘het stuur zelf in handen te hebben’. Snel inzicht in het bestaan van een propositie, het zelf kunnen bepalen hoe en wanneer ingezet, de motivatie voor de propositie en het gemakkelijk (lees: snel) kunnen afwikkelen bepalen het succes. Dit geldt ook voor de selfservice op de internet site. Het is belangrijk verstandig om te gaan met de wijze waarop – en de mate waarin – een propositie wordt getoond. Overvoering en bannering zijn in alle gevallen de dood in de pot. Belangrijk punt voor de analyse is de registratie van het resultaat van de aanbieding. Op basis van de resultaten kan het succes worden geëvalueerd en kan de analyse in een volgende fase worden verfijnd.
Stap 3: Het evalueren van de (multichannel marketing) acties
De resultaten van de commerciële actie zullen moeten worden bewaakt en geëvalueerd. Het monitoren houdt in dat bijvoorbeeld de conversie wordt bijgehouden en frequent vergeleken met de voorspelling. Een te grote afwijking kan leiden tot nader ondezoek en bijstelling. De evaluatie geeft aan of de actie heeft geleid tot het beoogde doel. Met deze evaluatie is de cirkel rond. De evaluatie geeft aan of het klantprofiel voor dit type door veranderend klantgedrag bijgesteld moet worden, dus opnieuw analyseren (stap 1).
Wat een actie op kan leveren wordt geïllustreerd in figuur 3 met een voorbeeld van het gericht mailen van kleinere groepen klanten en ook met een voorbeeld van het genereren van klantgerichte proposities om inkomende contacten op een callcenter commercieel te benutten.
Voorbeeld 1: Outbound mailing naar meest winstgevende klanten
Stel, per jaar verstuurt een bank voor een specifieke spaarverzekering 150.000 mailingen aan haar klanten. De gemiddelde conversie is 1,5%. Hierbij is de gemiddelde verwachte winst NLG 3.000 (over 10 jaar) per contract. In totaal worden 2.250 contracten verkocht. De totale winstbijdrage bedraagt NLG 6,8 miljoen gulden.
Door een model te maken waarmee de meest kansrijke en meest winstgevende klanten worden geselecteerd, zal de totale winstbijdrage stijgen. Bijvoorbeeld, bij een conversie van 2,5% op 100.000 mailingen, zullen 2.500 contracten worden verkocht. De winstverwachting ligt hoger, namelijk op NLG 3.500 gulden. De totale winstbijdrage is daarmee NLG 8,8 miljoen gulden. Een extra winst van NLG 2 miljoen gulden (over een periode van 10 jaar).
Voorbeeld 2: Inbound benutten van verkoopkansen in het callcenter
Stel, op het callcenter wordt online scoring gebruikt waarmee de meest kansrijke contacten worden gesignaleerd, en waarmee vervolgens de agent een duidelijke suggestie krijgt van welk product hij moet aanbieden. Dan geldt:
• 100.000 inbound contacten per maand.
• 5.000 contacten zijn potentieel interessant voor verkoop (=5%)
• 500 contracten gesloten (=10% van 5.000)
• NLG 3.500 gulden winstmarge (over 20 jaar)
• additionele winstbijdrage: NLG 1,8 mln per maand (=500 contracten * 3.500 gulden)
Sturen op winstgevendheid van de individuele klant
CRM is een business strategie die vraagt om een flinke investering en een goede conditie. De investering is slechts voor een (klein) deel in hard- en software, maar is vooral een investering in mensen. Bedrijfsprocessen zullen veranderen en functies zullen wijzigen, wat vraagt om training, begeleiding en motivatie (uit het management). Het implementeren van aCRM in een bestaande infrastructuur is een minimale inspanning van maanden. Een inspanning die u wel geld gaat opleveren. Immers, aCRM is erop gericht om de winstgevendheid van uw klanten te vergroten. Om dit te bereiken wordt een drietraps proces ingericht. Stap 1 is op basis van individuele klantdata profielen te genereren. Stap 2 is deze profielen in te zetten via proposities op maat bij elk klantcontact, de zogenaamde ‘scoring’. In stap 3 vindt de bewaking en tenslotte de evaluatie plaats van de commerciële actie. De genoemde voorbeelden laten zien dat deze directe sturing op klantniveau zeer winstgevend kan zijn.
Wie kiest u als klantenmanager?
Gert Haanstra en Marcel Holsheimer, Telebusiness Magazine, tijdschrift voor interactief customer contact management, BBP bv, Woerden, Nr. 3, 2001
Printversie: Wie kiest u als uw Klantenmanager
CRM helpt organisaties hun klantrelaties te managen, maar om dit optimaal te kunnen doen is inzage in klantgedrag en klantbehoeften noodzakelijk. Nog te vaak roept CRM vragen op. Hoe laat ik mijn klant zien dat ik hem en zijn behoefte werkelijk ken? Wat is het verschil tussen analytische en operationele CRM? En, last but not least, waar moet ik op letten bij de keuze voor een leverancier van CRM-oplossingen? Om deze laatste vraag te kunnen beantwoorden en zo een verantwoorde beslissing te kunnen nemen, zult u eerst de CRM-markt moeten leren begrijpen.
Vertwijfeling
Dat CRM twijfels en vragen oproept is zeker. Verdeeldheid onder managers van verschillende kanalen of producten die elkaar het gras voor de voeten wegmaaien in hun ijver de nieuwe mogelijkheden te benutten. Ontzetting bij de bedrijfsleiding die ziet dat kanalen en producten elkaar kannibaliseren, slapeloze nachten bij de ICT-afdeling die al die verschillende gegevensstromen uit al die kanalen in goede banen moet zien te leiden. Soms verwarring en ergernis bij de klant die ziet dat uw bedrijf op allerhande manieren contact met hem zoekt, maar die toch elke keer weer onzinnige of dubbele aanbiedingen krijgt. Of een klant die voor de zoveelste keer zijn gegevens moet invullen. Zaak is u niet te laten afschrikken om met CRM door te gaan, maar er voor te zorgen dat u de juiste beslissingen neemt, bijvoorbeeld over de keuze van een leverancier.
Goede beslissingen leveren u geld op. Dit geldt ook voor de keuze van een softwareleverancier. Duidelijk is dat er slechts enkele partijen zijn die tot nu toe concreet hebben bewezen dat ze echt succesvol kunnen zijn in het neerzetten van een business case. En dat maakt het voor u niet eenvoudig om de juiste beslissing te nemen.
Wel of niet een (extra) investering?
Een van de eerste zaken waar u tegen aan zult lopen is de vraag of een extra investering nodig is. Immers, u doet al aan CRM. Zeer waarschijnlijk heeft u een database met klantgegevens. U maakt daar misschien al selecties uit van groepen mensen die u dan, misschien wel gebruik makend van specifieke software, een brief of brochure wilt sturen. Of u heeft een website en een call center en u reageert op informatieverzoeken van klanten.
Het zijn voorbeelden van operationele CRM (oCRM) waarmee u het contact met uw klant (en de klant het contact met u) sneller en beter kunt afhandelen. De oCRM applicaties maken het werk van uw medewerkers een stuk makkelijker. Helaas is het efficiënt afhandelen van klantcontacten niet hetzelfde als een concrete verbetering in de winstgevendheid van uw klanten. De applicaties worden slechts gevoed met marketing ervaring van uw medewerkers. Maar enkel het inzetten van marketing ervaring is niet altijd voldoende om er voor te zorgen dat uw meest winstgevende en meest loyale klant het meest aantrekkelijke aanbod krijgt, op het juiste tijdstip (bijvoorbeeld als de klant u benadert) en via het beste kanaal.
U kunt zich afvragen of er geen extra (analytische) functionaliteit bij zou moeten. Analytische CRM (aCRM) geeft u de mogelijkheid om bijvoorbeeld Real Time Marketing te realiseren (waarbij het accent ligt op het direct benutten van binnenkomende verkoopkansen) of Customer Life Time Value Marketing (waarbij een switch wordt gemaakt van het maximaliseren van de verkoop van producten naar het optimaliseren van de winst van de verschillende klantgroepen).
Met behulp van aCRM software achterhaalt u met behulp van geavanceerde data mining technieken wie uw meest waardevolle klanten zijn en welke klanten of prospects een gemiddeld hogere kans hebben om te converteren. Deze klantprofielen worden vervolgens ingezet voor optimale doelgroepselecties voor mailingen, genereren van bellijsten en voor het bezoeken van kansrijke ‘leads’. Daarnaast worden de klantprofielen direct gebruikt voor het signaleren en benutten van de beste cross-selling en up-selling kansen op inbound klantcontacten. ACRM geeft u de mogelijkheid een winstgevende bedrijfsstrategie te ontwikkelen die uitgaat van uw klanten.
Wie moet ik kiezen en waarom?
De keuze van de leverancier hangt sterk af van het uiteindelijke doel dat u met behulp van CRM wilt bereiken. Maar de architectuur van het pakket is van even groot belang. Immers, tenzij u het bedrijf helemaal vanaf de grond (opnieuw) wilt gaan opbouwen, zal uw IT-afdeling de software willen integreren in de bestaande infrastructuur. Een goede integratie zorgt er voor dat alle gewenste functionaliteit over de verschillende applicaties heen gebruikt kan worden. Concreet betekent dit dat interessante (verkoop)kansen en ernstige bedreigingen overal en altijd moeten worden gesignaleerd en direct door iedereen kunnen worden gebruikt, bijvoorbeeld in het rechtstreekse gesprek met de klant. Bovendien zorgt een integratie ervoor dat u en uw medewerkers altijd volledig op de hoogte zijn. Daarbij gaat het er niet om of u de klant belt, mailt of een brief stuurt. Door het integreren van data verzamelen, consolideren, analyseren en distribueren kunt u optimaal gebruik maken van de gegevens die u over uw klanten heeft. Zo kunt u laten zien dat u uw klant werkelijk kent en hem een passend en consistent aanbod doen, via welk kanaal hij ook met u in contact komt.
Snelheid is een bijna allesbepalende factor voor het slagen van uw CRM-strategie. Kansen en bedreigingen moeten direct als zodanig worden herkend zodat u geen gelegenheid verliest om de kans te benutten of de bedreiging te counteren. Alleen als de verschillende applicaties goed zijn geïntegreerd, kunt u binnen seconden reageren en voorkomen dat u die klant verliest. Bijvoorbeeld door hem direct een mooi aanbod te doen als hij uw call center belt met een vraag of klacht. Had u hem de volgende dag een brief gestuurd met dat aanbod, dan was u misschien te laat of had u niet de kans gekregen om het aanbod met hem te bespreken. Ofwel, een gemiste kans.
Integratie zorgt er ook voor dat medewerkers via alle kanalen op de hoogte zijn van wat er in andere kanalen is gebeurd met een klant. U kunt alleen een consistent en volledig beeld vormen van uw klant als alle inkomende en uitgaande gegevens over uw klant goed bijeen worden gebracht, worden geïntegreerd. Dan kunt u uw activiteiten laten aansluiten op elkaar, zonder te kannibaliseren tussen de kanalen, dubbele aanbiedingen te doen of aanbiedingen te doen die niet gewenst zijn. Zo voorkomt u dat de klant u gaat verlaten. Maar dat werkt alleen als de onderliggende architectuur van het aCRM pakket een dergelijke integratie toelaat.
Open architectuur noodzakelijk
Als u kiest voor een softwareleverancier met een ‘open architectuur’ bent u in staat nieuwe software eenvoudig en snel te integreren in uw bestaande infrastructuur. Het heeft een aantal voordelen. Er is minder tijd nodig om de software te implementeren. Met kleine aanpassingen in een aantal instellingen werkt het pakket direct. En er hoeft geen klantspecifieke (integratie)software te worden geschreven. De kosten blijven dan ook beperkt omdat alleen het standaard pakket wordt geïnstalleerd. Daarnaast zijn er geen desinvesteringen in eerder gekochte software en hardware, want die blijft u gebruiken. Bovendien hoeven bij upgrades de onderlinge koppelingen niet worden aangepast en blijft de integratie moeiteloos intact. Het onderhoud van de integratie is zo wel erg eenvoudig met behoud van functionaliteit.
Het begrip ‘open architectuur’ houdt in dat op verschillende, algemeen geaccepteerde manieren, informatie en resultaten worden doorgesluisd naar andere applicaties. Open standaard architectuur heeft betrekking op de keuze van het platform waarop de database draait, het platform waarop de applicaties draaien en de manier waarop applicaties onderling met elkaar communiceren. Een leverancier moet in ieder geval de algemeen geaccepteerde ‘smaken’ kunnen leveren. Bij de serverplatforms gaat om de Windows- en Unix-smaken. Voor de applicatie platforms is Java compliant (als Java Beans geïmplementeerd) noodzakelijk. De n-tier aanpak heeft daarbij een grote voorkeur (met pakketten als Websphere en Weblogic), waarmee het ‘oude’ server/client concept zijn langste tijd lijkt te hebben gehad. Voor communicatie protocollen worden TCP/IP en MQ-Series veel gebruikt, en zijn XML en PMML moderne standaards en COM/DCOM vaak toegepaste alternatieven.
Solide investering
Veel bedrijven twijfelen over de resultaten van (huidige) CRM projecten, en zijn daarom terughoudend in het doen van een (extra) investering. De keuze van de juiste software-/hardwareleverancier lijkt hierbij een obstakel.
Vast staat dat een goed aCRM pakket direct geld oplevert. Dit is te bewijzen aan de hand van eerdere implementaties. Daarnaast kan de toegevoegde waarde worden aangetoond tijdens het project. Bijvoorbeeld door te werken met een business case die aantoont dat de winstgevenheid van uw klanten daadwerkelijk is verhoogd.
De keuze voor een pakket met een open architectuur is een voorwaarde. Een dergelijk pakket laat zich eenvoudig en volledig integreren, zodat alle benodigde functionaliteit voor iedereen snel en overal beschikbaar is. Een investering in open, analytische CRM is daarom een solide investering waarmee u de mogelijkheid krijgt om interessante (verkoop)kansen te benutten, en de winst op klantgroepen te optimaliseren.
Orginele publicatie: Klik hier
Strijd om het hart van CRM
Gert Haanstra en Marcel Holsheimer, Informatie maandblad voor de informatievoorziening, ten Hagen & Stam, Den Haag, December 2000, jaargang 42, blz 26 en 27
Printversie: Strijd om het hart van CRM
Customer Relationship Management (CRM) is populair. De vakbladen staan er vol van, ook in de directiekamers wil men weten wat CRM kan opleveren en steeds meer softwareleveranciers brengen CRM applicaties op de mark die de analytische, operationele, infrastructurele kant van CRM bedienen. Maar het CRM landschap zal er de komende jaren heel anders gaan uitzien. Alleen de leveranciers die een centrale plaats in het CRM proces weten in te nemen zullen overleven.
In beweging
Customer Relationship Management ontwikkelt zich tot een volledig geaccepteerde bedrijfsstrategie, gericht op verhoging van de Customer Life Time Value. Gericht op winst dus. De essentie van CRM is dat een organisatie zich in zijn totaliteit gaat richten op de individuele klant(contacten). Tijdens deze contacten probeert het bedrijf de eigen service te verbeteren door uit te gaan van de wensen en behoeften van de klant. De juiste technologie is hierbij een cruciale voorwaarde om CRM te laten werken. Maar wat is de juiste technologie? Het is voor leveranciers niet eenvoudig te begrijpen wat de markt nodig heeft. Slechts enkele leveranciers kunnen hun toegevoegde waarde vatten in een eenduidige productvisie die rekening houdt met toekomstige ontwikkelingen. En maar heel weinige kunnen de waarde van hun visie bewijzen met geslaagde toepassingen hiervan. Dat valt ook niet mee want de eisen uit de markt veranderen voortdurend en snel. Leveranciers die geen duidelijke visie hebben zwabberen daarom voortdurend heen en weer, om tegemoet te komen aan de vraag uit de markt. We zien verschillende verschuivingen in de markt.
Van operationeel naar het hart
Leveranciers van Operational CRM applicaties (zoals campaign management, sales force automation, web-service en call center applicaties) willen zich niet meer beperken tot het efficiënt afhandelen van klantcontacten in een enkel kanaal. Zij zoeken naar nieuwe functionaliteit die het mogelijk maakt alle distributiekanalen aan te sturen.
Van de backoffice naar het hart
Leveranciers van Collaborative CRM pakketten (bijvoorbeeld ERP-systemen, mid-office systemen en datawarehousing) willen meer bieden dan enkel het ontsluiten van klantgegevens. Ook zij zoeken nieuwe functionaliteit die kennis over klanten efficiënt verspreidt over de verschillende kanalen.
Van infrastructuur naar het hart
Leveranciers die zich tot nu toe bezig houden met het neerleggen van een infrastructuur (bijvoorbeeld process ware, copy management software en middle ware) zoeken naar uitbreiding. Vooral richting het ‘intelligent’ aansturen van klantinteracties.
Van datamining naar het hart
Leveranciers van Analytical CRM applicaties (bijvoorbeeld voor data mining, personalisatie en analyse) gaan zich met meer bezig houden dan enkel het modelleren van klantgedrag en achterhalen van het juiste aanbod, juiste klant, juiste kanaal en juiste moment. Zij gebruiken de resultaten van hun analyses om interessante klantinteracties te signaleren en benutten.
Functionaliteit in het hart; Integrated Customer Analytics
In het hart van CRM draait alles om de functionaliteit die nodig is om de totale opbrengst en winst over langere termijn voor elke individuele klant (Customer Life Time Value) te maximaliseren. Integrated Customer Analytics biedt die functionaliteit. Hiermee wordt klantkennis geanalyseerd om tot gefundeerde modellen te komen die tot op zekere hoogte kunnen voorspellen hoe een individuele klant zich zal gaan gedragen. Dat verklaart de woorden Customer en Analytics, maar nog niet Integrated. Geïntegreerd houdt in dat de klantkennis verzameld en weer verspreid wordt over de verschillende distributiekanalen heen, op elk willekeurig tijdstip en voor diverse proposities en bedrijfsdoelstellingen. Die integratie vanuit een centrale positie is nodig om werkelijk concurrentievoordeel te kunnen behalen, want:
• Om goede analyses te kunnen doen, hebben we volledige (dus ook de meest recente) informatie nodig over elke klant. Die informatie kan voortkomen uit de klant zelf (als hij bijvoorbeeld belt, de website bezoekt of een offerte retourneert. Het kan ook informatie betreffen die vanuit het bedrijf wordt geïnitieerd (direct mail, of een telefoontje naar de klant). Informatie die verzameld wordt vanuit verschillende kanalen en op verschillende momenten.
• Om elke klant optimaal en consistent te benaderen hebben we ook een eenduidig inzicht nodig in alle activiteiten richting de klant. Die benadering moet via elk gewenst distributiekanaal en op elk gewenst moment kunnen gebeuren. Alleen dan is het mogelijk om uit analyse gebleken kansen tijdig te signaleren en zich pro-actief voor te bereiden op interactie met de klant. Het moment van interactie wordt immers steeds vaker door de klant bepaald, bijvoorbeeld als de klant belt, of de web site bezoekt. Dat betekent dat de informatie snel verspreid moet worden vanuit het centrale punt, vanuit het hart van CRM.
Overleven in het hart
Een leverancier die op de centrale positie van CRM wil overleven moet kunnen samenwerken. Samenwerken met gelijk welke applicatie, database of infrastructuur. Om die samenwerking te kunnen realiseren moet je heel wat in je mars hebben: Intelligente tools waarmee de voorkeuren en gedragingen van de klant kunnen worden achterhaald. Tools met voorspellingskracht voor het ontwikkelen van gerichte proposities richting die klant. Tools die bovendien de doelstellingen op klantniveau in overeenstemming brengen met de bedrijfsdoelstellingen. Dan weet een organisatie wat hij zijn klant wil aanbieden. Nu nog uitvoeren!
Daarvoor zijn tools nodig die de communicatie met de klant op allerlei manieren initieren en bevorderen. Via allerlei distributiekanalen, contactmomenten, voor verschillende klantsegmenten en proposities. Van al die klantcontacten kan een organisatie veel leren. Een leverancier die zal overleven moet dus wel de ruimte bieden voor verbetering zodat de lange termijn doelstellingen worden bereikt.
Verder bepaalt de oorsprong van de leverancier de technische mogelijkheden die nog in het nieuwe gebied kunnen worden behaald (Gartner, 2000). Immers, de technologie zou wel eens heel snel verouderd kunnen zijn omdat in het verleden is gekozen voor een verkeerde architectuur. De technische ontwikkelingen gaan zo snel dat verkeerde keuzes aan de basis nauwelijks meer kunnen worden hersteld. De technologie voor het hart van CRM moet in ieder geval makkelijk uitgebreid kunnen worden over alle distributiekanalen, en eenvoudig geïntegreerd worden in de bestaande infrastructuur.
Gewaarschuwd
CRM biedt geweldige mogelijkheden. Maar een waarschuwing lijkt op zijn plaats. De wensen en mogelijkheden veranderen zo snel dat organisaties bij de keuze voor een CRM leverancier niet alleen de beschikbare functionaliteit van een pakket als criterium kunnen hanteren. De toekomstvisie van de leverancier en diens keuze in architectuur zijn van evengroot belang. Alleen zij die de juiste keuzes hebben gemaakt , hun beloftes waarmaken en bovendien met u kunnen en willen samenwerken, zullen overleven. Deze leveranciers worden het kloppend hart van de toekomstige CRM.
Orginele publicatie: Klik hier
Checklist Customer Relationship Management
Gert Haanstra, Checklisten InformatieManagement, Ten Hagen & Stam, Den Haag, Nr 38, 2001
Printversie: Checklist Customer Relationship Management
Orginele publicatie: Checklist implementeren CRM
Vertalingen
From shareholder value to customer value
Management according to anticipated customer returns is now possible using new analytical CRM techniques
Printversie: Banking Review-article From shareholder value to customer value
Gert Haanstra was responsible for product marketing and product strategy at Data Distilleries, a supplier of Analytical CRM software. Mr Haanstra has published several articles in national and international journals and is a regular speaker at CRM events, seminars and conferences.
Any financial institution is ultimately assessed according to its profitability. However, commercial departments are seldom managed with a view to making profits, but on the basis of turnover or costs. Translating profit objectives into concrete activities directed at the customers themselves has been regarded as too difficult or time-consuming. Until now, that is.
Cost reductions are finite
Financial institutions have been able to increase their profitability primarily through cost control: process automation, branch closures and greater use of the more efficient channels such as the call centre and electronic banking. This approach was implemented some time ago. It would now seem to have run its course, in that there remains little financial advantage to be gained. Yet there are opportunities for financial institutions to direct their activities with an eye to the individual customer, thus increasing profitability for both the customer and the company.
Conditions
The background and possibilities of Customer Relationship Management are regularly discussed in Banking Review. Financial institutions have considerable information concerning their customers, providing plentiful opportunities to gain a greater understanding of the client base as a whole. One example would be a refined segmentation of the client base according to current customer value combined with potential customer value.
The information held is also valuable as a predictive tool in that it reflects consumer spending and investment behaviour. Such information can be analysed and the results used to aid sales of new or additional products. This predictive aspect is particularly useful in enabling customer-specific offers to be made. However, an essential precondition is that the data analysts produce results which are readily accessible to marketeers and managers, who must then be able to use those results immediately in their customer contact. Examples of such analyses include the customer profile in which the customers most likely to be interested in an endowment investment policy are pre-selected, or a list of names and addresses of those customers most likely to respond to a ‘green’ investment fund proposal.
In order to increase profitability by means of this ‘customer-directed’ approach, a number of conditions must be met:
• Every promising contact with the customer (through any channel and at any time) must be identified and exploited. In other words, there must be a multi-channel approach. After all, today’s customer makes contact with his financial institution from any location and at any time. During the contact, it is extremely important that the customer’s attention is maintained. This can be achieved by tailoring the message or advice to his own personal wishes and requirements.
• Product-oriented or campaign-oriented managers with turnover targets at product level should give way to those serving a specific client group or segment, the aim being to increase the customer value of the client group.
The translation of knowledge into activity
Even where these two conditions have been met, the concept of ‘managing according to customer profitability’ will not yet be in place. Often, the missing factor will be a translation of the information concerning customers with greater potential for profitability into concrete actions directed at those customers. A crucial point is therefore the manner in which financial institutions adopt the ‘customer-directed’ approach in their day-to-day sales and marketing activities, thereby improving the returns for the company. In practice, this becomes possible through:
1. Approaching those customers with the greatest profit potential. Whenever the marketeer takes a decision to initiate a campaign or customer contact, he must have immediate access to information concerning such factors as the likely costs of the contact, the anticipated revenue, the potential customer profitability and the total profit contribution of the contact. These factors can be calculated on the basis of underlying predictive models.
2. Offering the products with the greatest returns. Here, it is necessary to calculate all relevant factors in advance and to give priority to the sale of these ‘high-return’ products. During the customer contact, the high-return products can then be offered ‘at the expense’ of another product. The priority assigned to a product must apply through all channels to ensure that the same personal offer is made to the customer, regardless of the form of communication chosen. Furthermore, general principles such as making only one offer per month must also be observed.
3. Making best possible use of inbound call centre contacts. All too frequently, the call centre is regarded as a service channel devoted to dealing with customer queries. Given appropriate CRM techniques, it becomes possible to ‘convert’ a service call into a sales opportunity. This sales opportunity will result in revenue, whereupon the time spent in selling can be charged on to the marketeer, and re-investment made in extra call centre personnel. In other words, the standard of service will remain constant while this channel also contributes to profit through its generation of turnover.
Profitable 1-to-1 customer relationship
The opportunities described above demonstrate that the gulf between shareholder value and customer value can indeed be bridged. The promise of maintaining a profitable ‘one-to-one’ relationship with the customer will also be seen to have been kept. The examples below demonstrate that direct management at customer level offers valuable opportunities and can be extremely profitable.
Example 1: Outbound mailing to customers with highest profit potential
Let us suppose that a bank sends out 150,000 mailshots for a specific savings policy product each year. The average take-up rate is 1.5%, representing an average anticipated profit of NLG 3,000 per contract over a ten-year period. A total of 2,250 policies are sold. The total contribution to profit is therefore NLG 6.8 million.
By producing a model to select the customers with highest profit potential, this total contribution to profit can be significantly increased. For example, with a take-up rate of 2.5% on a mailing of 100,000, there may be 2,500 contracts sold. The anticipated profit is also higher, at NLG 3,500. The total contribution to profit will then be NLG 8.8 million (over a ten-year period), being NLG 2 million greater than in the previous scenario.
Example 2: Converting inbound call centre queries into sales opportunities
Let us suppose that a call centre uses CRM techniques to identify the contacts with greatest sales potential. The call centre agent is automatically notified and is presented with a suggestion regarding the product he should offer (and why).
If we assume:
– 100,000 inbound contacts per month.
– 5,000 (= 5%) with potential sales opportunity
– 500 (= 10% of 5,000) contracts actually closed
– NLG 3,500 profit margin (over twenty-year period)
then the total contribution to profit will be NLG 1.8 million per month (= 500 contracts x NLG 3,500)
Analytic Customer Relationship Management
Analytic Customer Relationship Management (A-CRM) techniques are a separate component of the overall CRM concept. A-CRM is designed to strengthen the relationship with the customer and to increase profitability (customer value) by means of an analysis of individual customer information, resulting in the generation of ‘customised’ proposals. Specific products can then be offered during direct contact with the customer. A-CRM techniques also enable evaluation of the results of this approach.
Turning a service call into a sales opportunity
Earning money on incoming telephone calls
Printversie: Turning a service call into a sales opportunity
Over the last few years the management of customer relations has changed rapidly from a low-key, service-dominated market into a competitive business. A proactive approach to inbound customer contacts is fast becoming the norm – and is proving highly profitable. In order to exploit opportunities successfully, the call centre employee must be able to convert a service call into a sales call. Today’s personalised analytical Customer Relationship Management (aCRM) technology can provide just the support the employee needs. The system generates a recommendation relating to the product the employee is aiming to sell. This can optimize the decision-making process for the employee, enabling him to exploit the selling opportunities more effectively.
Recommendations assist the employee during customer interaction
From an analysis of customer data, the employee is given a recommendation with a clear message stating which product to offer. The information is available immediately during the call, and is based on the calculations by an underlying data mining model. The recommendation supports the employee in anticipating requirements of the customer during the call, enabling him to sell products which must benefit the company. The information on which the recommendation is based will relate to decisions about cross-selling, but may also concern such matters as resolving complaints or increasing the profitability of the customer.
The technical side of the recommendation
Technology plays an important part in producing a good recommendation. The required customer information must be copied very rapidly from one database to the other. This requires regular updates (for example daily or weekly) to be sent from the call centre to a central database (for example the data warehouse). The data is used for the initial rating right at the start of the call, when the customer is identified.
In order to provide a high-quality recommendation, the latest relevant changes in the customer’s situation must also be recorded during the call. For example, if a customer transfers his balance, the employee will want to incorporate this information in a new (improved) recommendation. He therefore carries out an immediate recalculation based on the most up-to-date details.
Conditions for maximum return
In order to generate the maximum return from incoming calls, a number of conditions have to be met. According to Peppers & Rogers (‘Enterprise one to one’, 1997) incoming calls can only be exploited successfully under the following circumstances:
each customer must be able to be approached as a unique person. This means the company must have a database with sufficient information about the customer. The information is ‘linked’ to the customer, for example by a customer number. This gives the call centre a better picture of the customer, for example on the basis of customer contacts in the past or the products previously purchased. The customer will have the impression that the employee knows him personally.
the company must be able to make personalised offerings which can be differentiated across a range of areas. These may include making appointments, offering services and selling products.
the communication between the customer and the employee must be effective. By listening well and showing empathy, an employee can find out whether the customer is satisfied with the company and its products and services, in order to take the appropriate action.
a personalised offering must be able to be adapted to the specific wishes and requirements of the customer. By customizing the offering, the employee can motivate the customer to purchase the product.
Preparing for implementation
In an ideal situation the implementation of the aCRM technology is preceded by an in-depth discussion to establish the extent to which the exploitation of customer contacts should be aligned with the overall business strategy. This involves adapting decisions concerning the organization, the management, the internal processes and staffing in order to meet the objectives.
The optimization of internal efficiency in the handling of customer contacts versus the development of intensive customer relations also plays an important part in the successful implementation of aCRM technology. The following points are of relevance:
a good balance must be achieved between the costs of a customer contact and the volumes which can be handled. For example, a company which expects a million telephone calls each year can ease the pressure on employees by using Interactive Voice Response (IVR) units. This system allows relatively simple actions, such as brochure requests, to be completed quickly, easily and at low cost.
a good balance must be achieved between efficiency in the handling of contacts and the attainment of the commercial objectives. For example, experience has shown that personal contact generates the highest number of products sold (per contact). But this channel can only process a limited number of contacts. Hence personal contact is only used if there is a relatively high chance of success and, for example, at night the Internet is used to provide information to interested parties.
the company must control the contact with the customer in such a way that customers will respond through the required channels, taking into account the costs of the channel and the expected volumes. The aCRM technology is then deployed selectively and flexibly, so that the most promising contacts are automatically identified and, for example, put straight through to an employee who takes over the call for the purpose of cross-selling.
Recommendations can be used in various ways
In what ways can a recommendation be used? On the basis of information in the database concerning a customer’s past, present and expected future, the recommendation can be used in the following ways:
‘event detection’. Relevant events (for example whether someone has a birthday or has recently requested information) are immediately detected and presented to the employee. The information can then be presented as:
a pop-up window showing a product and a scoring chance. This shows the employee which product should be offered and what chance it has of succeeding.
a traffic light showing a green, amber or red light, depending on whether there have been no complaints, minor complaints or serious complaints. This shows whether the customer is satisfied or dissatisfied. In the latter case a recommendation is then given for appropriate action to be taken to retain the customer.
‘performance management’. This shows the employee how profitable the customer is. The following solutions are possible:
a recommendation in the form of a medal (gold, silver or bronze) which indicates how this customer has performed. This enables an employee to devote extra attention to the best customers.
a customer pyramid. This is useful for showing the segment in which the customer is situated. The segment gives a clue to such matters as product ownership and/or the overall (expected) value of the customer.
‘customer profile’. In order to give the employee a more complete picture of this customer, relevant background information can be presented (at the same time as the recommendation), namely:
a description of the underlying data mining model,
a number of opening sentences which are appropriate for the offering,
a summary of all products,
graphic information on payment performance, information searching behaviour and contacts with the company.
Good aCRM technology combines customer information from various relevant sources and can process it in various ways to form a clear, easy-to-understand message for the employee.
No significant increase in employee’s workload
The presentation of a recommendation must not increase the employee’s workload too much. This can be achieved by:
‘prioritising the communication’. If only one message is shown, the employee will not become confused about which product he/she should offer. Moreover, no time is lost as a result of the employee having to choose.
‘flexibility in workflow’. Employees can decide individually whether they wish to be connected to the system, and hence whether or not to use recommendations.
‘high-quality prediction’. An employee must be able to rely on the quality of the recommendation, both with regard to the information and the underlying prediction. This must therefore be accurate and up to the minute.
‘timing of the recommendation’. Only relevant recommendations will be presented, at the appropriate moment.
From a technical point of view, the employee’s workload is controlled as follows:
only the recommendation with the highest score is presented. A condition is that all models must be calculated and the results compared, so that the recommendation with the greatest chance of success is presented.
‘threshold values’ are introduced which the employee can use to decide whether to take part. Moreover, the employee is presented with those recommendations with which he feels familiar and can therefore sell easily.
business rules are implemented which enable certain customers to be excluded (temporarily) if contact with them is not permitted (for example, a maximum of one offering every six months).
graphic presentation are used, with colours and symbols which are easy to understand.
Co-ordination with employee qualities
As well as the technical side, there is also the human side. Good co-ordination is necessary between the various skills of the individual employees and the offerings which are to be directed towards the customer. It is a question of recognising the specific qualities of the employee at an early stage and co-ordinating them with the recommendations from the database. In other words, as soon as a customer is identified for product A, this customer can be put through to somebody from the most suitable group of employees for product A. This can be achieved by means of number identification or by entering a customer and/or contract number at an early stage.
Improved results through greater interactivity
A final stage in the use of aCRM is greater interactivity. For example, specific customer requirements can be incorporated in the production of a recommendation. The information may not be available in the databases, but can be requested directly from the customer. Often just asking the right question (suggested from the database) suffices to begin a useful sales discussion. Here too the employee must be given an opportunity to listen, with guidance being given on the right questions to ask. An appropriate process of information gathering will make the customer feel he is being understood and will improve the quality of the prediction.
In short, aCRM technology in a call centre is a valuable tool for exploiting incoming customer contacts. The employee’s decisions can be optimised by automatically indicating the prospects of a sale and immediately producing a recommendation with regard to the right offering. It is voluntary participation and effective co-ordination between the technology and the qualities of the employee that will give the best result.
Web Mining: den einzelnen Kunden auf intelligente Weise ansprechen
Gert Haanstra, Mnet, media voor electronisch ondernemen, ten Hagen & Stam, Den Haag, Nr. 11, 2000
Printversie: Webmining in het Duits
Immer stärker wird der Druck, über Websites im Internet tatsächlich Umsätze zu erzielen. Es ist erkennbar, dass die Betreiber einiger großer Websites die Amortisation ihrer Investitionen mit Hilfe bewährter Techniken der eher traditionellen Wirtschaft, wie beispielsweise der Steigerung des Bekanntheitsgrads der Website mit Fernsehreklame oder der Steigerung der Kundentreue mittels Customer Relationship Management (CRM), erreichen wollen. Data Mining, die Analyse von Kundendaten, ist ebenfalls eine dieser bewährten Techniken. Wenn einige wichtige Vorbedingungen erfüllt sind, bietet es Betreibern von Websites die Möglichkeit, die Investitionen auf effektive Weise mit den Informationen über neue und alte Kunden mehr als auszugleichen.
Erfolgreich mit Web Mining
Was ist Web Mining? Web Mining ist Data Mining, das speziell auf Webdaten ausgerichtet ist. Zentral ist dabei die Nutzung der in der Datenbank enthaltenen Kundendaten. Diese Kundendaten werden analysiert und anschließend für eine Folgeaktion verwendet. Die Analyse erfolgt anhand verschiedener Techniken. Oft ist das Ergebnis eine Liste, beispielsweise eine Liste aller Personen, die vor kurzem die untersuchten Produkte gekauft haben. Ein weiterer Schritt ist die Entwicklung eines Score-Modells, mit dem sich vorhersagen lässt, bei wem die (größere) Wahrscheinlichkeit gegeben ist, dass das Produkt ein folgendes Mal gekauft wird.
Will man verkaufen, muss man dem Kunden, der die Website besucht, ein Angebot machen. Entscheidend ist dabei, das richtige Angebot vorzulegen, das heißt ein Angebot, „auf das der Besucher gewartet hat“. Dazu muss in dem Moment, in dem ein Kunde die Website abruft, untersucht werden, ob für diesen Kunden in der Datenbank ein Angebot vorliegt und ob dieses Angebot auch für diesen Kunden Attraktivität besitzt. Im Hintergrund berechnet das System speziell für diesen Kunden verschiedene Score-Modelle (= Echtzeitauswertung). Nach seiner Auswahl wird das aussichtsreichste Angebot bereitgestellt und auf einer Seite der Website präsentiert. Das Angebot kann in einem persönlich gestalteten Banner, einer Graphik, Seite usw. verarbeitet werden. All dies geschieht, während der Kunde die Website abruft.
Web Mining ist erfolgreich, wenn zum einen korrekte Vorhersagen gemacht werden und zum anderen das entwickelte Modell auch tatsächlich regelmäßig verwendet wird. Insbesondere Letzteres wird häufig vergessen und/oder falsch verstanden. Unternehmen vergessen oft, dass das Ergebnis des Modells zu einer konkreten Aktion gegenüber dem Kunden führen muss. Dabei kann es sich um eine spezielle Seite handeln, die im Laufe eines Websitebesuchs präsentiert wird, oder aber auch um einen Vorschlag an den Mitarbeiter im Callcenter, ein bestimmtes Produkt anzusprechen. Um für die Anschlussaktion eine klare Marschvorgabe abgeben zu können, muss der Endbenutzer (beispielsweise der Mitarbeiter im Marketing oder Callcenter) verstehen, „was geschehen soll“ und „warum dies besser ist als die bisherige Arbeitsweise“. Erst wenn er davon überzeugt ist, wird der Endbenutzer bereit sein, das, was das Modell von ihm verlangt, zu tun, und dem Kunden ein spezielles Produkt anbieten. Es dürfte deutlich sein, dass eine derartige Arbeitsweise hohe Anforderungen an den Aufbau des Modells und den Einsatz der ermittelten Ergebnisse stellt.
Was wird für Web Mining benötigt?
Ohne Datenbank und ein Instrument zur Analyse werden sich nur wenig ergiebige Resultate erreichen lassen. Die Datenbank mit den Kundendaten wird benötigt, um die Eigenschaften der Personen, die das Produkt kaufen, festzustellen. Ein wesentlicher Unterschied im Vergleich zum traditionellen Data Mining besteht darin, dass beim Web Mining nur ein relativ kleiner Kreis von Kunden, die regelmäßig die Website besuchen, erfasst werden muss, mit anderen Worten, eine kleine Datenbank, in vielen Fällen mit Daten gleicher Art. Im Idealfall kann die Internet-Datenbank mit bereits vorhandenen Datenbanken, in denen über einen längeren Zeitraum umfassendere Daten gesammelt wurden, verknüpft werden. Auf diese Weise kann das Bild des Kunden vervollständigt werden und lassen sich die einzelnen Kundengruppen differenzieren.
Welche Daten sind (möglicherweise) relevant für eine Vorhersage: Welche Seiten wurden besucht, welche Funktionen wurden abgerufen, welche Links wurden bewandert, auf welche Banner wurde geklickt? Oder wie sieht das Surfmuster aus und wie präsentiert sich das Klickverhalten? Wie oft wurde die Website besucht, wie lange dauerte der Besuch, wann wurde die Website geöffnet und wieder verlassen? Neben Verhaltensweisen können aber auch Präferenzen festgestellt werden. Mit dynamischen Seiten ist es sogar möglich, den Besucher während des Besuchs nach fehlenden Daten, die für eine gute Vorhersage notwendig sind, zu fragen. So kann man beispielsweise den Besucher fragen, ob er innerhalb eines Monats Urlaub machen will, wenn man feststellen will, ob eine interessante Ferienreise angeboten werden soll.
Die Techniken des Web Mining lassen sich weiter gliedern in die generischen Techniken, wie beispielsweise die kollaborative Filterung, bei der das Kaufverhalten eines willkürlichen Besuchers auf der Grundlage der Übereinstimmungen mit anderen Besuchern taxiert wird. Daneben gibt es die reinen One-to-one-Techniken, wie beispielsweise Assoziationsregeln, die vorhersagen, welche Produkte ein Besucher kaufen wird. Der Hauptunterschied besteht darin, dass bei den generischen Techniken die zu verkaufenden Produkte in den Mittelpunkt gestellt werden, während die One-to-one-Techniken von der gesamten Kundenbeziehung ausgehen. Letztere basieren nicht nur auf den Produkten, die ein Kunde erworben hat, sondern auch auf anderen Kundeneigenschaften, wodurch sich beispielsweise dann auch das Cross-Selling optimieren lässt. Daneben stützen sich die generischen Techniken bei ihren Vorhersagen auf ein allgemeines Kundenbild und eignen sich daher auch für anonyme Besucher einer Website. One-to-one-Techniken gehen dem gegenüber von einer Kombination individueller Eigenschaften der Kunden, die das Produkt gekauft haben, aus. Vorbedingung dafür ist allerdings die Identifikation des Kunden.
Es ist jedenfalls deutlich, dass Web Mining eine Entscheidung zwischen der Verbesserung des direkten Verkaufs und der Vertiefung der Kundenbeziehung zugunsten des (zukünftigen) Verkaufs impliziert. Letzteres verlangt eine persönliche Beziehung, für die ein umfassendes Kundenbild erforderlich ist, das sich häufig aus verschiedenen Datenbanken (mit historischen Daten) zusammensetzen lässt. Darüber hinaus werden dabei andere Techniken für die Analyse der Daten benötigt.
Die Notwendigkeit eines persönlichen Angebots
Je persönlicher das Angebot an den Kunden gestaltet wird, desto effektiver ist die Beeinflussung. Insbesondere im Internet gibt es nicht viele Gelegenheiten, den Kunden zu steuern. Denn es gibt viel Ablenkung und mehr als genug Alternativen. Ein Besucher nimmt in kurzer Zeit oberflächlich wahr, ob die Seite interessant genug ist, sie näher zu betrachten und weiter zu lesen, und gibt sich nicht allzu viel Mühe, die Information vollständig aufzunehmen. Dies verlangt die ununterbrochene Anstrengung, die Aufmerksamkeit des Besuchers festzuhalten. Mit einer persönlicheren Gestaltung des Angebots gelingt es, die Aufmerksamkeit des Besuchers länger festzuhalten und ihn in eine bestimmte Richtung zu steuern.
Für das Web Mining bedeutet dies eine weitgehende Differenzierung in der Abgabe einer Vorhersage. Der Vorschlag im Hinblick auf die Wahl des anzubietenden Produkts muss auf individueller Ebene erfolgen. Daneben muss klar sein, in welchen Worten der Vorschlag am besten „verpackt“ werden kann, um dabei so weit wie möglich an die Erlebniswelt des Besuchers anzuschließen. Der Inhalt der Nachricht basiert auf dem Modell, in dem auf das Verhalten und/oder die Interessen des Besuchers verwiesen wird. Und darüber hinaus wird es nach Möglichkeit in einem Layout präsentiert, das den Besucher anspricht, wobei im Übrigen nicht der Eindruck entstehen darf, der Autor bzw. Absender sei unzuverlässig und/oder unglaubwürdig.
Eine persönliche Vorgehensweise bedeutet auch, das der Kunde den Distributionskanal für die Transaktion wählen kann. Möglicherweise kann der Kunde über das Internet noch nicht ausreichend Antwort auf seine Fragen erhalten oder werden ihm dort noch zu wenig Möglichkeiten zum Verdeutlichen seiner eigenen Wünsche geboten. In einem solchen Fall muss er beispielsweise auf ein Callcenter „ausweichen“ können. Zahlreiche große Internetunternehmen betreiben neben ihren Websites ein Callcenter mit entsprechender Kapazität, um ihren Kunden diese Möglichkeit zu bieten.
Dabei ist vor allem darauf zu achten, dass die Nachricht, die an den Kunden geht, nicht unpräzise wird und ihre Schlüssigkeit verliert, denn dies ist die größte Gefahr dieser „Mehrkanal-Strategie“. Innerhalb kurzer Zeit werden verschiedene Angebote abgegeben (beispielsweise über eine Internetseite und später über das Callcenter), die einander nicht widersprechen dürfen, sondern, im Gegenteil, auf den Angaben, die ein Kunde zuvor gemacht hat, aufbauen müssen. Für das Web Mining bedeutet dies, dass die Vorhersage auf die Daten der Kundenvergangenheit, d. h. die Daten eines oder mehrerer vor kurzem stattgefundener Kundenkontakte aufbaut. Daneben sollte sie flexibel angelegt sein, um direkt auf die wichtigsten Einwände des Kunden reagieren und so die Erfolgsaussichten tatsächlich steigern zu können.
Schlussfolgerung
Resümierend lässt sich festhalten, dass sich Web Mining zum Aufspüren und Nutzen eingehender Kundenkontakte über das Internet eignet. Mit Hilfe von Analysen werden Modelle erstellt, auf deren Grundlage dem Kunden ein spezifisches Angebot vorgelegt werden kann. Es erweckt den Anschein großer Komplexität, was nur von Vorteil ist, da es so Interesse hervorruft und Respekt abnötigt. Der Erfolg von Web Mining wird jedoch zu einem großen Teil vom Einsatz der Modelle bestimmt. Implizit muss an dieser Stelle zwischen einer Steigerung der direkten Verkäufe oder der Verbesserung der Kundenbeziehung im Hinblick auf (zukünftige) Verkäufe entschieden werden. Im letzten Fall steht der Kunde im Mittelpunkt und ist eine Echtzeitauswertung in mehreren Distributionskanälen erforderlich. Dabei wird der Kunde auf intelligente Weise persönlich angesprochen, wobei seine persönlichen Präferenzen sowie seine individuelle Vergangenheit berücksichtigt werden. Hier liegen die Ursachen der großen Chancen des Web Mining und seiner Rentabilität.
Privacy en ethiek
Niets te verbergen in Analytische CRM
Gert Haanstra en Nathalie van Capelle, Connect, Kluwer, Deventer, Nr. 6, 2001
Printversie: Niets te verbergen in Analytische CRM
Het heeft iets magisch. De mogelijkheid om verborgen kooppatronen uit diverse databases te halen en met één druk op de knop iemands koopgedrag te kunnen voorspellen. Tegelijkertijd roept het angstige gevoelens op: houden bedrijven mij constant in de gaten? Komen mijn gegevens zomaar op straat te liggen? Worden ze zomaar doorverkocht?
Klanten laten op allerlei manieren sporen na waarmee ze zich ‘bekend’ maken bij bedrijven. Via ‘clicks’ en ‘cookies’ tijdens het surfen over het web, via videobeelden tijdens het winkelen of door mee te doen aan diverse (telefonische) onderzoeken. Het spreekt voor zich dat bedrijven hier zorgvuldig en terughoudend mee om moeten gaan. Nieuwe Europese wetgeving verplicht bedrijven inzichtelijk te maken hoe zij aan klantgegevens zijn gekomen en welke bewerkingen er mee zijn gedaan. Willen ze bepaalde gegevens vasthouden, dan zullen ze daarvoor vooraf toestemming moeten vragen. Daar komt bij dat die gegevens alleen gebruikt mogen worden voor het specifieke doel waarvoor ze zijn aangelegd.
Zijn we hiermee gerustgesteld? Kunnen we ervan uit gaan dat bedrijven zich aan de wettelijke kaders houden en dat we ons derhalve geen zorgen hoeven te maken over onze privacy? En dat bedrijven ethisch handelen wanneer ze met behulp van analytische Customer Relationship Management software databases onderzoeken en klantgedrag trachten te voorspellen?
Nu is het sowieso erg moeilijk om vast te stellen of een persoon of een bedrijf ‘ethisch’ handelt of niet. Een ding is echter zeker: uiteindelijk komt het aan op daden, niet op woorden. Organisaties kunnen wel zeggen dat ze zich ethisch en verantwoord gedragen en fatsoenlijk met de gegevens van klanten omgaan, maar uiteindelijk worden ze – terecht – beoordeeld op wat ze doen.
Openheid in doen en laten wordt dan cruciaal. Alleen door volledig transparant te opereren zijn bedrijven in staat het vertrouwen van klanten te winnen en – minstens even belangrijk – te behouden. Goede Analytische Customer Relationship Management software ondersteunt deze openheid door enerzijds gebruikers succesvol klantinformatie te laten benutten en anderzijds rekening te houden met de privacy(gevoelens) van klanten.
Dit betekent kortom:
• Dat je bij het verzamelen van data klanten goed moet kunnen uitleggen waarom bepaalde data worden vastgehouden en waarom het belangrijk is dat men juiste en volledige gegevens opgeeft. Lukt dit niet, ga er dan maar vanuit dat je als bedrijf niet het juiste doel voor ogen hebt en dat klanten zich verder niet druk zullen maken over wat je gaat doen met hun foutieve gegevens;
• Dat je bij het analyseren van (klant)data duidelijk kunt aangeven welke bewerkingen worden uitgevoerd. Hierbij geldt dat klanten niet eens het naadje van de kous willen weten, zolang ze maar het gevoel hebben dat je hen begrijpt;
• Dat je bij het toepassen van klantkennis in de klantinteracties laat zien dat het effectief gebruiken van (persoonlijke) klantinformatie de klant voordelen oplevert. Klanten willen het effect van een gerichte, persoonlijke aanbieding zeker ervaren, maar merken een verlies aan privacy zodra de aanbieding de plank volledig misslaat.
Consument krijgt meer macht over eigen gegevens
Data is widely available; what is scarce is the ability to extract wisdom from them
- Hal Varian